ESTIMATION OF THE RISK OF PROGRESSION AND RENAL SUBSTITUTION TREATMENT IN PATIENTS WITH CHRONIC KIDNEY DISEASE USING AN ARTIFICIAL INTELLIGENCE MODEL
Nephrology Dialysis Transplantation(2022)
Abstract
Abstract BACKGROUND AND AIMS The use of tools that allow to estimate the probability of progression of chronic kidney disease (CKD) to advanced stages, has not yet gained a notable space in our clinical practice. The aim of this study was to develop and validate a model to predict disease progression, and the need for renal replacement therapy, based on machine learning, for patients with CKD grades 3 to 5. This is the first model of its kind to be implemented in Colombia. METHOD This is an observational retrospective closed cohort study. We included patients with CKD from a primary care program and followed them for 5 years. Demographic, clinical and laboratory predictors were included. The outcomes were CKD stage progression, >25% reduction in baseline glomerular filtration rate (eGFR) and the need for renal replacement therapy (RRT), dialysis, or kidney transplantation. We designed two prediction models, one time-independent, based on logistic regression, neural networks and random forest; and a second model, a survival analysis (time to event), by Cox regression, random forest and gradient boosting. Finally, we conducted an internal validation with bootstrapping for the first model, and external validation for the second model in a second real cohort. As a result, we designed a risk calculator. RESULTS Time-independent model included 2619 patients, 62.7% female, 92.6% with hypertension, 23.5% diabetics, with a baseline eGFR 51.06 mL/min/1.73m2 (40–61.9). An estimated performance for predicting RRT risk of F1: 0.61 and Kappa: 0.60 (113 events) was detected; stage progression of F1: 0.4 and Kappa: 0.25; reduction in eGFR F1: 0.29 and Kappa: 0.21. For this first model, the most relevant predictors were: initial eGFR, hemoglobin, sex, central region, total cholesterol and socioeconomic status. The second, a 5-year Time-to-Event prediction model, included data from 1803 patients, had good accuracy with a C-Index assessed performance for RRT risk of 0.96, grade progression of 0.71 and eGFR reduction of 0.69 respectively. The most relevant predictors were initial eGFR, mean heart rate, hemoglobin, hospitalizations, sex, hypertension, diabetes, total cholesterol and central region. The validation cohort, with 658 patients with CKD, 63.9% male, 39% diabetics, with a mean eGFR of 34.9 mL/min/1.73m2, showed good accuracy with a C-Index for RRT risk of 0.935, grade progression of 0.69 and eGFR reduction of 0.0.62 respectively. CONCLUSION Our study demonstrated the utility of novel tools to predict renal outcomes in a CKD population in Colombia. This is the first study conducted with our local population. The resulted risk calculator is intended to be part of the electronic medical history in our hospitals.
MoreTranslated text
Key words
chronic kidney disease,renal substitution treatment,artificial intelligence
AI Read Science
Must-Reading Tree
Example
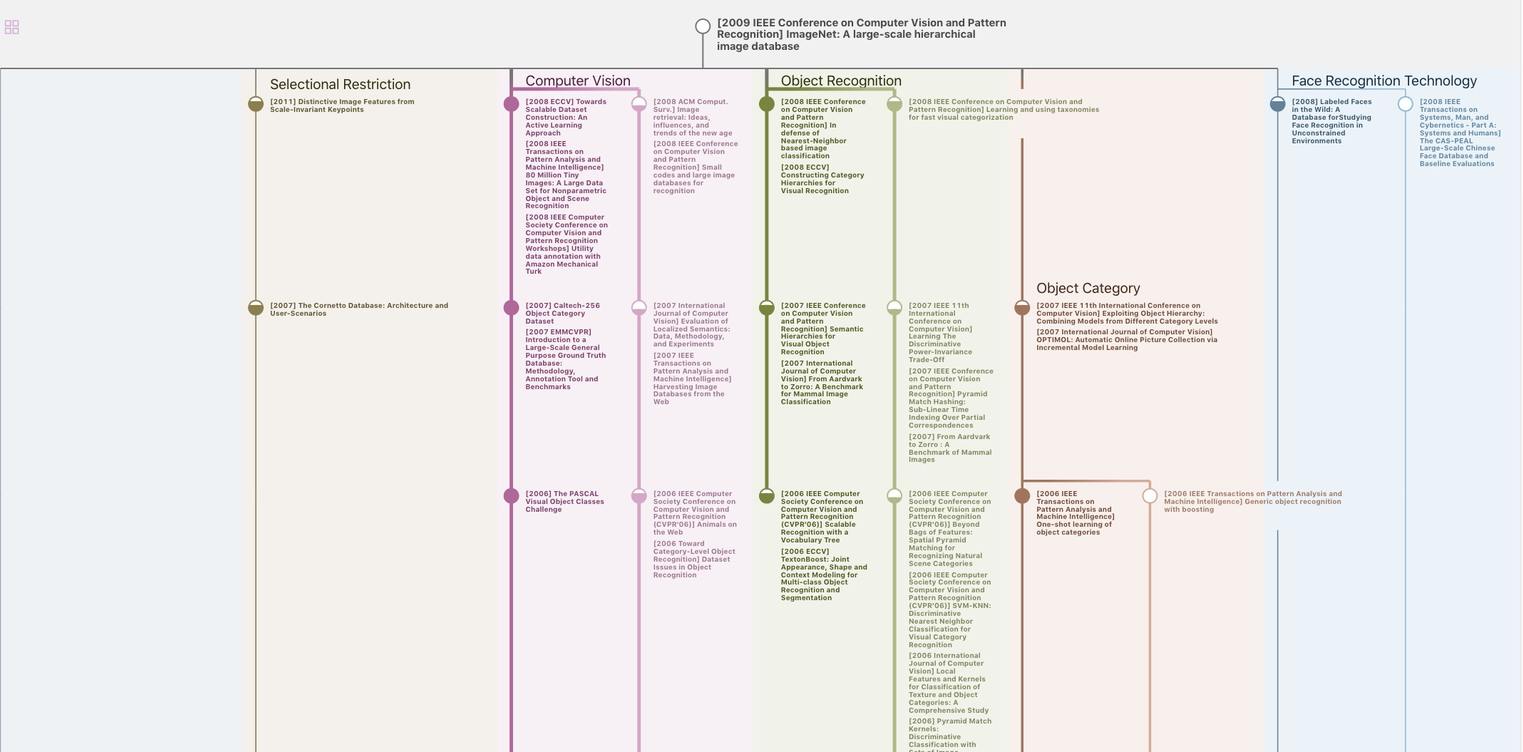
Generate MRT to find the research sequence of this paper
Chat Paper
Summary is being generated by the instructions you defined