MCWS-Transformers: Towards an Efficient Modeling of Protein Sequences via Multi Context-Window Based Scaled Self-Attention
IEEE/ACM Transactions on Computational Biology and Bioinformatics(2022)
摘要
This paper advances the self-attention mechanism in the standard transformer network specific to the modeling of the protein sequences. We introduce a novel context-window based scaled self-attention mechanism for processing protein sequences that is based on the notion of (i) local context and (ii) large contextual pattern. Both notions are essential to building a good representation for protein sequences. The proposed context-window based scaled self-attention mechanism is further used to build the multi context-window based scaled (MCWS) transformer network for the protein function prediction task at the protein sub-sequence level. Overall, the proposed MCWS transformer network produced improved predictive performances, outperforming existing state-of-the-art approaches by substantial margins. With respect to the standard transformer network, the proposed network produced improvements in F1-score of +2.30% and +2.08% on the biological process (BP) and molecular function (MF) datasets, respectively. The corresponding improvements over the state-of-the-art ProtVecGen-Plus+ProtVecGen-Ensemble approach are +3.38% (BP) and +2.86% (MF). Equally important, robust performances were obtained across protein sequences of different lengths.
更多查看译文
关键词
Amino Acid Sequence,Software Design,Proteins
AI 理解论文
溯源树
样例
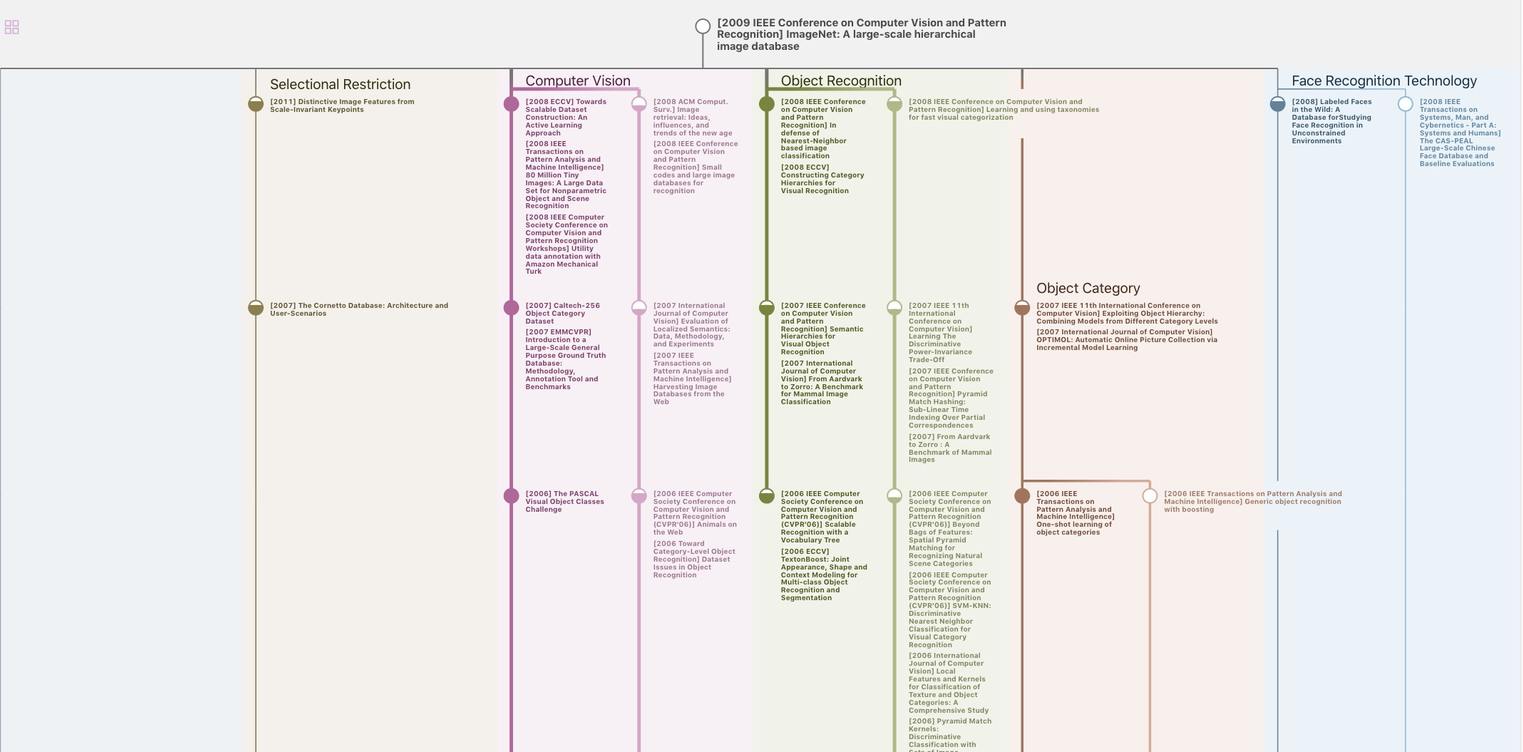
生成溯源树,研究论文发展脉络
Chat Paper
正在生成论文摘要