Abstract LB119: Precision combination therapies based on recurrent oncogenic co-alterations
Cancer Research(2022)
摘要
Abstract Cancer cells depend on multiple driver alterations whose oncogenic effects can be suppressed by drug combinations. Discovering effective combination therapies and selecting patients to maximize therapeutic benefit are challenging due to the complexity of the molecular landscape of drug responses. Here, we provide a comprehensive resource of precision combination therapies tailored to oncogenic co-alterations that are recurrent across patient cohorts. To generate the resource, we developed REcurrent Features Leveraged for Combination Therapy (REFLECT), which integrates machine learning and cancer informatics algorithms. Based on multi-omic data, the method maps recurrent co-alteration signatures in patient cohorts to combination therapies. The resource matches > 2,000 drug combinations to co-alteration signatures across 201 cohorts stratified from 10,392 patients and 33 cancer types. We validated the REFLECT pipeline using data from patient-derived xenografts (PDX), in vitro drug screens, and combination therapy clinical trials. These validations demonstrate that REFLECT selects combination therapies with significantly improved efficacy, drug synergy, and survival outcomes. In patient cohorts with immunotherapy response markers, HER2 activation, and DNA repair aberrations, we have identified therapeutically actionable and recurrent co-alteration signatures. REFLECT provides a resource and framework to design combination therapies tailored to tumor cohorts in data-driven clinical trials and pre-clinical studies. Citation Format: Anil Korkut, Xubin Li, Elizabeth Kong, Gonghong Yan, Zeynep Dereli, Behnaz Bozorgui, Parisa Imanirad, Jacob Elnaggar, Augustin Luna, David Menter, Patrick Pilié, Timothy Yap, Scott Kopetz, Chris Sander. Precision combination therapies based on recurrent oncogenic co-alterations [abstract]. In: Proceedings of the American Association for Cancer Research Annual Meeting 2022; 2022 Apr 8-13. Philadelphia (PA): AACR; Cancer Res 2022;82(12_Suppl):Abstract nr LB119.
更多查看译文
AI 理解论文
溯源树
样例
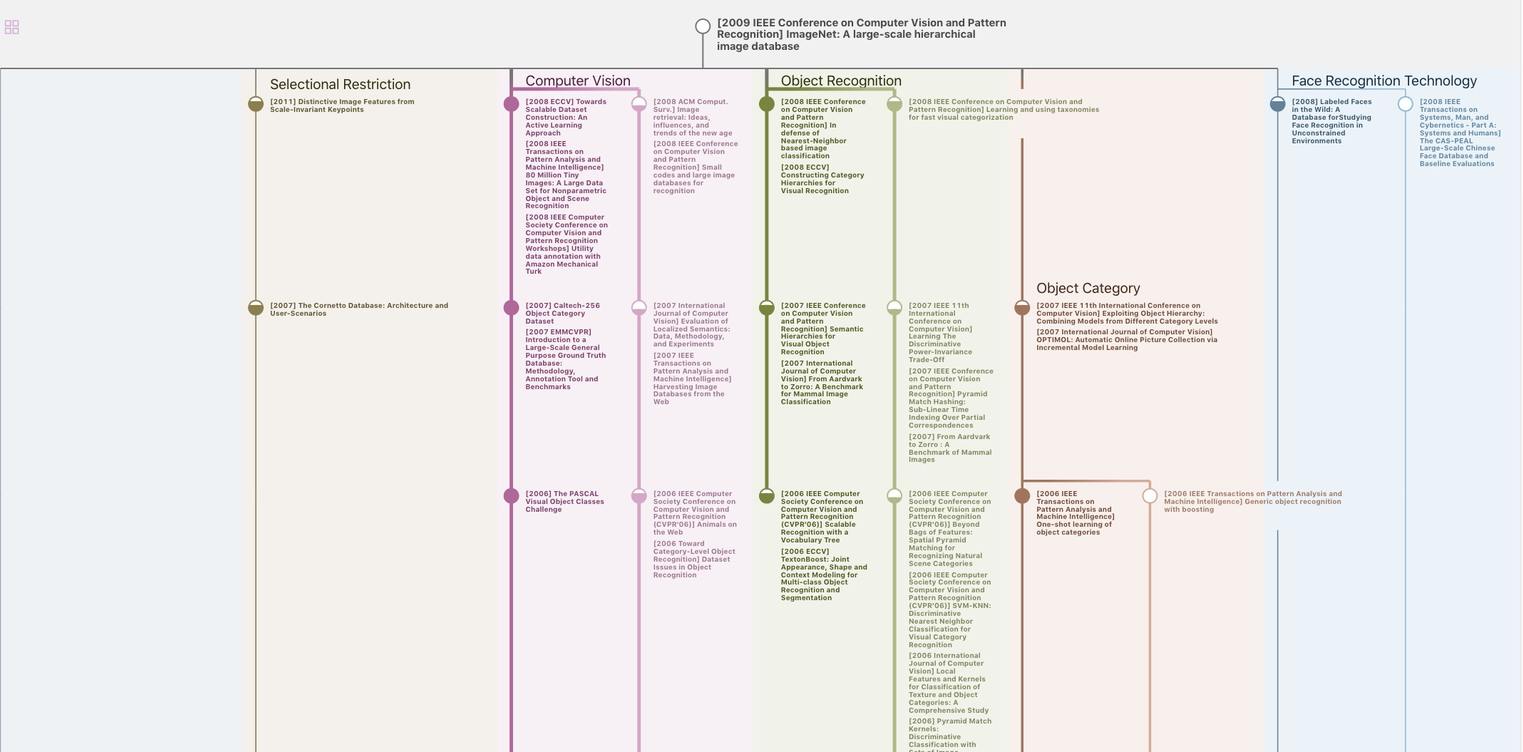
生成溯源树,研究论文发展脉络
Chat Paper
正在生成论文摘要