An explainable semi-personalized federated learning model
INTEGRATED COMPUTER-AIDED ENGINEERING(2022)
摘要
Training a model using batch learning requires uniform data storage in a repository. This approach is intrusive, as users have to expose their privacy and exchange sensitive data by sending them to central entities to be preprocessed. Unlike the aforementioned centralized approach, training of intelligent models via the federated learning (FEDL) mechanism can be carried out using decentralized data. This process ensures that privacy and protection of sensitive information can be managed by a user or an organization, employing a single universal model for all users. This model should apply average aggregation methods to the set of cooperative training data. This raises serious concerns for the effectiveness of this universal approach and, therefore, for the validity of FEDL architectures in general. Generally, it flattens the unique needs of individual users without considering the local events to be managed. This paper proposes an innovative hybrid explainable semi-personalized federated learning model, that utilizes Shapley Values and Lipschitz Constant techniques, in order to create personalized intelligent models. It is based on the needs and events that each individual user is required to address in a federated format. Explanations are the assortment of characteristics of the interpretable system, which, in the case of a specified illustration, helped to bring about a conclusion and provided the function of the model on both local and global levels. Retraining is suggested only for those features for which the degree of change is considered quite important for the evolution of its functionality.
更多查看译文
关键词
Decentralized learning,federated learning,privacy-preserving architecture,explainable AI,local and global interpretability,shapley values,lipschitz constant
AI 理解论文
溯源树
样例
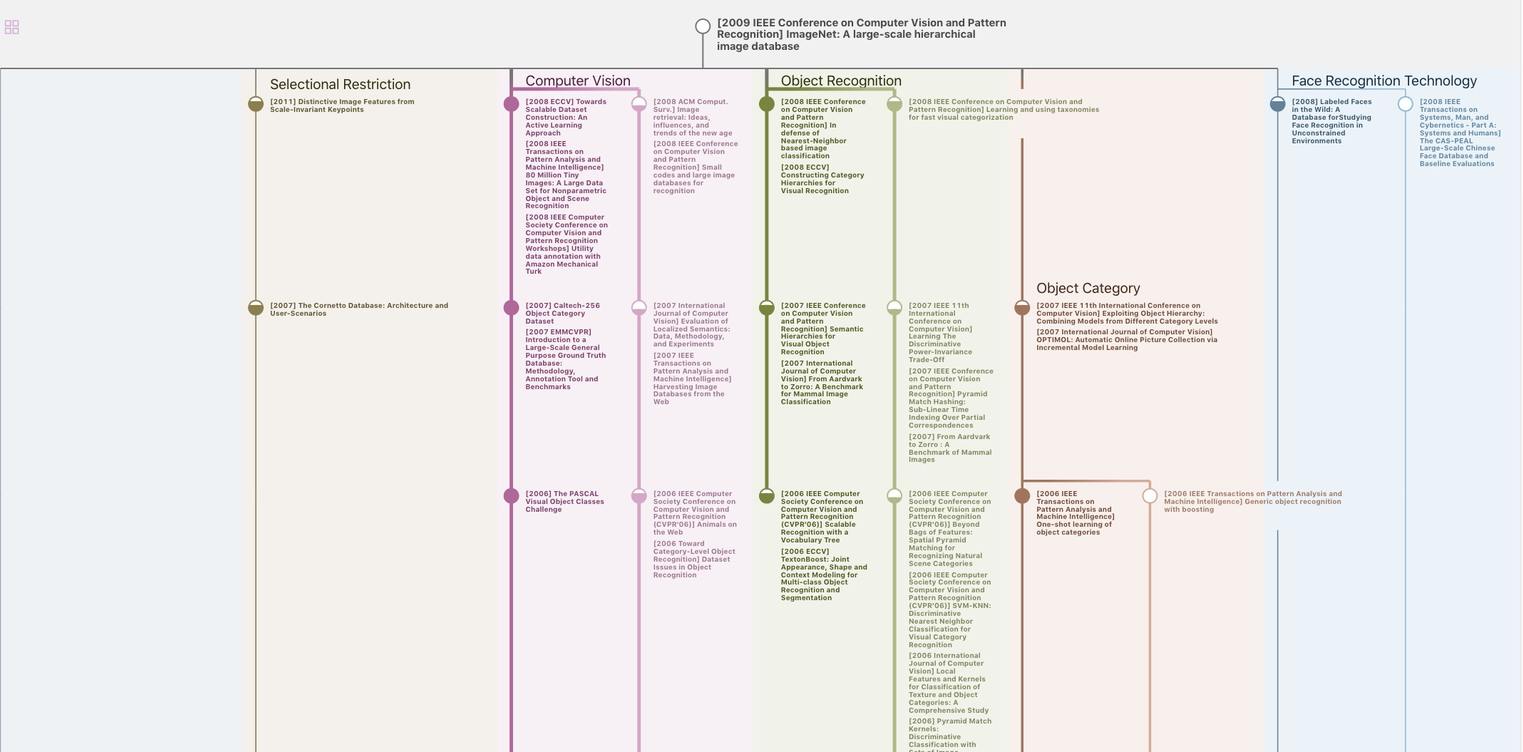
生成溯源树,研究论文发展脉络
Chat Paper
正在生成论文摘要