Federated Learning for Electronic Health Records
ACM Transactions on Intelligent Systems and Technology(2022)
摘要
In data-driven medical research, multi-center studies have long been preferred over single-center ones due to a single institute sometimes not having enough data to obtain sufficient statistical power for certain hypothesis testings as well as predictive and subgroup studies. The wide adoption of electronic health records (EHRs) has made multi-institutional collaboration much more feasible. However, concerns over infrastructures, regulations, privacy, and data standardization present a challenge to data sharing across healthcare institutions. Federated Learning (FL), which allows multiple sites to collaboratively train a global model without directly sharing data, has become a promising paradigm to break the data isolation. In this study, we surveyed existing works on FL applications in EHRs and evaluated the performance of current state-of-the-art FL algorithms on two EHR machine learning tasks of significant clinical importance on a real world multi-center EHR dataset.
更多查看译文
关键词
Federated learning,electronic health records,healthcare,neural networks
AI 理解论文
溯源树
样例
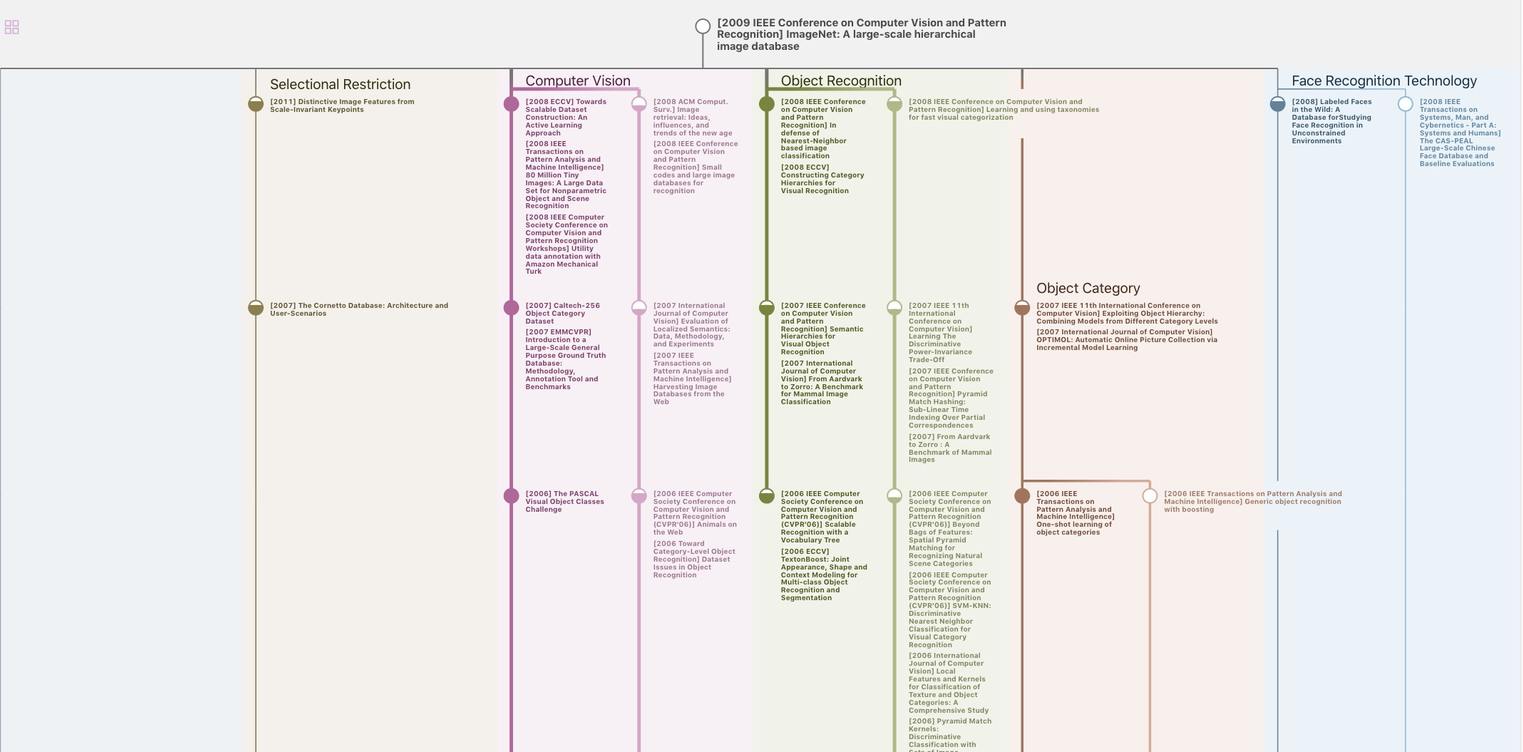
生成溯源树,研究论文发展脉络
Chat Paper
正在生成论文摘要