Real-world data on the clinical utility of a novel artificial intelligence-based computational method to support treatment decisions in gastrointestinal cancers.
Journal of Clinical Oncology(2022)
摘要
e13616 Background: Several molecularly targeted agents (MTA) are registered in the same indication, even in the same line, to treat gastrointestinal (GI) cancers. Comprehensive molecular profiling is readily available for many patients, but only a few biomarkers are used to make clinical decisions between on-label MTAs. Most tumors harbor a combination of driver alterations potentially affecting the sensitivity to MTAs according to hundreds or thousands of evidence items that can explain the heterogeneous clinical response of individual patients to these therapies. We have developed an AI-based computational method, an automated, evidence-based reasoning framework, called digital drug assignment (DDA) to provide decision support based on the complete molecular profile for each patient. DDA has been shown to potentially improve treatment decisions in case of complex molecular profiles acquired in the SHIVA01 trial. In the present study, we analyzed the clinical utility of DDA in on-label treatment decisions in GI cancers. Methods: We analyzed the next-generation sequencing (NGS) data obtained using 50-591-gene panels of 239 patients with GI tract cancers from our everyday practice. Molecular profiles were uploaded to a DDA-based software system to calculate the aggregated evidence level (AEL) values of associated MTAs registered in the same indication of selected GI cancers. Decision support rate (DSR) was calculated as the percentage of patients where DDA could support clinical decisions based on the presence of genetic alterations associated with any of the available MTAs. Results: Targeted treatment options registered in the same indications and the DDA-based decision support rates (DSR) are shown in the table. For example, DDA could support the decision between cetuximab and bevacizumab based on NGS results in 47% of RAS/RAF wild-type colorectal cancer cases. Larger NGS panels yielded higher rates of decision support (44% vs 52% with gene panels under and over 300 genes, respectively). Conclusions: DDA-based computational reasoning can support molecular profile-based treatment decisions among different on-label MTAs in a clinically meaningful fraction of GI cancers.[Table: see text]
更多查看译文
关键词
gastrointestinal cancers,treatment decisions,real-world,intelligence-based
AI 理解论文
溯源树
样例
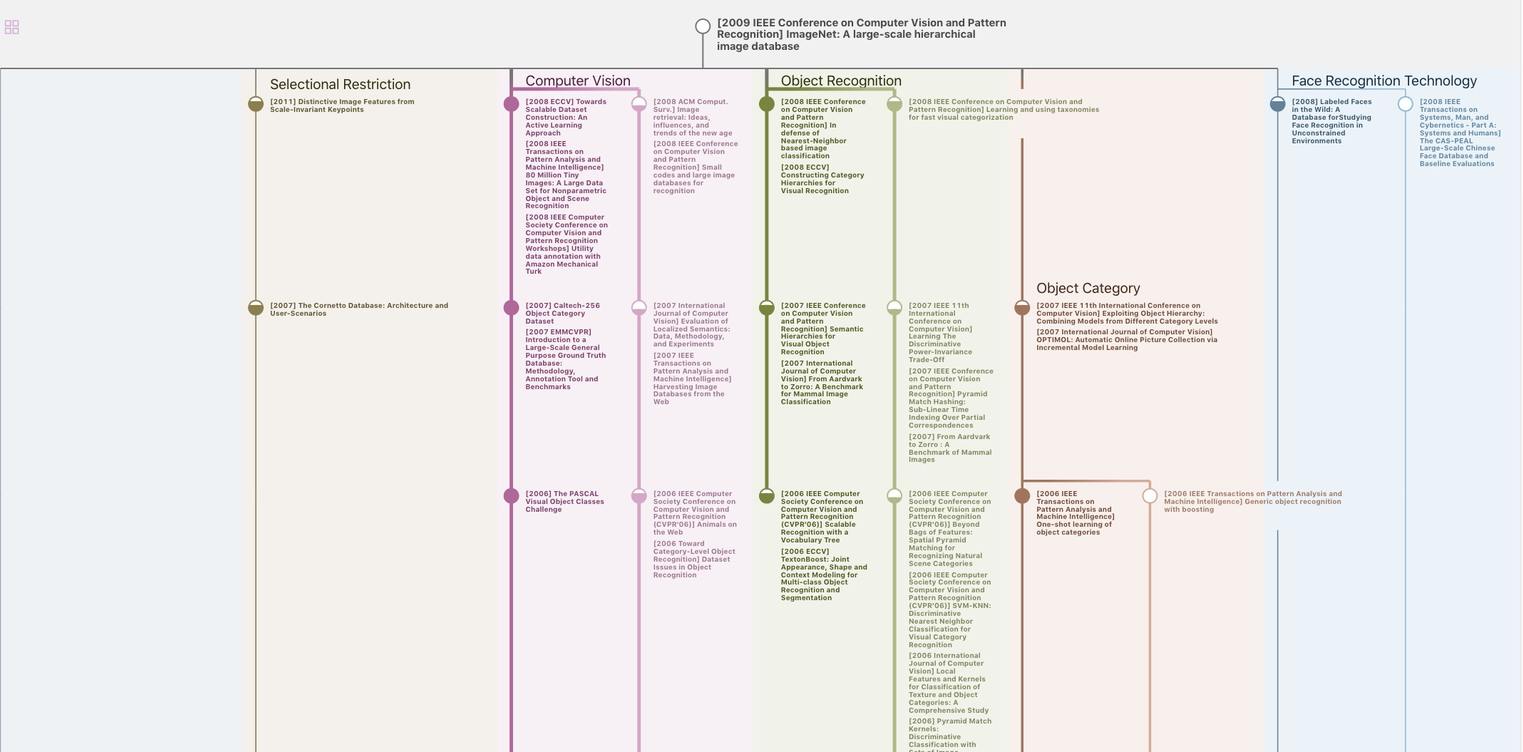
生成溯源树,研究论文发展脉络
Chat Paper
正在生成论文摘要