Defining resistance mechanisms to CDK4/6 inhibition in hormone receptor-positive HER2-negative metastatic breast cancer (MBC) through a machine learning approach applied to circulating tumor DNA (ctDNA).
Journal of Clinical Oncology(2022)
摘要
3055 Background: Although cyclin-dependent kinase 4/6 inhibitors (CDK4/6i) are a primary treatment for hormone receptor-positive/HER2 negative MBC, data regarding resistance mechanisms are still an unmet need. The aim of the study was to highlight new resistance pathways using machine learning (ML) to detect multiparametric patterns in complex datasets. Methods: The study retrospectively analyzed a cohort of 610 hormone receptor positive HER2 negative MBC patients (pts) at Northwestern University, Massachusetts General Hospital and Washington University in St. Louis between 2015-2020 with baseline ctDNA testing by Guardant360. Pathways were defined based on previous work (Sanchez-Vega F et al, Cell. 2018) (i.e., RTK, RAS, RAF, MEK, NRF2, ER, WNT, MYC, P53, cell cycle, notch, PI3K). Only pathogenic variants according to OncoKB were included in the models. Associations among single nucleotide (SNVs) and copy number (CNVs) variations, pathway classification and previous exposure to CDK4/6i were explored through logistic regression and Gradient boosted machines (GBMs) ML algorithm. Results: at baseline, 322 pts (52.8%) were previously treated with CDK4/6i. The most detected pathway alterations were SNVs in PI3K (37.1%), P53 (31.8%), ER (29.2%) and RTK (22.3%). After stepwise logistic regression, RB1, NF1 and ESR1 SNVs were associated with previous exposure to CDK4/6i (respectively OR: 3.55 P = 0.017; OR: 3.06 P = 0.026 and OR: 1.82 P < 0.001), while SNVs in the ER pathway were associated with CDK4/6i (1.56 P < 0.001). Two GBMs models were designed based on gene variants (training AUC: 0.695, cross validation AUC: 0.631) and oncogenic pathways (training AUC: 0.713, cross validation AUC: 0.619). The highest relative importance (RI) was observed for ESR1 SNVs (RI: 35.35), TP53 SNVs (RI: 11.33), NF1 SNVs (RI: 3.45), SMAD4 SNVs (RI: 3.39) and RB1 SNVs (RI: 3.33). Alterations at a pathway level with the highest RI were ER SNVs (RI: 33.50), P53 SNVs (RI: 14.98), PI3K SNVs (RI: 14.40), RTK SNVs (RI: 10.55), RTK CNVs (RI: 10.26), cell cycle CNVs (RI: 6.99), cell cycle SNVs (RI: 6.77) and RAS SNVs (RI: 6.54). Of the previously highlighted pathway alterations, a significant impact on PFS after ctDNA collection was observed among de novo pts treated with CDK4/6i (165 pts) for ER SNVs (P < 0.0001), RTK SNVs (P = 0.0011), RTK CNVs (P = 0.0006), Cell cycle CNVs (P = 0.0010) and Cell cycle SNVs (P = 0.0143). No impact was observed on PFS for pts who had not received a CDK4/6i-based regimen. Conclusions: The combination of ctDNA-based datasets and machine learning algorithms defined novel resistance pathways for patients treated with CDK4/6i. Although preliminary, these results suggest that alterations of the ER, RTK and Cell cycle pathways might be crucial to optimize treatment strategy and drug development.
更多查看译文
关键词
breast cancer,tumor dna,ctdna,receptor-positive
AI 理解论文
溯源树
样例
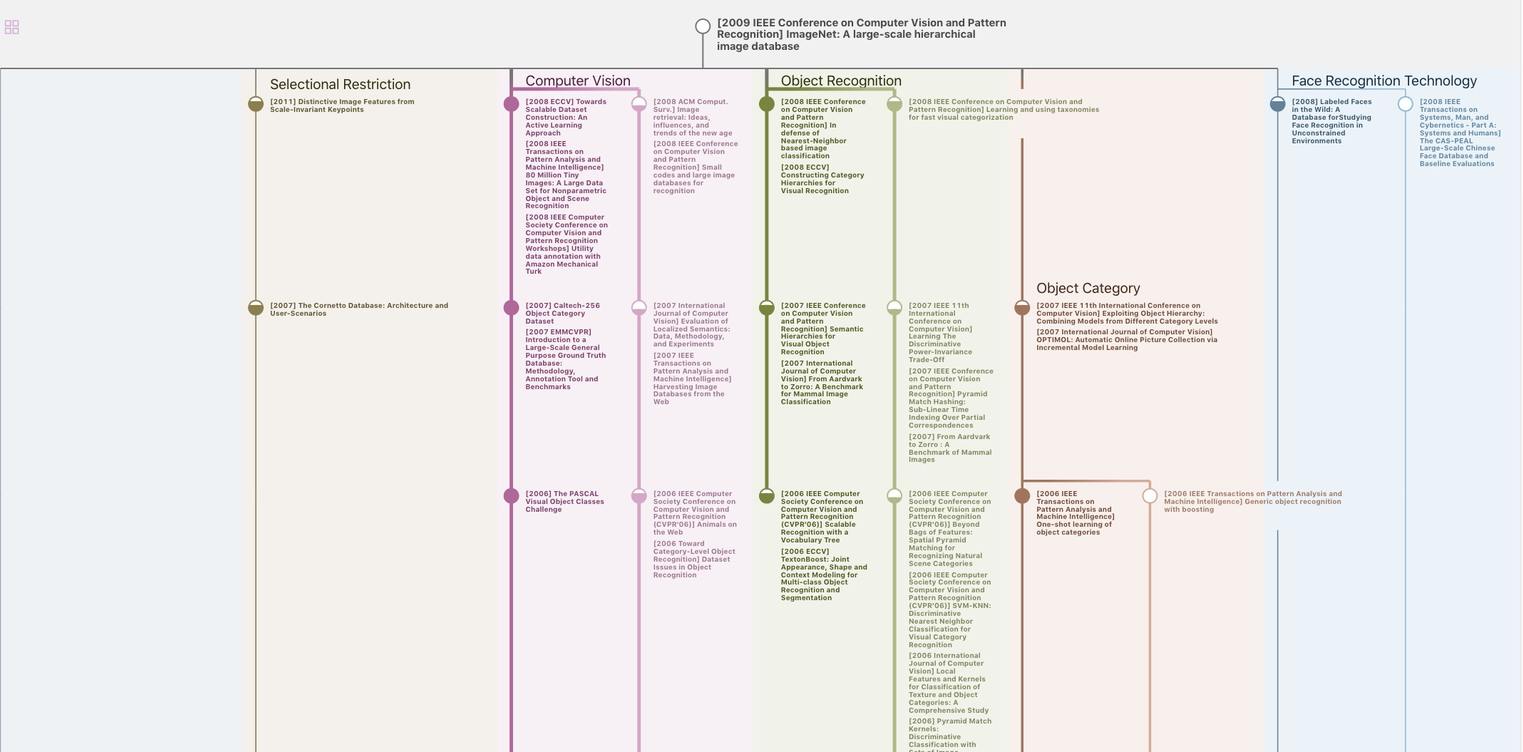
生成溯源树,研究论文发展脉络
Chat Paper
正在生成论文摘要