Cross-Receptive Focused Inference Network for Lightweight Image Super-Resolution
IEEE TRANSACTIONS ON MULTIMEDIA(2024)
摘要
Recently, Transformer-based methods have shown impressive performance in single image super-resolution (SISR) tasks due to the ability of global feature extraction. However, the capabilities of Transformers that need to incorporate contextual information to extract features dynamically are neglected. To address this issue, we propose a lightweight Cross-receptive Focused Inference Network (CFIN) that consists of a cascade of CT Blocks mixed with CNN and Transformer. Specifically, in the CT block, we first propose a CNN-based Cross-Scale Information Aggregation Module (CIAM) to enable the model to better focus on potentially helpful information to improve the efficiency of the Transformer phase. Then, we design a novel Cross-receptive Field Guided Transformer (CFGT) to enable the selection of contextual information required for reconstruction by using a modulated convolutional kernel that understands the current semantic information and exploits the information interaction within different self-attention. Extensive experiments have shown that our proposed CFIN can effectively reconstruct images using contextual information, and it can strike a good balance between computational cost and model performance as an efficient model.
更多查看译文
关键词
Transformers,Computational modeling,Computed tomography,Feature extraction,Convolution,Adaptation models,Task analysis,SISR,Cross-receptive,contextual information,efficient model
AI 理解论文
溯源树
样例
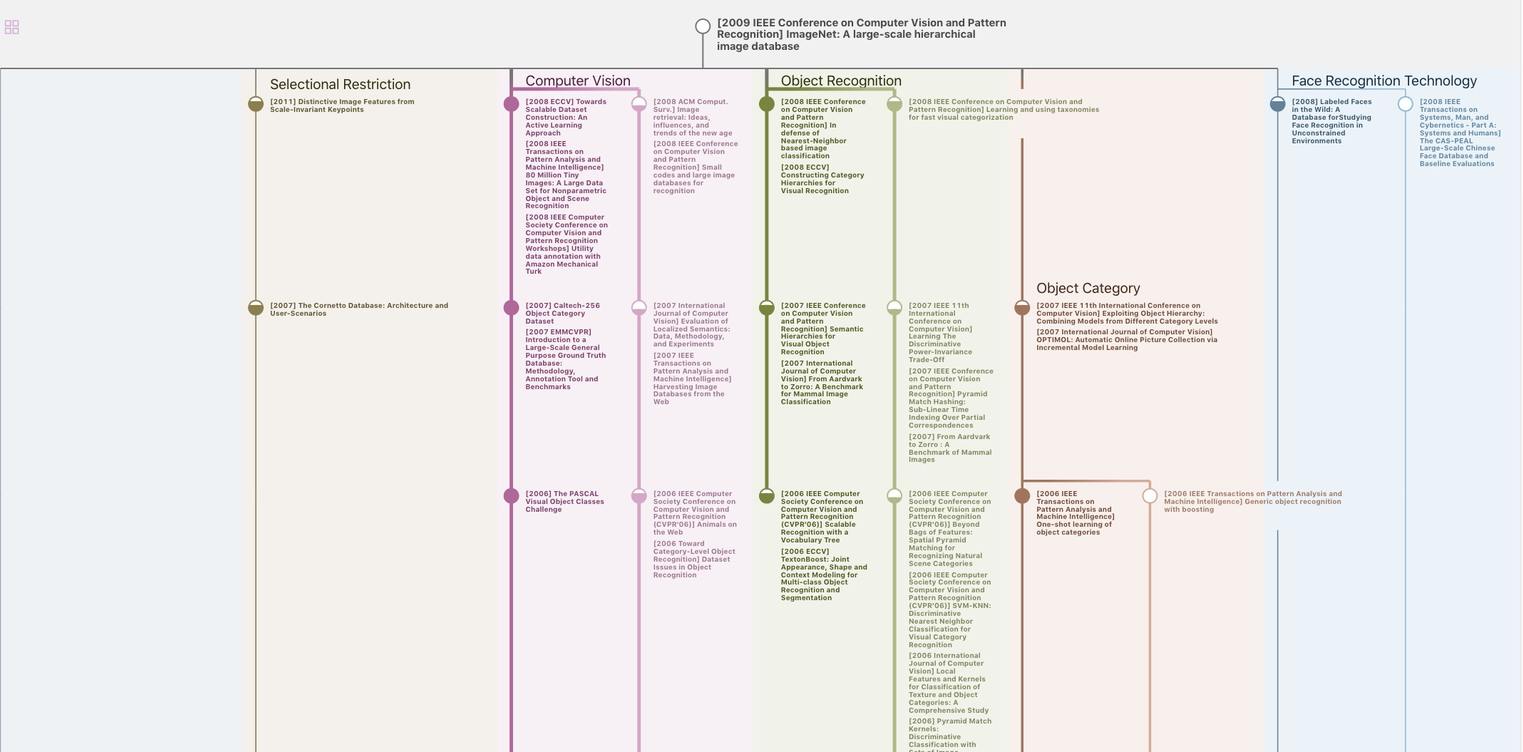
生成溯源树,研究论文发展脉络
Chat Paper
正在生成论文摘要