Brain Shape Correspondence Analysis Using Variational Mixtures for Gaussian Process Latent Variable Models
Artificial Intelligence in Neuroscience: Affective Analysis and Health Applications(2022)
摘要
Analyzing brain structures in the medical imaging field poses challenging problems due to neurological diseases’ heterogeneity. Besides, measuring brain changes quantitatively in neurodevelopmental is crucial to evaluate clinical outcomes correctly. From a computer-vision perspective, establishing correspondences between shapes often requires computing similarity measures that, in most cases, are unavailable. This paper proposes an unsupervised probabilistic framework for shape correspondence analysis on brain structures by using variational unsupervised learning. The probabilistic framework comprehensively captures the form of brain shapes from surface descriptors. Then, we learned clustered latent space representations of surface descriptors by using mixtures distributions for Gaussian process latent variable models to avoid computing similarity measures, which classify the resulting latent vectors to establish group-wise correspondences. The experimental results show how the proposed model captures non-linearities in non-rigid 3D shapes even when they present occlusion or partialities. These results demonstrated that the proposed model is suitable for shape correspondence analysis.
更多查看译文
关键词
Brain modelling, Shape correspondence, Variational inference, Gaussian process latent variable models
AI 理解论文
溯源树
样例
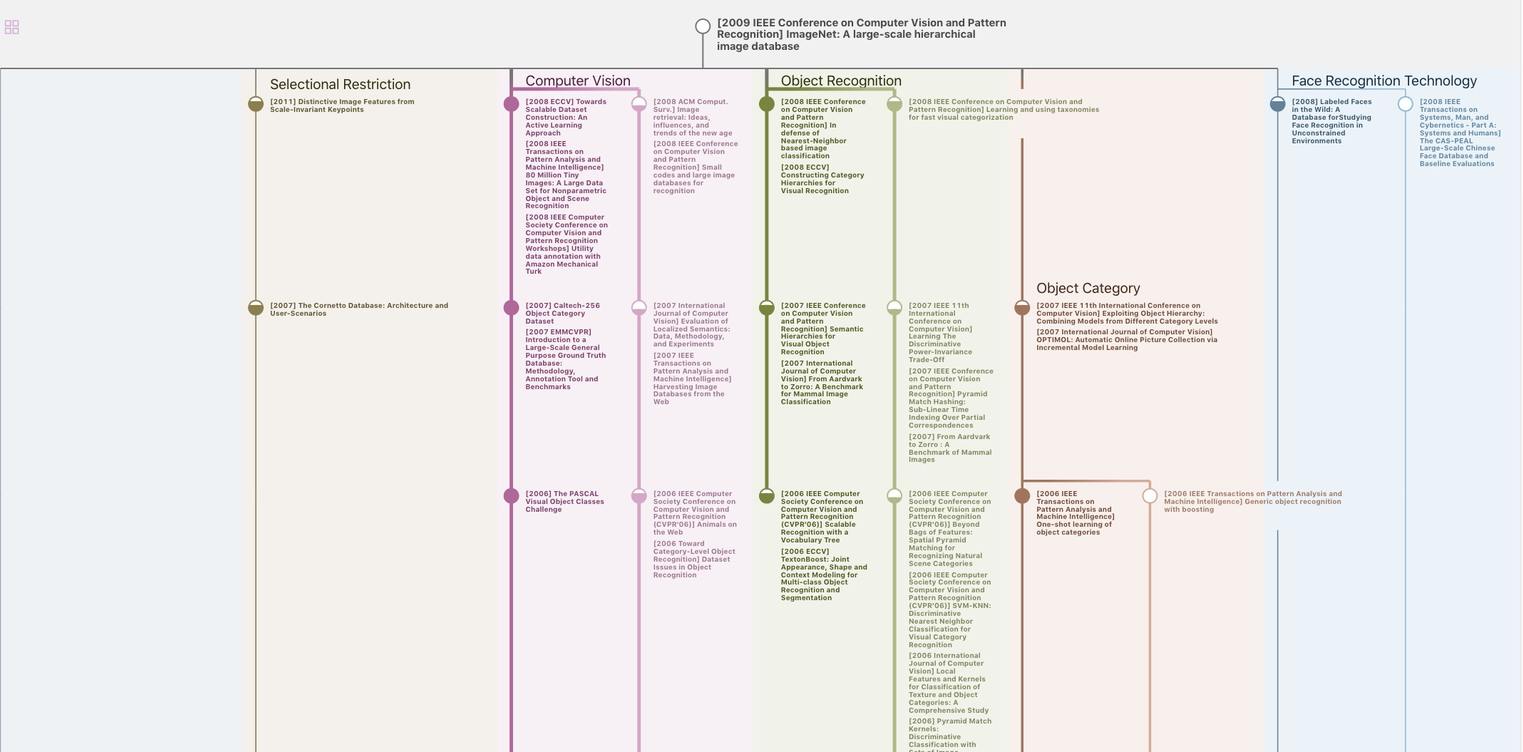
生成溯源树,研究论文发展脉络
Chat Paper
正在生成论文摘要