Self-Heating of FinFET Circuitry Simulated by Multi-Correlated Recurrent Neural Networks
IEEE Electron Device Letters(2022)
摘要
A series of multi-correlated recurrent neural networks is used to predict the relative temperature of inverter chains folded in 3 rows. The circuit hotspot temperature is predicted by a fully connected neural network. The correlated recurrent neural networks trained by the SPICE data within 17 stages can predict
$ {T}$
up to 37 stages (
$2.2\times $
SPICE complexity) with the error as low as 0.9 °C, outperforming the previous fully connected neural network (
$1.9\times $
SPICE, 3 °C error) and non-correlated recurrent neural network (
$2.2\times $
SPICE, 3 °C error) by considering the thermal coupling between rows. The precise prediction of temperature profiles and hotspot positions indicate that the thermal physics is learned by correlated recurrent neural networks. Therefore, an 82-stage folded inverter chain can be predicted and optimized confidently by neural networks, while SPICE can only simulate a 37-stage chain due to the high computational cost. A 100-stage chain is also predicted.
更多查看译文
关键词
FinFETs,self-heating,chain circuit,neural network
AI 理解论文
溯源树
样例
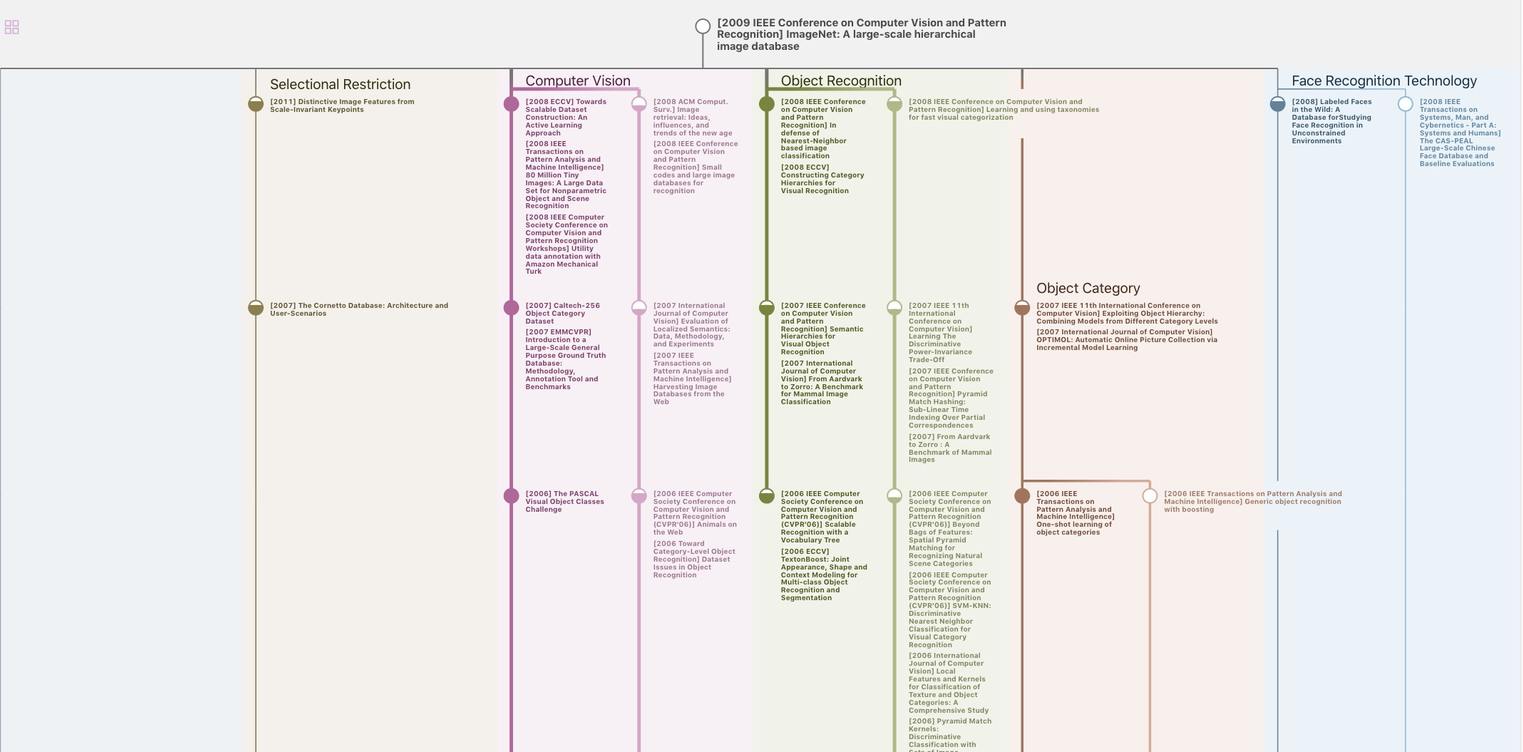
生成溯源树,研究论文发展脉络
Chat Paper
正在生成论文摘要