Fusion of Visual and Textual Features for Table Header Detection in Handwritten Text Images
2021 INTERNATIONAL CONFERENCE ON COMPUTATIONAL SCIENCE AND COMPUTATIONAL INTELLIGENCE (CSCI 2021)(2021)
Abstract
This paper introduces a new procedure to improve table header detection in handwritten text images from the fusion of the posterior probabilities provided by two baseline classifiers. Each classifier considers a different modality, namely visual or textual features. Both baseline classifiers implements convolutional neural networks, particularly adopting the U-Net architecture. Four fusion methods are considered: the mean; linear discriminant analysis and random forest as meta-classifiers; and a recently developed method called alpha integration. The testing dataset consisted of 89 page images drawn from the Passau dataset. The improved performance provided by the fusion methods in the specific experiments is interesting considering the complexity of the challenging problem approached. In terms of area under the receiver operating characteristic curve the best results were obtained by alpha integration. This method incorporates least mean square parameter optimization. The improvement is relevant in the context of the targeted problem.
MoreTranslated text
Key words
Handwritten text recognition,Table header detection,Layout analysis,Decision fusion,Alpha integration
AI Read Science
Must-Reading Tree
Example
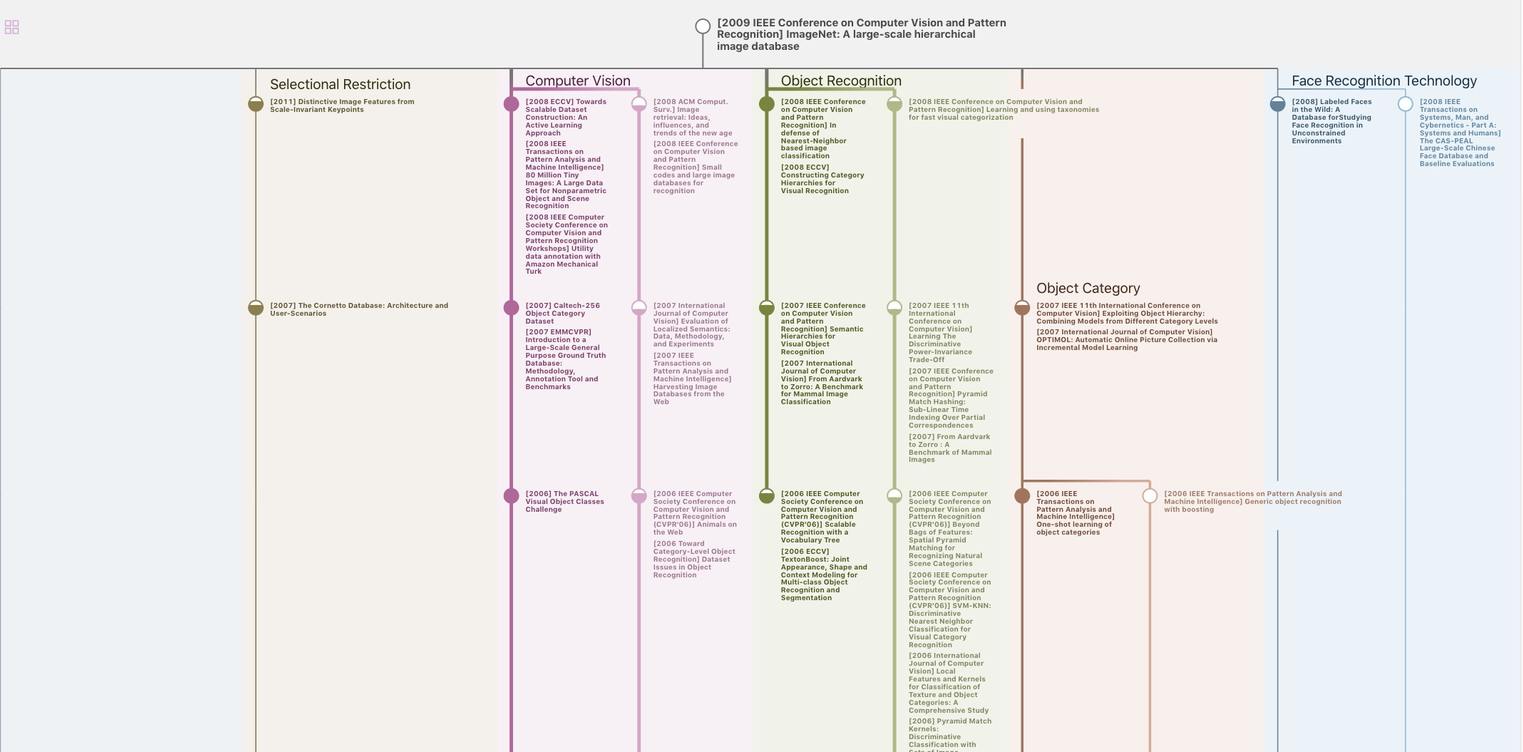
Generate MRT to find the research sequence of this paper
Chat Paper
Summary is being generated by the instructions you defined