Learning-Based User Clustering in NOMA-Aided MIMO Networks With Spatially Correlated Channels
IEEE Transactions on Communications(2022)
摘要
This paper considers the integration of non-orthogonal multiple access (NOMA) into massive multi-input multi-output (MIMO) systems for downlink transmission. We consider the joint design of user clustering, transmit beamforming, and power allocation to minimize the total transmit power while meeting the signal-to-interference-and-noise ratio targets. We decompose this challenging mixed-integer programming problem into three separate subproblems to solve. We propose a low-complexity learning-based user clustering algorithm, which is a modified version of mean shift clustering with a new channel correlation based clustering metric. The proposed clustering algorithm determines the clusters to trade-off between spatial dimension and power dimension offered by respective MIMO and NOMA for user multiplexing. We then design zero-forcing transmit beamformers to eliminate inter-cluster interference and optimize power allocation to minimize the total transmit power. We provide two case studies for both co-located and distributed massive MIMO systems in spatially highly correlated prorogation environments. Simulation results show that our proposed algorithm forms NOMA clusters based on the available degrees of freedom in the system to effectively use both spatial and power dimensions, which results in a substantial performance improvement over MIMO-only methods or other existing clustering methods in such environments.
更多查看译文
关键词
Massive MIMO,NOMA,user clustering,mean shift clustering,power allocation,correlated channel
AI 理解论文
溯源树
样例
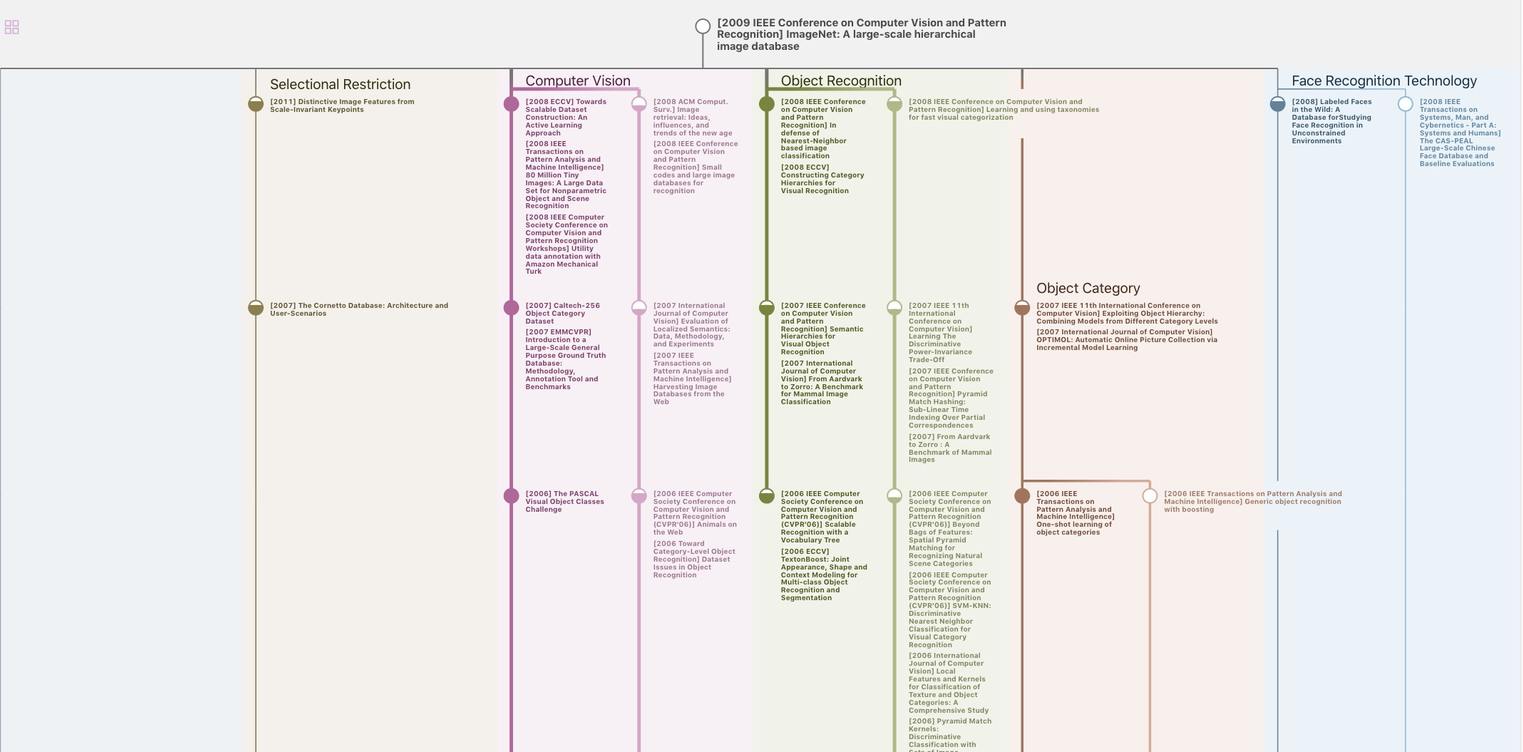
生成溯源树,研究论文发展脉络
Chat Paper
正在生成论文摘要