Joint-Channel-Connectivity-Based Feature Selection and Classification on fNIRS for Stress Detection in Decision-Making
IEEE TRANSACTIONS ON NEURAL SYSTEMS AND REHABILITATION ENGINEERING(2022)
Abstract
Stress is one of the contributing factors affecting decision-making. Therefore, early stress recognition is essential to improve clinicians' decision-making performance. Functional near-infrared spectroscopy (fNIRS) has shown great potential in detecting stress. However, the majority of previous studies only used fNIRS features at the individual level for classification without considering the correlations among channels corresponding to the brain, which may provide distinguishing features. Hence, this study proposes a novel joint-channel-connectivity-based feature selection and classification algorithm for fNIRS to detect stress in decision-making. Specifically, this approach integrates feature selection and classifier modeling into a sparse model, where intra- and inter-channel regularizers are designed to explore potential correlations among channels to obtain discriminating features. In this paper, we simulated the decision-making of medical students under stress through the Trier Social Stress Test and the Balloon Analog Risk Task and recorded their cerebral hemo- dynamic alterations by fNIRS device. Experimental results illustrated that our method with the accuracy of 0.961 is superior to other machine learning methods. Additionally, the stress correlation and connectivity of brain regions calculated by feature selection have been confirmed in previous studies, which validates the effectiveness of our method and helps optimize the channel settings of fNIRS. This work was the first attempt to utilize a sparse model that simultaneously considers the sparsity of features and the correlation of brain regions for stress detection and obtained an admirable classification performance. Thus, the proposed model might be a useful tool for medical personnel to automatically detect stress in clinical decision-making situations.
MoreTranslated text
Key words
Functional near-infrared spectroscopy, stress detection, decision-making, sparse model
AI Read Science
Must-Reading Tree
Example
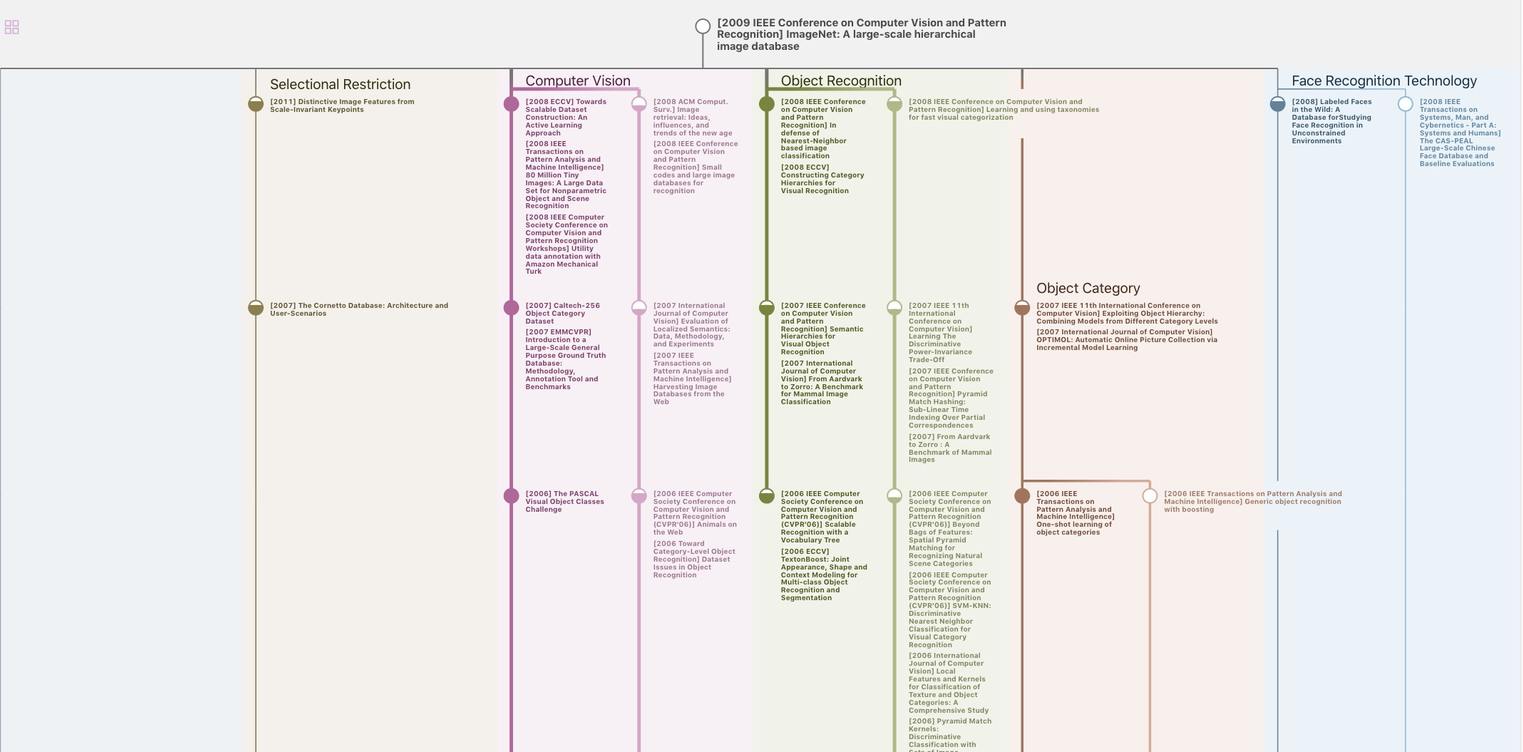
Generate MRT to find the research sequence of this paper
Chat Paper
Summary is being generated by the instructions you defined