EEG-GCN: Spatio-Temporal and Self-Adaptive Graph Convolutional Networks for Single and Multi-View EEG-Based Emotion Recognition
IEEE SIGNAL PROCESSING LETTERS(2022)
摘要
Graph networks are naturally suitable for modeling multi-channel features of EEG signals. However, the existing study that attempts to utilize graph-based neural networks for EEG-based emotion recognition doesn't take the spatio-temporal redundancy of EEG features and differences in brain topology into account. In this paper, we propose EEG-GCN, a paradigm that adopts spatio-temporal and self-adaptive graph convolutional networks for single and multi-view EEG-based emotion recognition. With spatio-temporal attention mechanism employed, EEG-GCN can adaptively capture significant sequential segments and spatial location information in EEG signals. Meanwhile, a self-adaptive brain network adjacency matrix is designed to quantify the connection strength between the channels, in which way to represent the diverse activation patterns under different emotion scenarios. Additionally, we propose a multi-view EEG-based emotion recognition method, which effectively integrates the diverse features of EEG signals. Extensive experiments conducted on two benchmark datasets SEED and DEAP demonstrate that our proposed method outperforms other representative methods from both single and multiple views.
更多查看译文
关键词
Electroencephalography, Feature extraction, Emotion recognition, Convolution, Frequency-domain analysis, Brain modeling, Network topology, EEG, emotion recognition, graph convolutional neural network, spatio-temporal attention mechanism
AI 理解论文
溯源树
样例
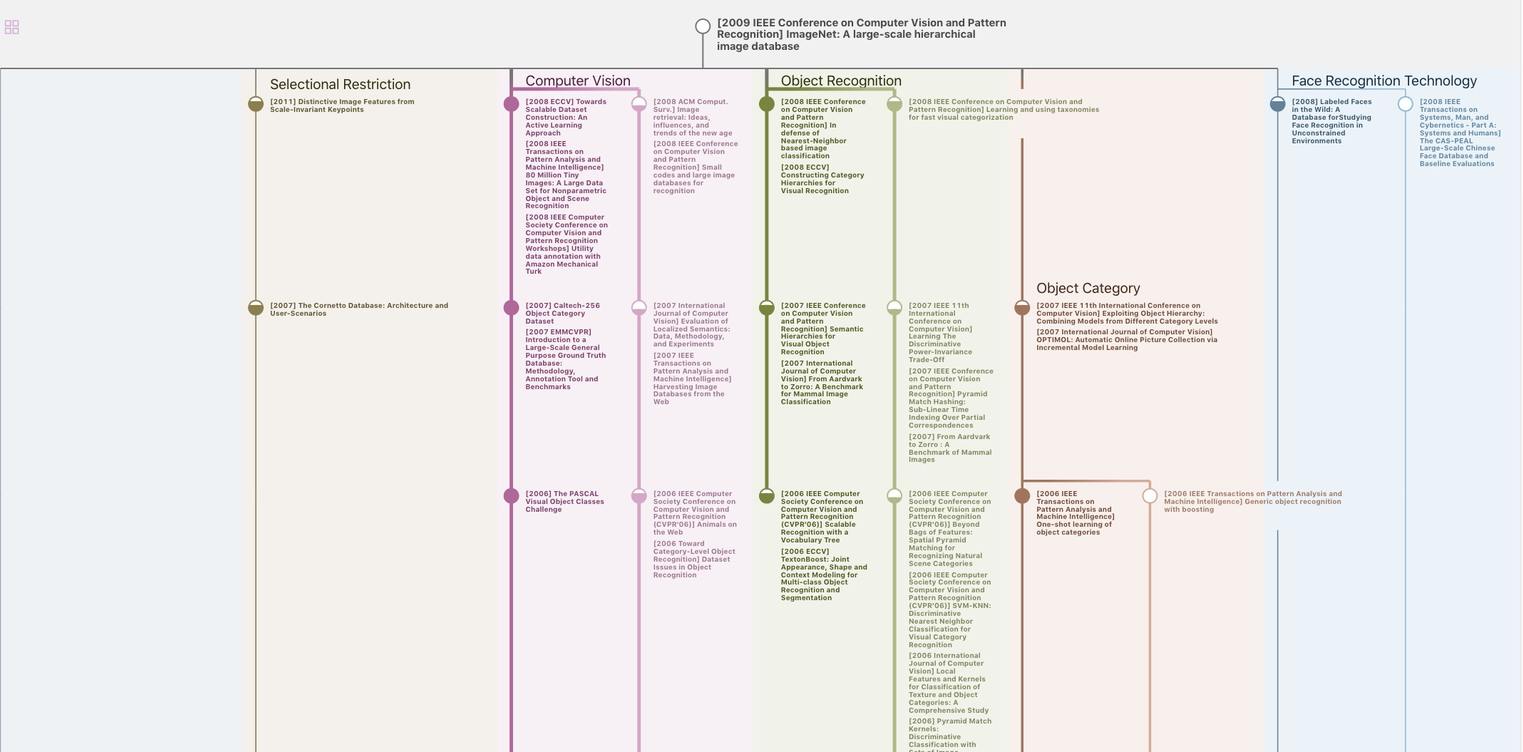
生成溯源树,研究论文发展脉络
Chat Paper
正在生成论文摘要