Am I Positive? Improving HIV Testing in the Era of Pre-Exposure Prophylaxis (PrEP) and Immediate Anti-Retroviral Therapy (iART) using Machine Learning
Open Forum Infectious Diseases(2022)
摘要
Abstract Background HIV testing is the first step in the HIV prevention cascade. The CDC HIV laboratory diagnostic testing algorithm was developed before pre-exposure prophylaxis (PrEP) and immediate antiretroviral therapy (iART) became standards of care. PrEP and iART have been shown to delay antibody development and affect the performance of screening HIV assays. Quantitative results from fourth-generation HIV testing may be helpful to disambiguate HIV testing. Methods We retrospectively reviewed 38,850 results obtained at an urban, academic medical center. We assessed signal-to-cutoff (s/co) distribution among positive and negative tests, patients engaged and not engaged in an HIV prevention program, and evaluated changes in patients with multiple results. Classification and regression tree (CART) analysis was used to determine a threshold cutoff, and logistic regression was used to identify predictors of true positive tests. Results 97% of patients with a negative HIV test had a result that was <=0.2 s/co. For patients tested more than once, we found differences in s/co values did not exceed 0.2 s/co for 99.2% of results. CART identified a s/co value, 38.78, that in logistic regression on a unique validation cohort remained associated with the likelihood of a true positive HIV result (OR 2.49). Conclusions Machine learning methods may be used to improve HIV screening by automating and improving interpretations, incorporating them into robust algorithms, and improving disease prediction. Further investigation is warranted to confirm if s/co values combined with a patient's risk profile will allow for better clinical decision-making for individuals on PrEP or eligible for iART.
更多查看译文
AI 理解论文
溯源树
样例
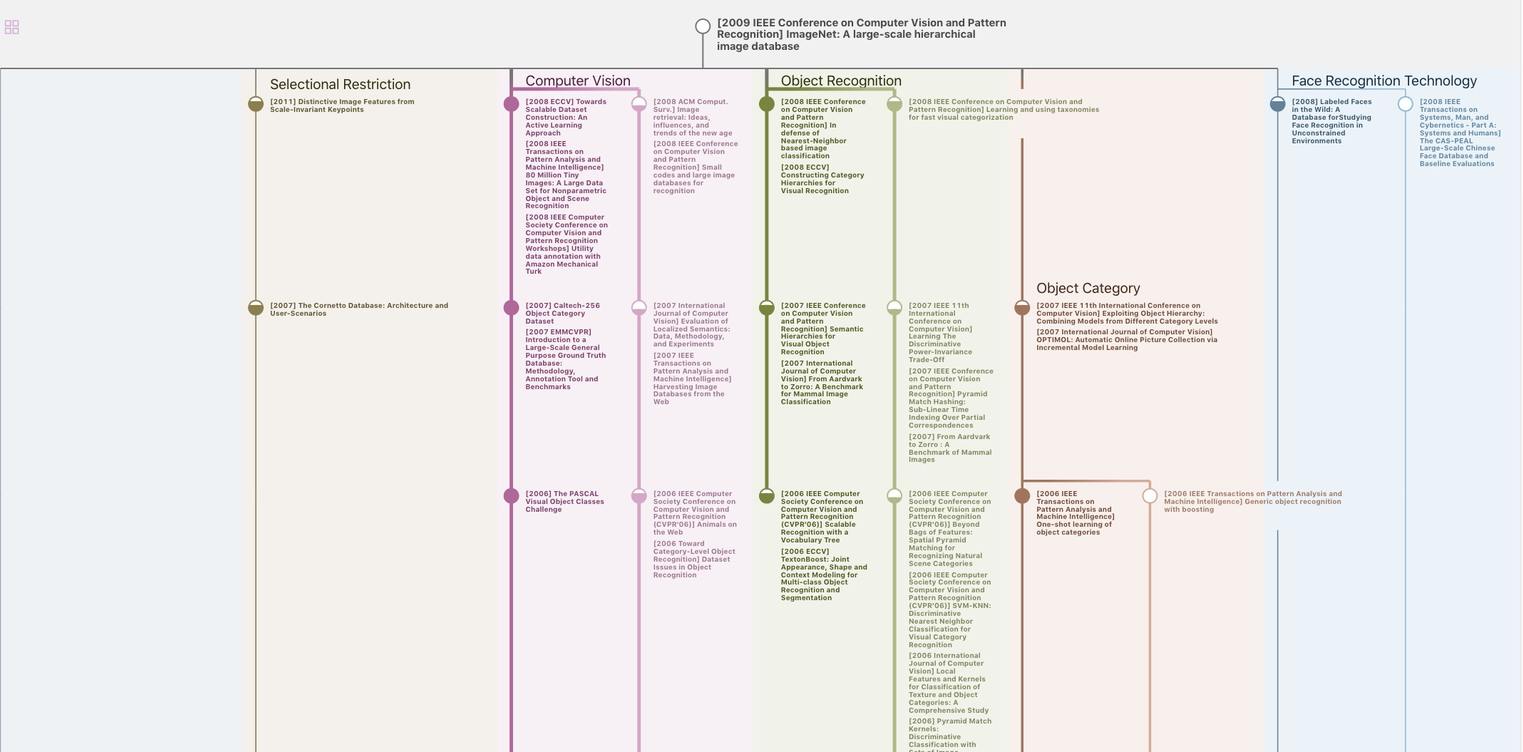
生成溯源树,研究论文发展脉络
Chat Paper
正在生成论文摘要