UAV-based indicators of crop growth are robust for distinct water and nutrient management but vary between crop development phases
Field Crops Research(2022)
Abstract
Unmanned aerial vehicle (UAV) remote sensing and machine learning have emerged as a practical approach with ultra-high temporal and spatial resolutions to overcome the limitations of ground-based sampling for continuous crop monitoring. However, little is known on the suitability of distinct sensing indices for different crop management and distinct crop development phases. In this study, we assessed the potential of the UAV-based modeling to monitor field-scale crop growth under different water and nutrient supply considering distinct phenological phases of maize. UAV multispectral observations were deployed over two long-term experimental sites in three growing seasons. Calibration and validation of the random forest model took place at the Nutrient Balance Experimental Site (NBES) and the Water Nitrogen Crop Relation Site (WNCR), respectively. Leaf area index, leaf chlorophyll concentration, and aboveground dry matter were measured at the jointing, heading, and grain filling phases of maize in 2018–2020. Our results revealed that the suitability of sensing indicators differed at distinct maize phenological phases. Overall, red edge, red edge reflectance ratio, and chlorophyll index green are the most appropriate UAV indicators for estimating maize growth variables. The random forest model developed and calibrated at NBES with nutrient supply detected the signal of nitrogen × irrigation interactions at the other experimental site (WNCR) in different development phases and years very well, suggesting that random forest models developed by UAV images of same spatial and spectral attributes could be transferred across sites with the same cultivar while different irrigation and fertilizer management. We conclude that the selected number of UAV detected indicators processed with a random forest model could be used for robustly estimating environment × management (fertilizer and irrigation) interactions on maize growth variables.
MoreTranslated text
Key words
AGDM,CIG,cv-RMSE,EVI,GNDVI,LAI,LCC,MCARI,MEAN,MAPE,MSAVI,NA,NBES,NDVI,NDRE,NIR,NS,OSAVI,R2,RD1,RE,RFE,RMSE,RQ,RVI,SAVI,SR,SRRE,UAV,WNCR,WNS
AI Read Science
Must-Reading Tree
Example
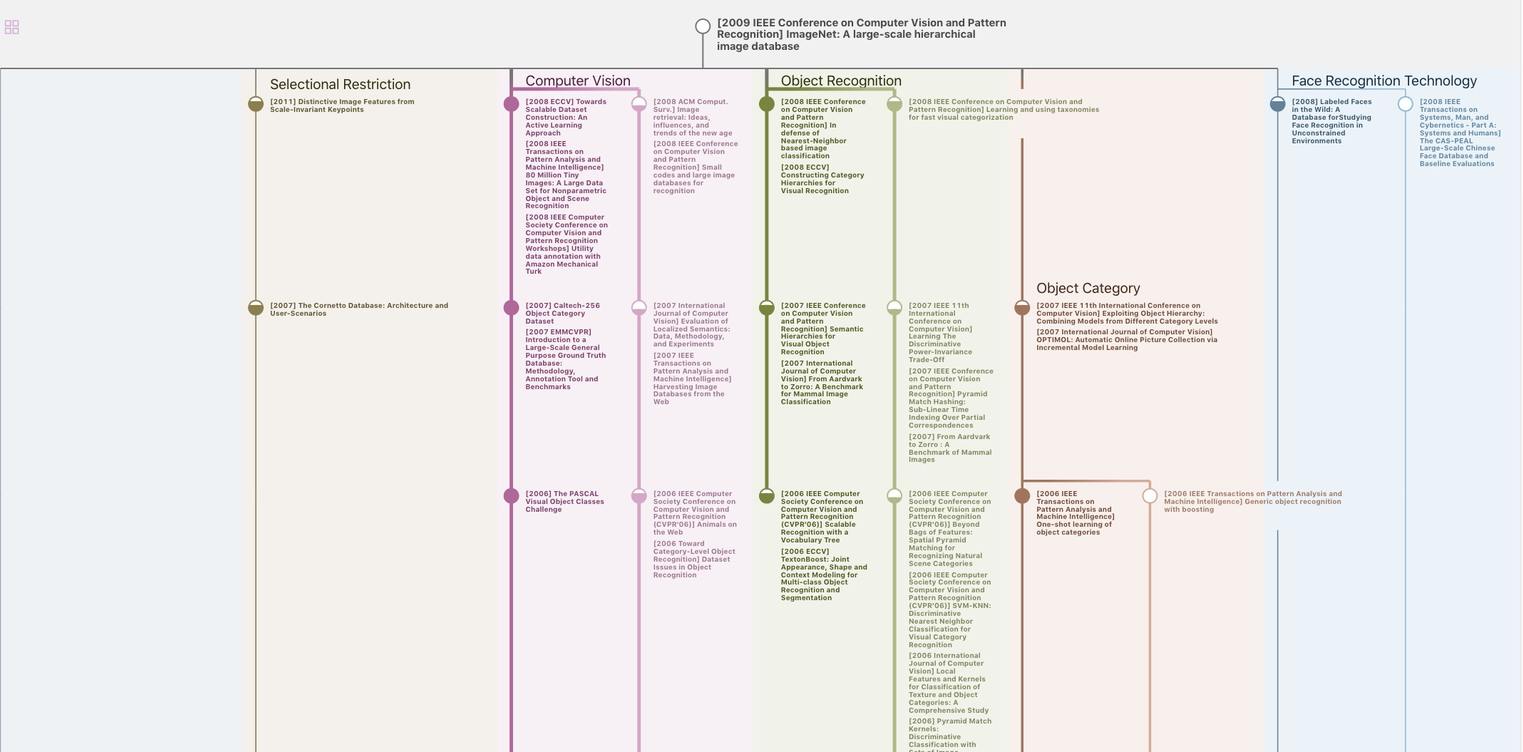
Generate MRT to find the research sequence of this paper
Chat Paper
Summary is being generated by the instructions you defined