Label distribution learning through exploring nonnegative components
Neurocomputing(2022)
摘要
Label distribution learning (LDL) is a new machine learning paradigm to solve label ambiguity and has drawn increasing attention in recent years. The importance of all labels needs to be considered under the LDL settings. A series of approaches have been proposed to deal with the LDL problem by considering the correlation of labels or instances. However, none of them focuses on finding interpretable bases to reduce the dimensions of the feature space. Inspired by the semi-nonnegative matrix factorization (semi-NMF) method, we propose a new LDL learning framework to deal with the problem through learning nonnegative components. The key insight is to explore the bases, each of which represents a class, through the label distribution and to transform the input matrix into a coefficient matrix of the space constructed by the bases. Consequently, a maximum entropy model can be adopted to learn the label distribution from the coefficient matrix. Experimental results on real-world datasets comparing our method with several state-of-the-art methods validate the performance of our approach.
更多查看译文
关键词
Label distribution learning,Nonnegative components learning
AI 理解论文
溯源树
样例
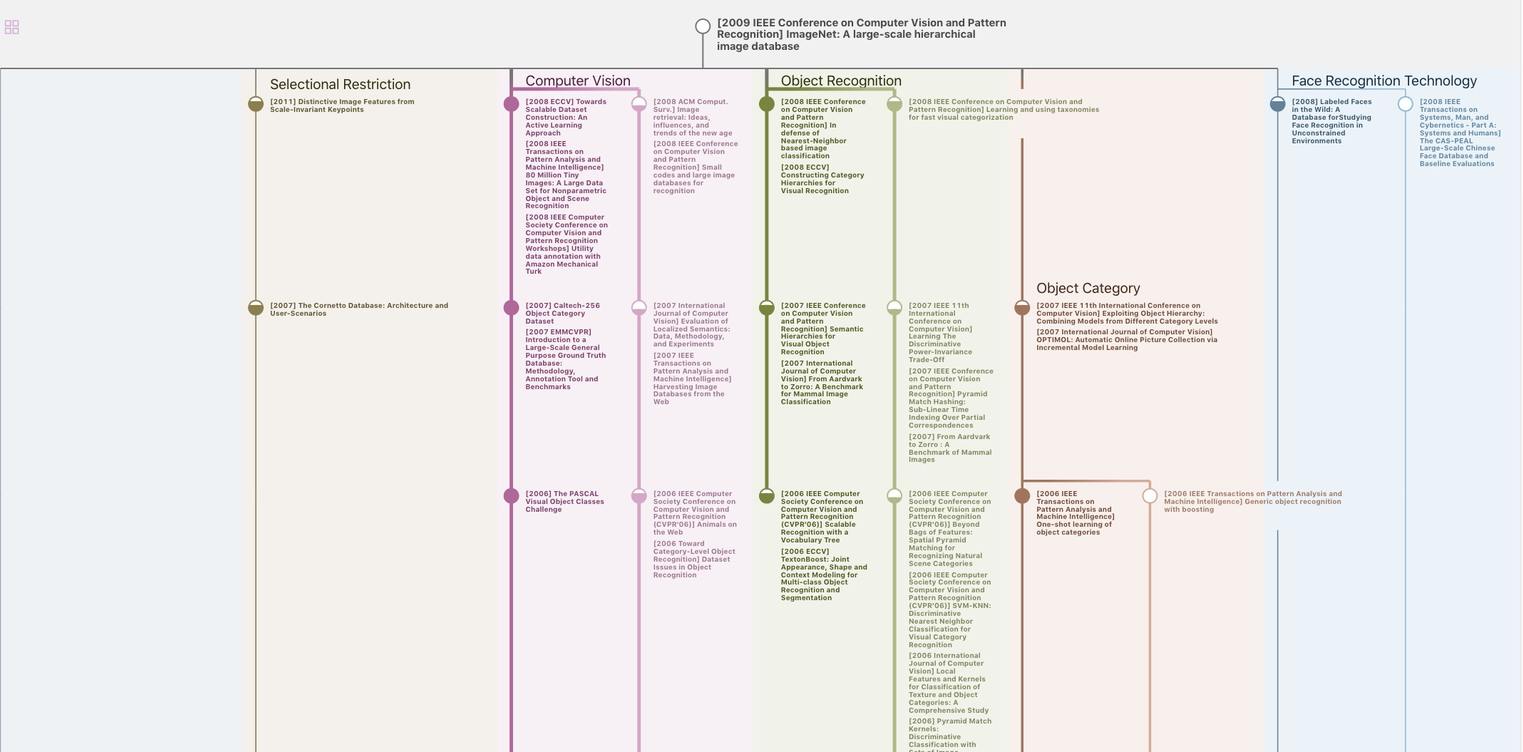
生成溯源树,研究论文发展脉络
Chat Paper
正在生成论文摘要