Ensemble Knowledge Tracing: Modeling interactions in learning process
EXPERT SYSTEMS WITH APPLICATIONS(2022)
Abstract
Knowledge Tracing (KT) aims to continuously estimate students' evolving knowledge state during their learning process, which has attracted much research attention due to its potential for delivering personalized and optimal experiences to students in intelligent learning systems. The learning process is essentially the pairwise interactions of Students, Concepts, and Questions (S-C, S-Q, C-Q for short). Modeling all these interactions will improve the performance of KT. However, existing KT methods hardly exploit all the interactions in a single model. Specifically, Bayesian Knowledge Tracing (BKT) and most of its variants neglect C-Q; Deep Knowledge Tracing (DKT) and other deep neural network approaches mostly neglect S-Q and C-Q. We propose the Ensemble Knowledge Tracing (EnKT), which models all three types of interactions. The base model of EnKT is a hybrid of BKT and DKT. We also present an ensemble algorithm Recurrent Boosting (RB), which extends AdaBoost to deal with KT sequential data. Inspired by BKT, EnKT represents S-C and S-Q using learning and performance parameters, respectively. Besides, EnKT defines C-Q as the correlation complexity among the concepts involved in a question. Experiments show EnKT significantly outperforms state-of-the-art methods (by up to 6% in AUC in some cases) on four real-world benchmark datasets and illustrate better interpretability by several typical case studies.
MoreTranslated text
Key words
KnowledgeTracing,Deepneuralnetwork,Learninginteractions,Usermodeling
AI Read Science
Must-Reading Tree
Example
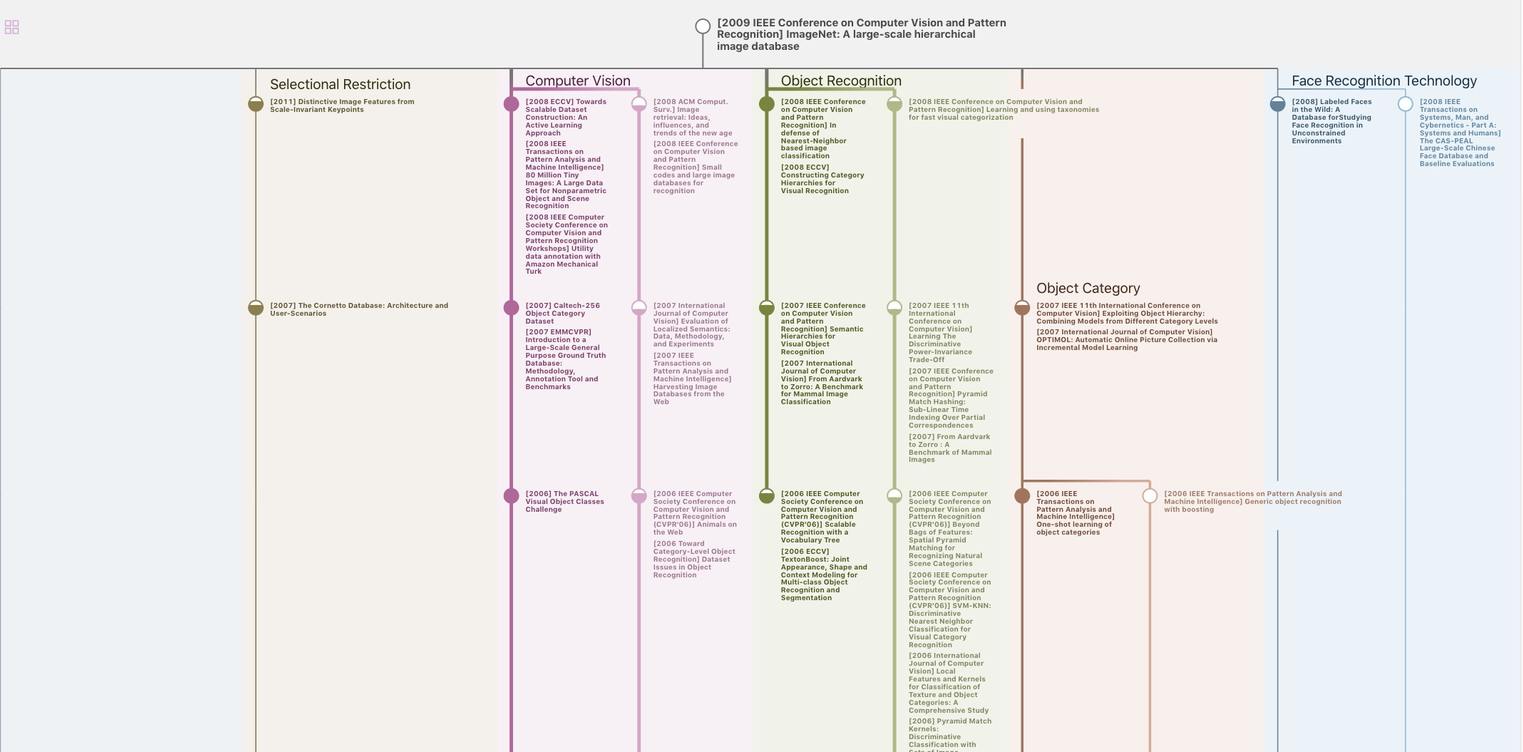
Generate MRT to find the research sequence of this paper
Chat Paper
Summary is being generated by the instructions you defined