Deep Learning Model to Detect and Localize the Air Pollution Using Spatiotemporal Data
2022 8th International Conference on Advanced Computing and Communication Systems (ICACCS)(2022)
Abstract
Monitoring air quality is very important in our lives today. Due to the surge in the number of vehicles and industries. By monitoring air quality in advance, you can easily minimize the threat to life before you get sick. The AQI-air quality index indicates the quality of the air around us. A higher AQI means more serious air pollution. First, we need to monitor air quality at spatial and temporal resolutions. In this project, we will use a number of sensor nodes in different locations to wirelessly transfer these datasets to the host. This project details the prediction of air quality based on deep learning. This deep learning uses LSTM (long short-term memory). A multipoint deep learning model based on convolutional long short-term memory (ConvLSTM) has been proposed for highly dynamic air quality prediction. Airborne gaseous pollutant data (mainly Nitric Oxide, Nitric Dioxide, Nitric x-Oxide, Carbon Monoxide, Sulphur Dioxide) were trained and integrated for a specific period of time. All data has been converted to a specific learning format and normalized. We use LSTM to predict the value of gaseous pollutants. This article is used to validate the performance of the model against other base models. Our LSTM model outperforms all other baseline models.
MoreTranslated text
Key words
Wireless sensor network,air quality index,deep learning algorithm,map integration
AI Read Science
Must-Reading Tree
Example
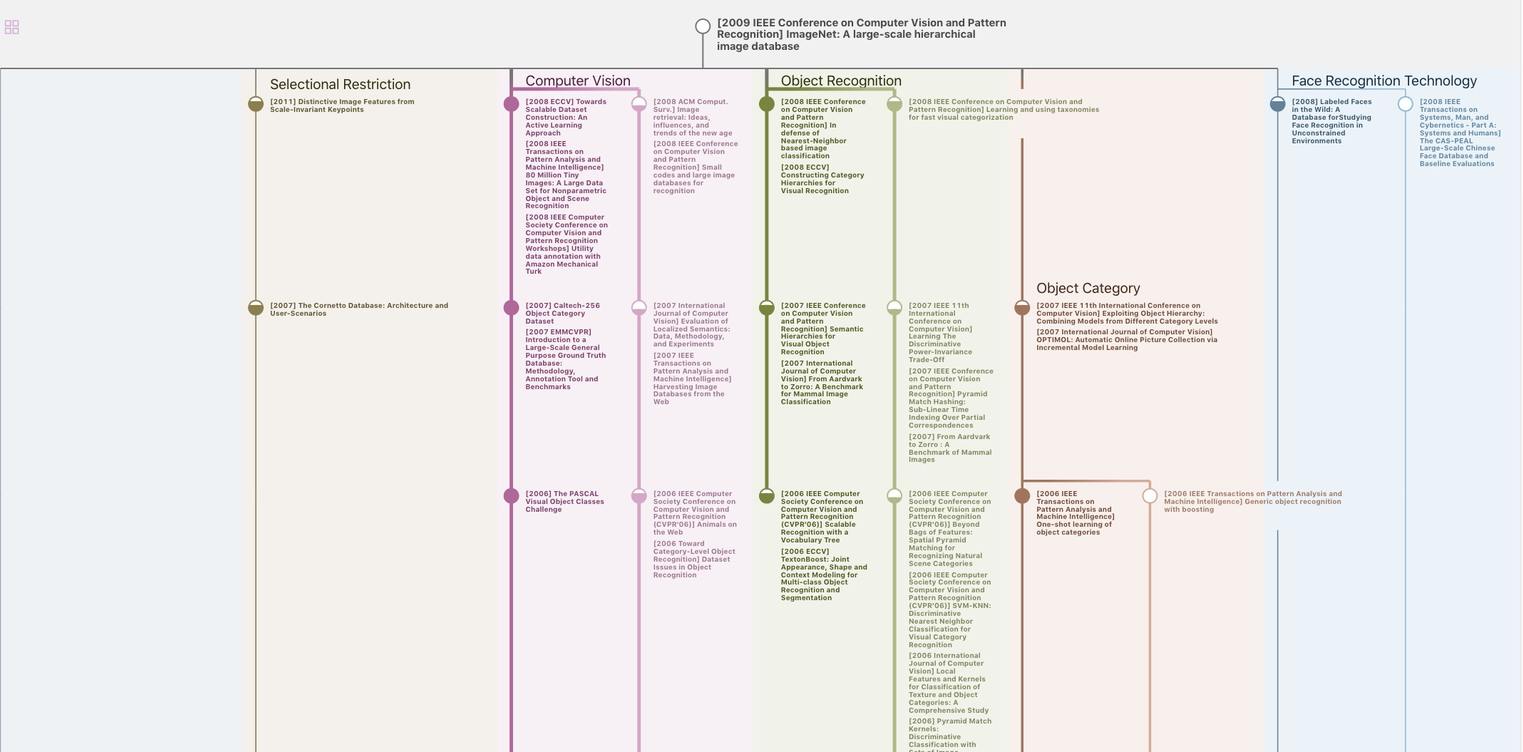
Generate MRT to find the research sequence of this paper
Chat Paper
Summary is being generated by the instructions you defined