Model-Predictive Quantum Control via Hamiltonian Learning
IEEE Transactions on Quantum Engineering(2022)
摘要
This article proposes an end-to-end framework for the learning-enabled control of closed quantum systems. The proposed learning technique is the first of its kind to utilize a hierarchical design, which layers probing control, quantum state tomography, quantum process tomography, and Hamiltonian learning to identify both the internal and control Hamiltonians. Within this context, a novel quantum process tomography algorithm is presented, which involves optimization on the unitary group, i.e., the space of unitary operators, to ensure physically meaningful predictions. Our scalable Hamiltonian learning algorithms have low memory requirements and tunable computational complexity. Once the Hamiltonians are learned, we formalize data-driven model-predictive quantum control (MPQC). This technique utilizes the learned model to compute quantum control parameters in a closed-loop simulation. Then, the optimized control input is given to a physical quantum system in an open-loop fashion. Simulations show model-predictive quantum control to be more efficient than the current state-of-the-art, quantum optimal control, when sequential quadratic programming (SQP) is used to solve each control problem.
更多查看译文
关键词
Quantum computing,quantum consensus,quantum control,quantum Hamiltonian learning,quantum networks,quantum process tomography
AI 理解论文
溯源树
样例
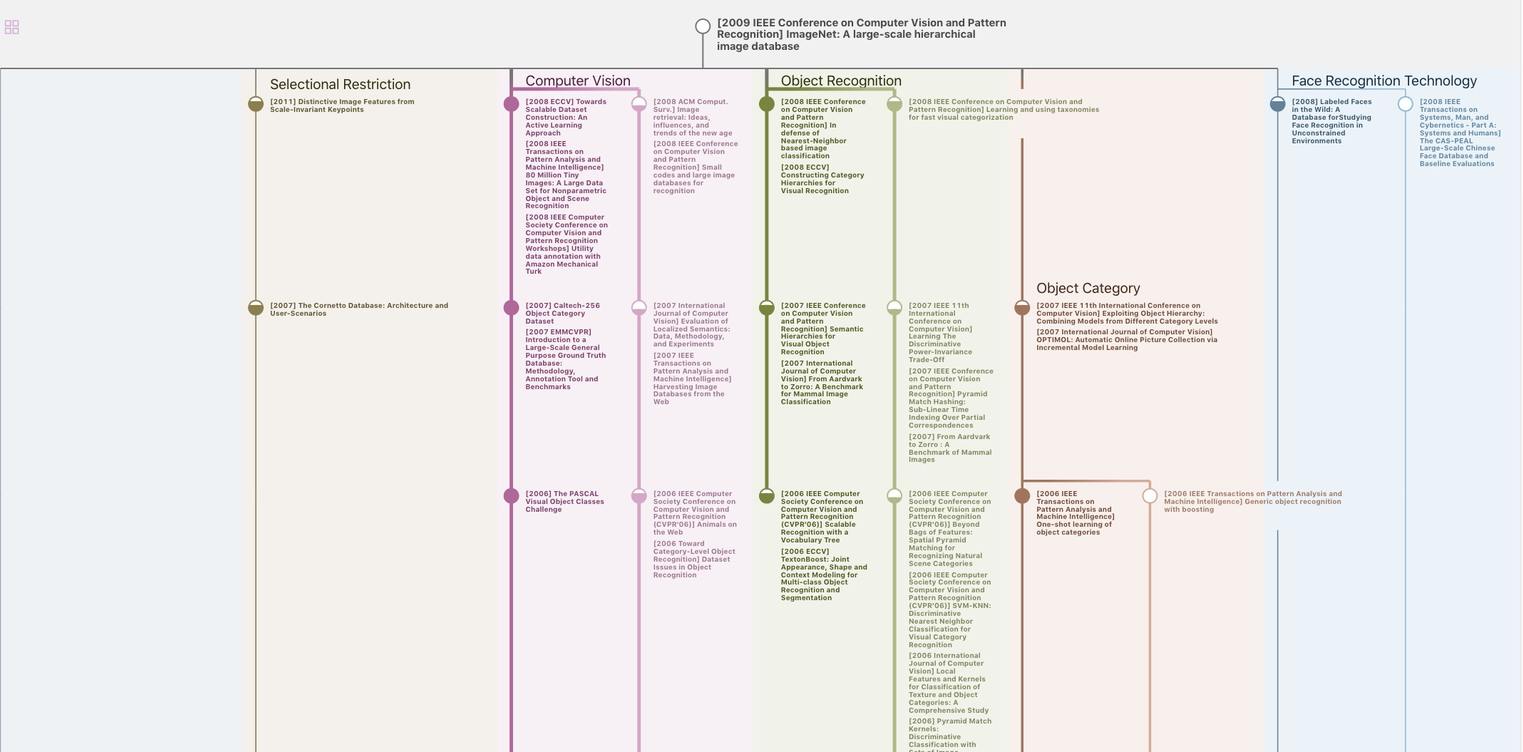
生成溯源树,研究论文发展脉络
Chat Paper
正在生成论文摘要