A novel hybrid data-driven method based on uncertainty quantification to predict the remaining useful life of lithium battery
Journal of Energy Storage(2022)
摘要
Accurate future capacity and remaining useful life (RUL) prediction of lithium battery, as well as uncertainty quantification, are critical for lithium battery management system. A hybrid data-driven method is utilized in this research. Firstly, using complementary ensemble empirical mode decomposition and Hurst exponent-based data refactoring method, raw capacity data is split into two local fluctuation components with various time scales and one component with long-term memory feature. Then, Gaussian process regression (GPR) is utilized to predict the two local fluctuation components, and long short-term memory (LSTM) neural network is utilized to predict the long-term memory feature component, while the prediction error and its uncertainty quantification are given by using GPR learning training error. Finally, all results are combined into the future capacity prediction value and its 95% confidence interval. Applying multiple datasets of lithium battery capacity degradation, the proposed method is compared to others. The experimental results reveal that the proposed method outperforms the other methods in the future capacity prediction, with root mean squared error (RMSE) and mean absolute error (MAE) of 0.01057 and 0.00659. Furthermore, when RUL prediction is conducted early in the cycle, the proposed method has strong generalization ability and trustworthy uncertainty management capacity.
更多查看译文
关键词
Lithium battery,Hybrid data-driven technology,Remaining useful life prediction,Uncertainty quantification
AI 理解论文
溯源树
样例
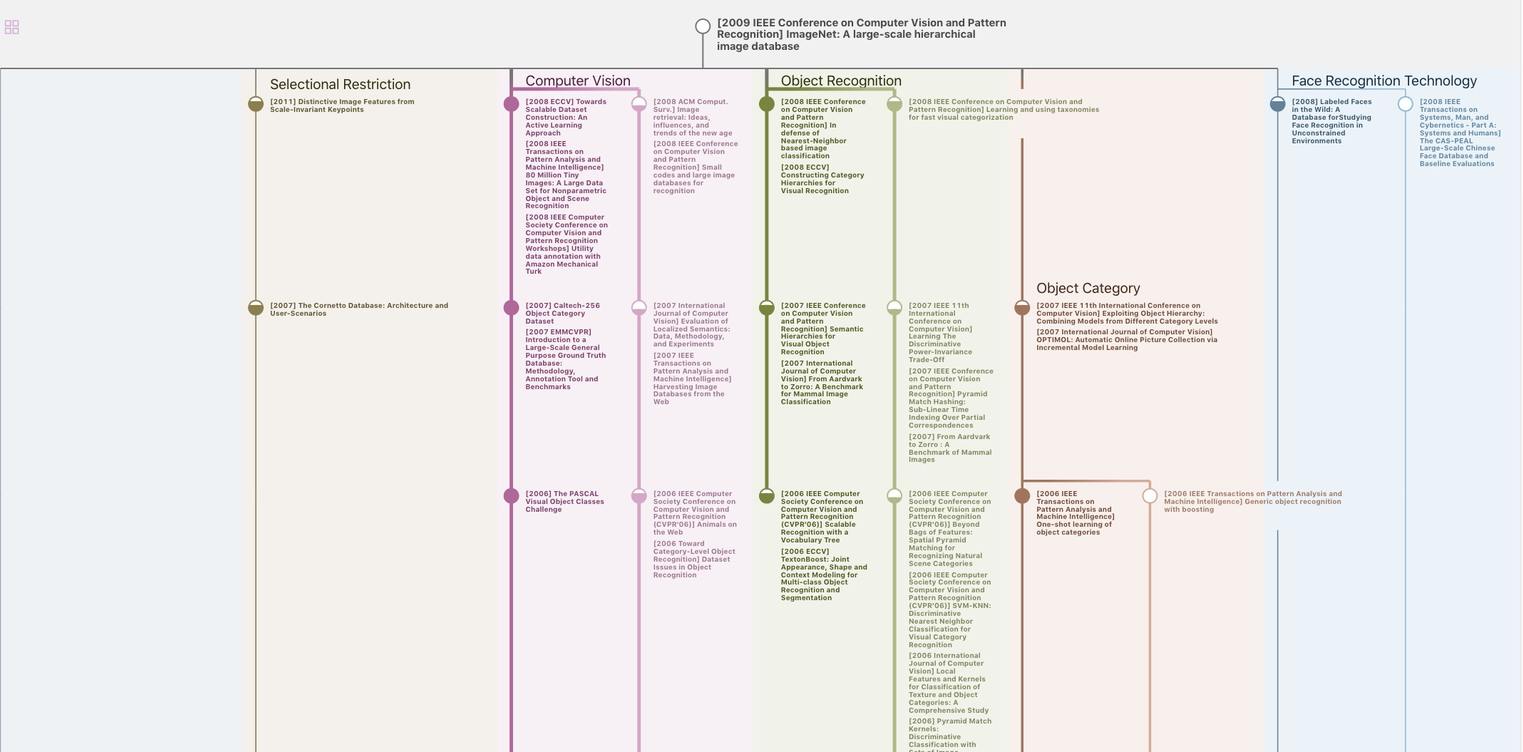
生成溯源树,研究论文发展脉络
Chat Paper
正在生成论文摘要