Feature self-calibration network with global-local training strategy for multi-region deformable medical image registration
Neural Computing and Applications(2022)
摘要
3D deformable medical image registration has important clinical significance. Deep learning-based methods have shown outstanding advantages in medical image registration. However, most current learning-based practices only have sound registration effects for large-area overall deformed medical images such as lung and liver CT scans. Medical images with multiple registration regions, such as brain MR scans, have become a significant shortcoming in the field of medical image registration. The current learning-based method uses only one displacement field to deform the entire moving image. For brain images with multiple registration areas, this method cannot guarantee accurate deformation of all regions, nor can it ensure the excellent maintenance of the topological structure of each part. Aiming to resolve this unresolved problem in the field of medical image registration, we propose a novel training strategy suitable for multi-region registration: global and local joint training strategy ( GoLo ). And then, we combine it with our proposed feature self-calibration network ( FSCN ) to solve the registration optimization problem of multi-region medical images. We conducted many quantitative and qualitative evaluation tests on the public brain MR scan data set(OASIS). The test results show that our method can further improve the accuracy of the registered image and ensure reversibility compared with the existing state-of-the-art methods.
更多查看译文
关键词
Multi-region medical image registration,Global and local joint training strategy,Feature self-calibration network
AI 理解论文
溯源树
样例
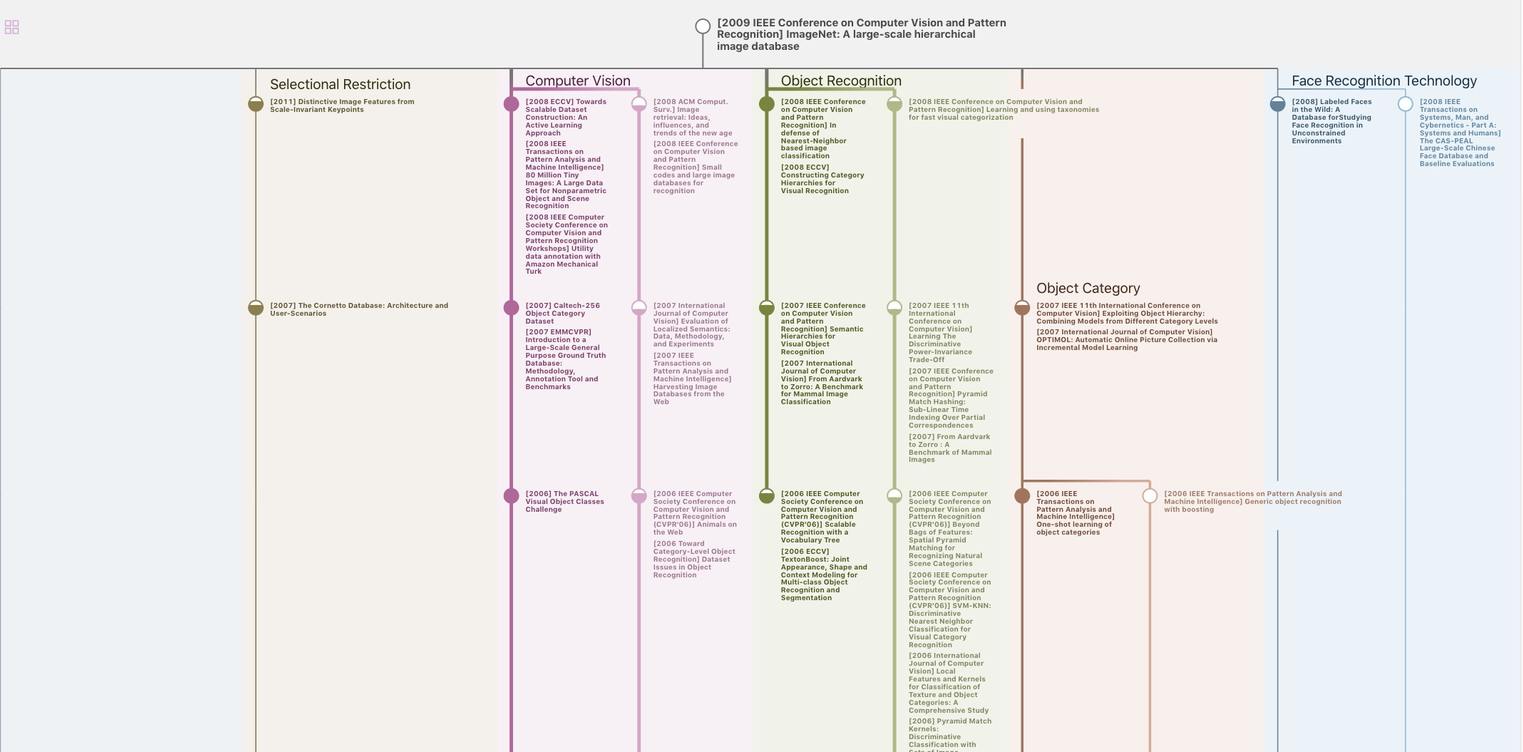
生成溯源树,研究论文发展脉络
Chat Paper
正在生成论文摘要