SSIS-Seg: Simulation-Supervised Image Synthesis for Surgical Instrument Segmentation
IEEE Transactions on Medical Imaging(2022)
摘要
Surgical instrument segmentation can be used in a range of computer assisted interventions and automation in surgical robotics. While deep learning architectures have rapidly advanced the robustness and performance of segmentation models, most are still reliant on supervision and large quantities of labelled data. In this paper, we present a novel method for surgical image generation that can fuse robotic instrument simulation and recent domain adaptation techniques to synthesize artificial surgical images to train surgical instrument segmentation models. We integrate attention modules into well established image generation pipelines and propose a novel cost function to support supervision from simulation frames in model training. We provide an extensive evaluation of our method in terms of segmentation performance along with a validation study on image quality using evaluation metrics. Additionally, we release a novel segmentation dataset from real surgeries that will be shared for research purposes. Both binary and semantic segmentation have been considered, and we show the capability of our synthetic images to train segmentation models compared with the latest methods from the literature.
更多查看译文
关键词
Image Processing, Computer-Assisted,Computer Simulation,Algorithms,Surgical Instruments,Semantics
AI 理解论文
溯源树
样例
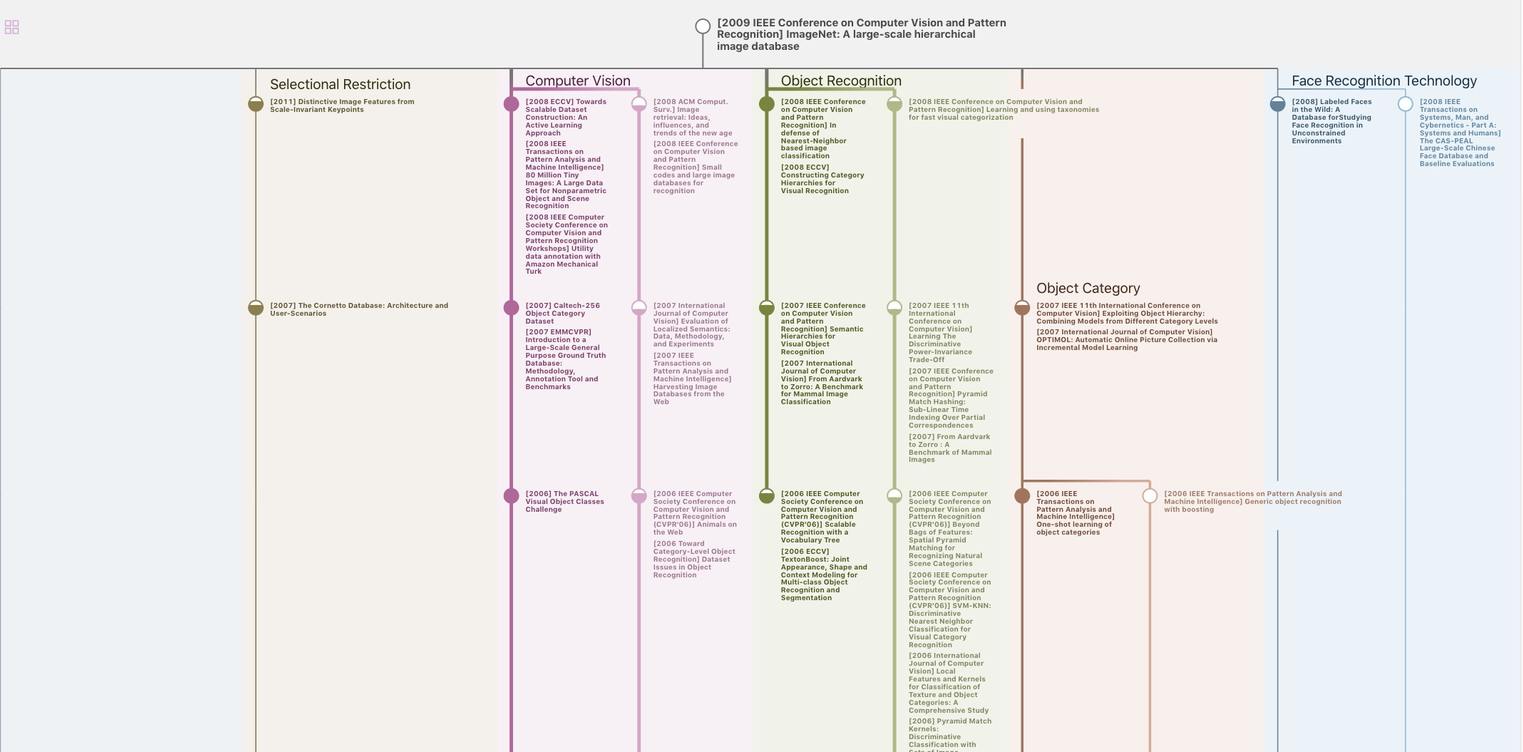
生成溯源树,研究论文发展脉络
Chat Paper
正在生成论文摘要