Feasibility of Conducting Long-term Health and Behavior Follow Up in Adolescents (Preprint)
JMIR Formative Research(2022)
Abstract
UNSTRUCTURED Machine Learning (ML) is a field of artificial intelligence studying algorithms that improve automatically through experience. This statistical learning approach is a natural extension of traditional statistical methods and can offer potential advantages for certain problems. The feasibility of using ML techniques in healthcare is predicated on access to patients’ data and building reliable data sets. This study assesses the feasibility of data collection from an adolescent population before and after a major surgical operation. Both physical and psychosocial data were collected. Adolescents scheduled for a Posterior Spine Fusion operation were approached at the time when they were scheduled for the surgery. The study collected repeated measures of patient data, including at least two weeks prior to the operation, and six months after patients were discharged from the hospital. Patients were provided with a Fitbit Charge 4 (consumer-grade health tracker) and instructed to wear it as often as possible. Patients were trained to download personal data on step counts, sleep time, and heart rate into a web-based portal, which could then be accessed by the study team. We retrieved demographic and physiologic data, stored in the Hospital Data Warehouse, from the patients’ EMR. We evaluated changes in the patients’ psychological profile over time using several validated questionnaires (i.e., Pain Catastrophizing Scale, Patient Health Questionnaire, Generalized Anxiety Disorder Scale, Pediatric Quality of Life Inventory). Questionnaires were administered to patients using Qualtrics software. Patients received the questionnaire prior to and during the hospitalization and then three and six months post-surgery. We administered paper-based questionnaires for self-report of daily pain scores and usage of analgesic medications. We faced several challenges. Only 38% of the patients we approached were eligible to be part of the study and among enrolled patients, 38% dropped out during the follow-up period, on average 2.2 months into the study. Of those who completed the study, 60% reliably wore the Fitbit and downloaded data into the Web-based portal. These patients also had a high response rate to the psychosocial surveys. However, none of the patients who finished the study completed the paper-based pain diary. We did not find any difficulty accessing demographic and clinical data stored in the hospital data warehouse. In conclusion, although we engaged teenagers in long-term medical follow-up, we identified several challenges related to the lack of incentives and personal contact between the researchers and patients that should be addressed in future studies.
MoreTranslated text
Key words
Self-Monitoring,Behavior Change
AI Read Science
Must-Reading Tree
Example
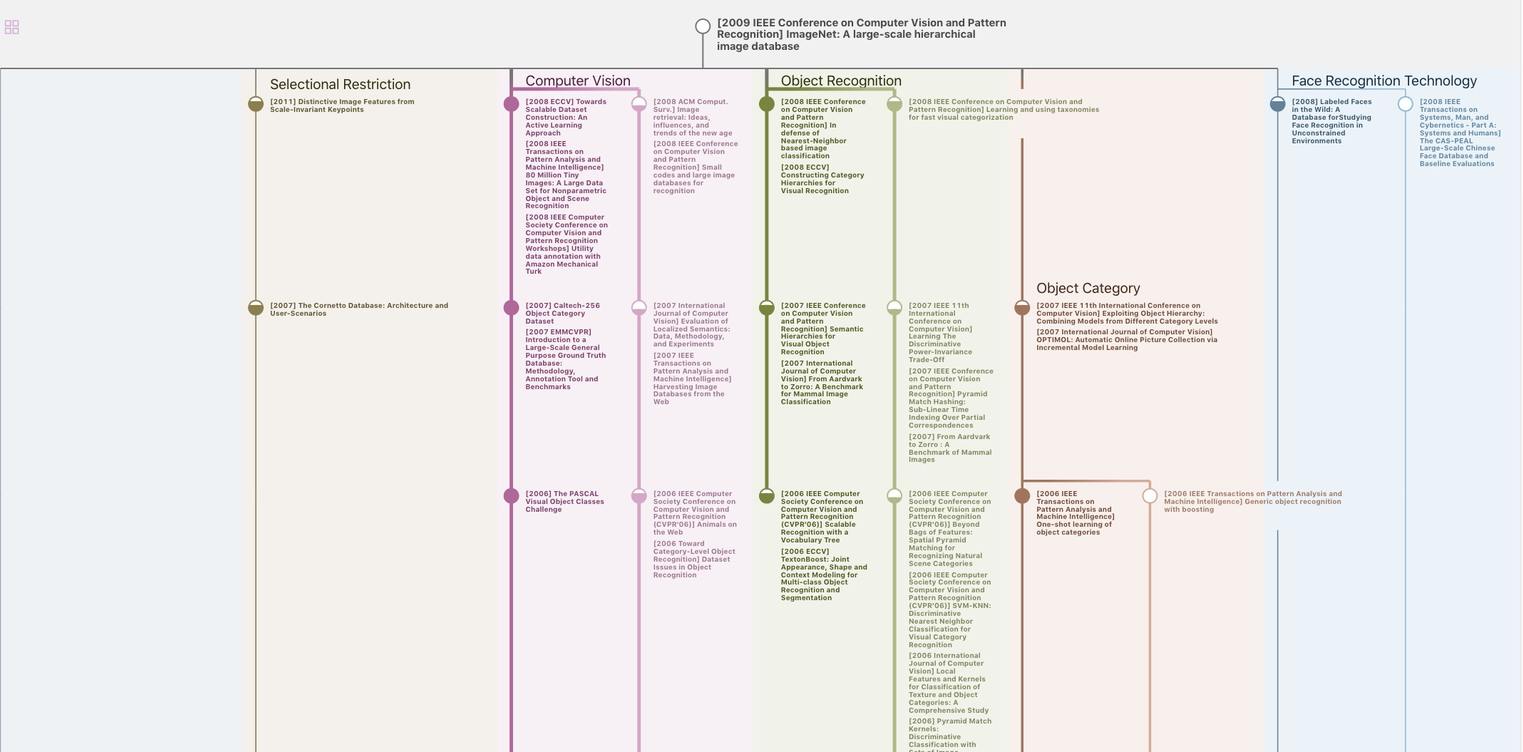
Generate MRT to find the research sequence of this paper
Chat Paper
Summary is being generated by the instructions you defined