An Efficient Retinal Segmentation-Based Deep Learning Framework for Disease Prediction
WIRELESS COMMUNICATIONS & MOBILE COMPUTING(2022)
摘要
Deep learning (DL) technology has shown to be the most effective method of completing class assignments in the last several years. Specifically, these approaches were used for segmentation, classification, and prediction of retinal blood vessels, which was previously unattainable. U-Net deep learning technology has been hailed as one of the most significant technological advances in recent history. In the proposed work, improved segmentation of retinal images using U-Net, bidirectional ConvLSTM U-Net (BiDCU-Net), and fully connected convolutional layers, such as absolute U-Net, BiConvLSTM preferences, and also the fully connected convolutional layer method are proposed. Three well-known datasets were subjected to the suggested technique's evaluation: the DRIVE, STARE, and CHASE DB1 databases. This suggested technique was tested using the required precise measures in percentage of accuracy, F1 score, sensitivity, and specificity in DRIVE, 97.32, 83.85, 82.56, and 98.68 in CHASE, 97.44, 81.94, 83.92, and 98.45 in STARE, 97.33, 82.3, 82.12, and 98.57 in STARE, respectively. Furthermore, we assert that the strategy outperforms three other similar strategies in terms of effectiveness.
更多查看译文
关键词
disease prediction,deep learning framework,deep learning,segmentation-based
AI 理解论文
溯源树
样例
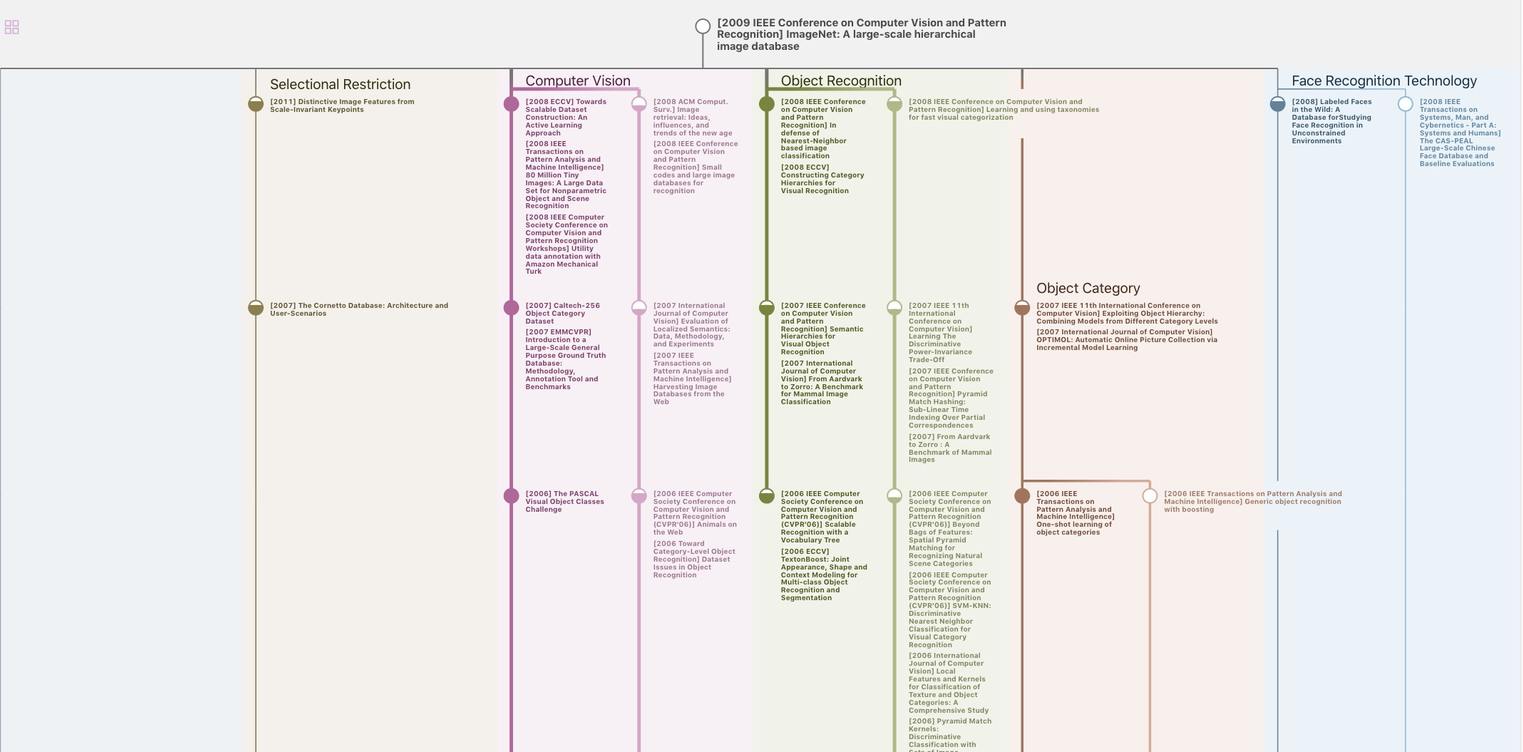
生成溯源树,研究论文发展脉络
Chat Paper
正在生成论文摘要