Explainable Hidden Markov Model for road safety: a case of road closure recommendations in extreme weather conditions
Journal of Intelligent & Fuzzy Systems(2023)
摘要
Recommendations analysis of road safety requires decision-making tools that accommodate weather uncertainties. Operation and maintenance of transport infrastructure have been one of the sub-areas that require attention due to its importance in the quality of the road. Several investigations have proposed artificial neural networks and Bayesian networks to assess the risk of the road. These methods make use of historic accident records to generate useful road safety metrics; however, there is less information on how climatic factors and road surface conditions affect the models that generate recommendations for safe traffic. In this research, Bayesian Network, as a Hidden Markov Models, and Apriori method are proposed to evaluate the open and closed state of the road. The weather and road surface conditions are explicitly written as a sequence of latent variables from observed data. Different weather variables were studied in order to evaluate both road states (open or close) and the results showed that the Hidden Markov Model provides explicit insight into the sequential nature of the road safety conditions but does not provide a directly interpretable result for human decision making. In this way, we complement the study with the Apriori algorithm using categorical variables. The experimental results show that combining the Hidden Markov Model and the Apriori algorithm provides an interpretable rule for decision making in recommendations of road safety to decide an opening or closing of the road in extreme weather conditions with a confidence higher than 90%.
更多查看译文
关键词
Road safety analysis,hidden markov models,apriori methods
AI 理解论文
溯源树
样例
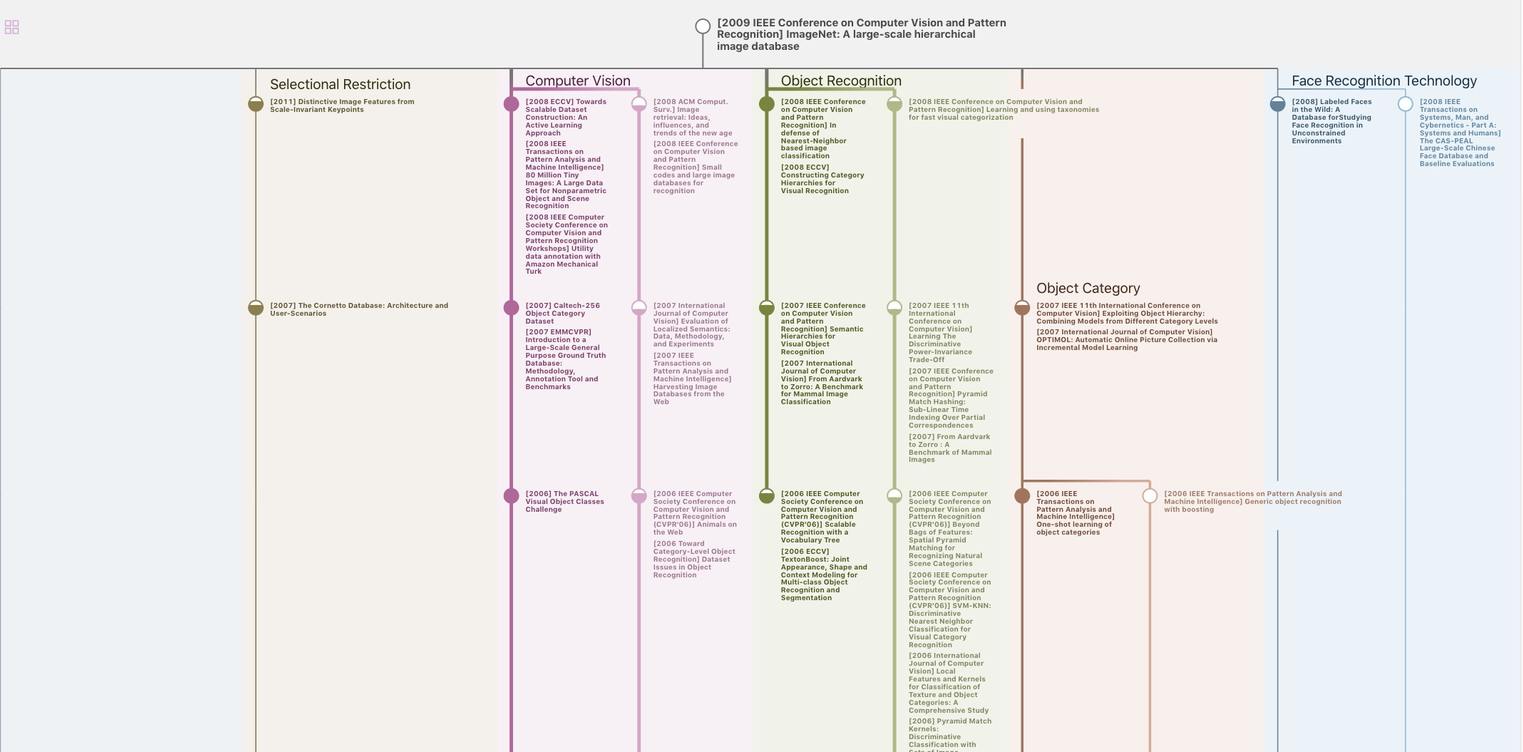
生成溯源树,研究论文发展脉络
Chat Paper
正在生成论文摘要