Predicting major bleeding events in anticoagulated cancer patients with venous thromboembolism using real-world data and machine learning.
Journal of Clinical Oncology(2022)
摘要
e18744 Background: Evidence regarding the clinical predictors of bleeding risk in patients with cancer and venous thromboembolism (VTE) is lacking. Our aim was to develop a predictive model to assess the risk of major bleeding (MB) in anticoagulant-treated patients with active cancer during the first 6 months following VTE diagnosis. Methods: Observational, retrospective, and multicenter study based on the secondary analysis of unstructured clinical data in electronic health records (EHRs). Using the EHRead technology, based on Natural Language Processing (NLP) and machine learning (ML), data were collected from EHRs from 9 Spanish hospitals between 2014 and 2018. The study population comprised all adult cancer patients with a diagnosis of VTE under anticoagulant treatment and no history of MB. This population was downsampled to prevent bias and class imbalance. A total of 94 patient characteristics were explored, and Random Forest (RF) feature selection was performed to identify the most relevant predictors. Multiple algorithms were used to train different prediction models, which were subsequently validated in a hold-out dataset. The model with the best performance metrics (i.e., ROC-AUC) was selected as the final model. Results: Among a source population of 2,893,208 patients, 21,227 anticoagulant-treated patients with VTE and active cancer were identified from EHRs. Of these, 53.9% men, with a median age (Q1, Q3) of 70 (59,80) years. The median duration of follow up across all patients was 0.7 (0.11, 2.03) years. During the study period, estimated in-hospital prevalence of cancer-related VTE was 5.8 %. The most common type of VTE at baseline was deep vein thrombosis (68.2 % of patients), followed by pulmonary embolism (28.4%). The most frequent primary cancers were colorectal (10.1%) and lung cancer (8.5 %). Of all trained and validated models, the RF approach yielded the best performance, with a ROC-AUC = 0.7. The following predictors of MB were identified: hemoglobin levels, presence of metastasis, patient’s age, platelet count, leukocyte count, and serum creatinine levels. Conclusions: This is the first multicenter study to use NLP to extract the unstructured information from EHRs to develop a predictive model for MB in anticoagulated cancer patients with VTE. These results may improve the prevention and management of bleeding in these patients.
更多查看译文
关键词
major bleeding events,venous thromboembolism,machine learning,cancer patients,real-world
AI 理解论文
溯源树
样例
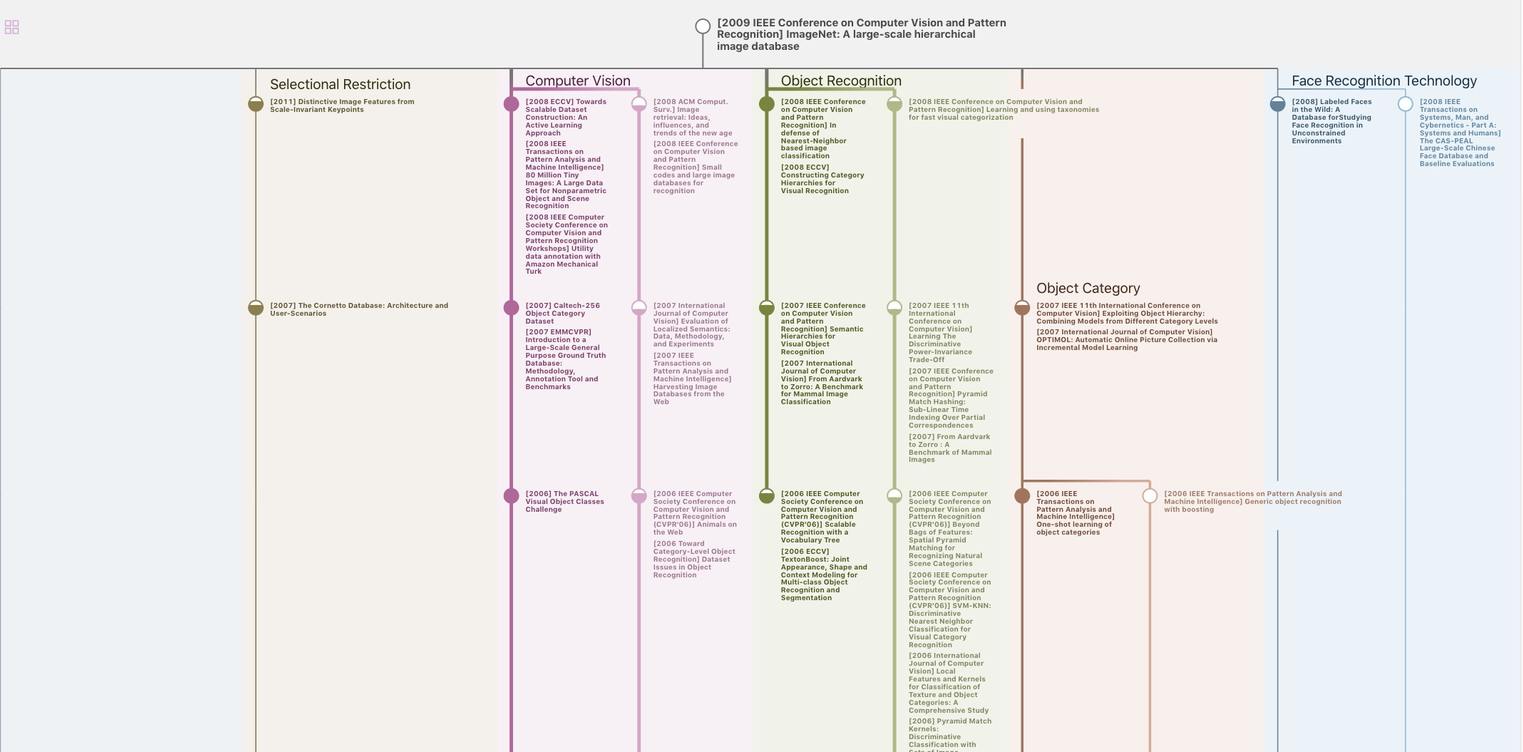
生成溯源树,研究论文发展脉络
Chat Paper
正在生成论文摘要