Tradeoffs between UAS Spatial Resolution and Accuracy for Deep Learning Semantic Segmentation Applied to Wetland Vegetation Species Mapping
REMOTE SENSING(2022)
摘要
Recent advances in image classification of fine spatial resolution imagery from unoccupied aircraft systems (UASs) have allowed for mapping vegetation based on both multispectral reflectance and fine textural details. Convolutional neural network (CNN)-based models can take advantage of the spatial detail present in UAS imagery by implicitly learning shapes and textures associated with classes to produce highly accurate maps. However, the spatial resolution of UAS data is infrequently examined in CNN classification, and there are important tradeoffs between spatial resolution and classification accuracy. To improve the understanding of the relationship between spatial resolution and classification accuracy for a CNN-based model, we captured 7.6 cm imagery with a UAS in a wetland environment containing graminoid (grass-like) plant species and simulated a range of spatial resolutions up to 76.0 cm. We evaluated two methods for the simulation of coarser spatial resolution imagery, averaging before and after orthomosaic stitching, and then trained and applied a U-Net CNN model for each resolution and method. We found untuned overall accuracies exceeding 70% at the finest spatial resolutions, but classification accuracy decreased as spatial resolution coarsened, particularly beyond a 22.8 cm resolution. Coarsening the spatial resolution from 7.6 cm to 22.8 cm could permit a ninefold increase in survey area, with only a moderate reduction in classification accuracy. This study provides insight into the impact of the spatial resolution on deep learning semantic segmentation performance and information that can potentially be useful for optimizing precise UAS-based mapping projects.
更多查看译文
关键词
UAS,convolutional neural networks,semantic segmentation,deep learning,spatial resolution,classification accuracy,vegetation remote sensing,wetlands,Phragmites australis
AI 理解论文
溯源树
样例
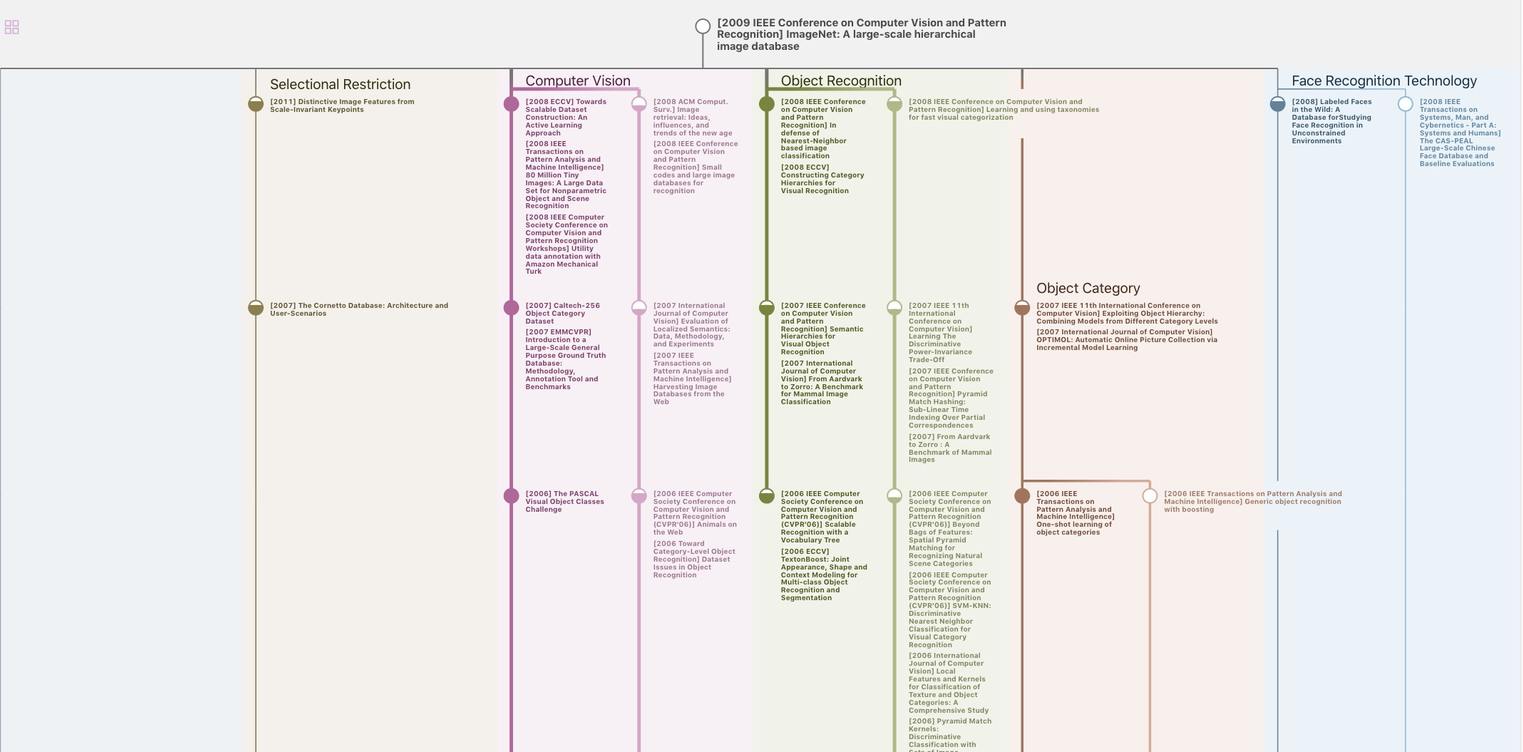
生成溯源树,研究论文发展脉络
Chat Paper
正在生成论文摘要