Machine learning enhanced electrical impedance tomography for 2D materials
INVERSE PROBLEMS(2022)
摘要
Electrical impedance tomography (EIT) is a non-invasive imaging technique that reconstructs the interior conductivity distribution of samples from a set of voltage measurements performed on the sample boundary. EIT reconstruction is a non-linear and ill-posed inverse problem. Consequently, the non-linearity results in a high computational cost of solution, while regularisation and the most informative measurements must be used to overcome ill-posedness. To build the foundation of future research into EIT applications for 2D materials, such as graphene, we designed and implemented a novel approach to measurement optimisation via a machine learning adaptive electrode selection algorithm (A-ESA). Furthermore, we modified the forward solver of a python-based EIT simulation software, pyEIT, to include the complete electrode model (CEM) and employed it on 2D square samples (Liu B et al 2018 SoftwareX 7 304-8; Somersalo E et al 1992 SIAM J. Appl. Math. 52 1023-40). In addition, the deep D-Bar U-Net convolutional neural network architecture was applied to post-process conductivity map reconstructions from the GREIT algorithm (Hamilton and Hauptmann 2018 IEEE Trans. Med. Imaging 37 2367-77; Adler et al 2009 Physiol. Meas. 30 S35). The A-ESA offered around 20% lower reconstruction losses in fewer measurements than the standard opposite-adjacent electrode selection algorithm, on both simulated data and when applied to a real graphene-based device. The CEM enhanced forward solver achieved a 3% lower loss compared to the original pyEIT forward model. Finally, an experimental evaluation was performed on a graphene laminate film. Overall, this work demonstrates how EIT could be applied to 2D materials and highlights the utility of machine learning in both the experimental and analytical aspects of EIT.
更多查看译文
关键词
GREIT algorithm,electrical impedance tomography,2D materials,machine learning,pyEIT,deep D-bar
AI 理解论文
溯源树
样例
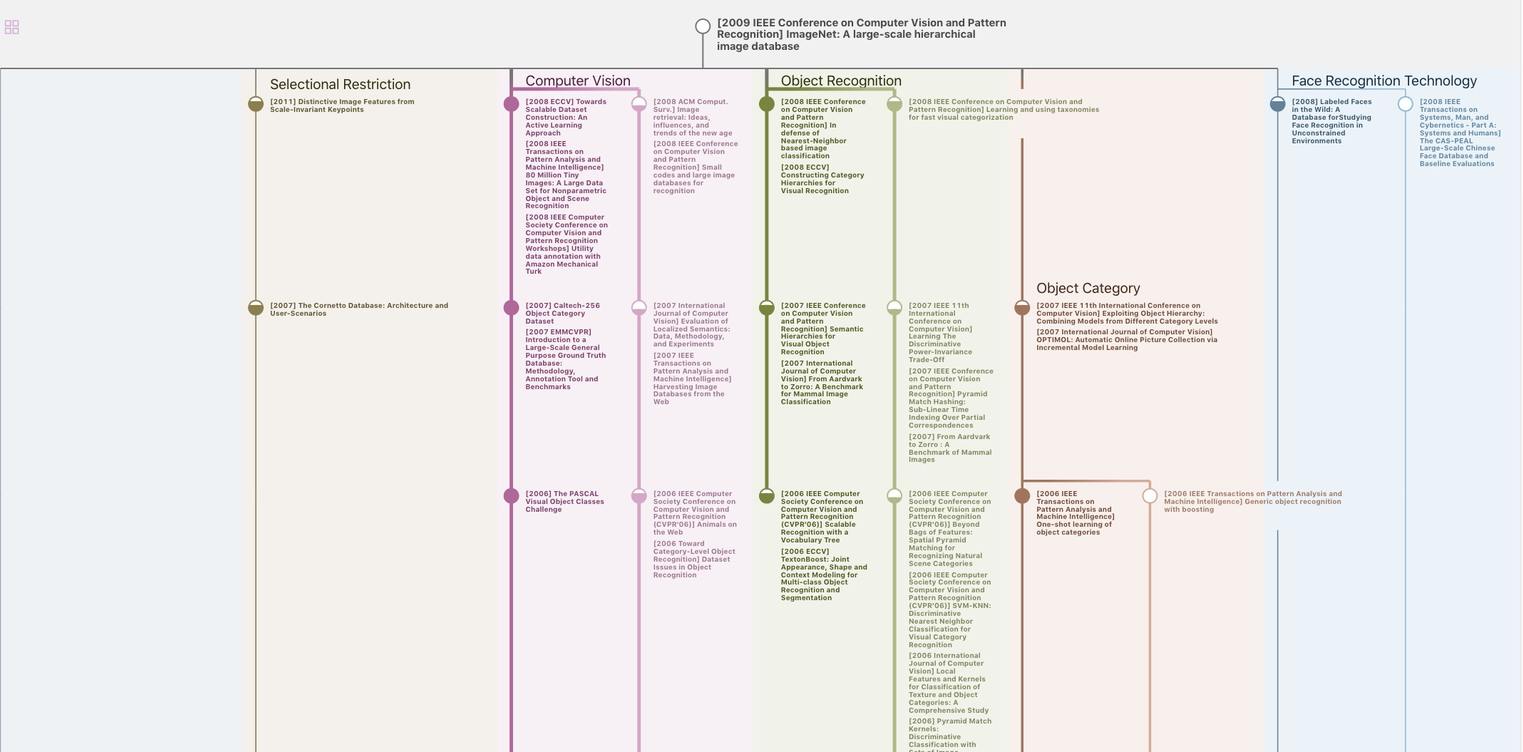
生成溯源树,研究论文发展脉络
Chat Paper
正在生成论文摘要