Federated Self-supervised Learning for Video Understanding.
European Conference on Computer Vision(2022)
摘要
The ubiquity of camera-enabled mobile devices has lead to large amounts of unlabelled video data being produced at the edge. Although various self-supervised learning (SSL) methods have been proposed to harvest their latent spatio-temporal representations for task-specific training, practical challenges including privacy concerns and communication costs prevent SSL from being deployed at large scales. To mitigate these issues, we propose the use of Federated Learning (FL) to the task of video SSL. In this work, we evaluate the performance of current state-of-the-art (SOTA) video-SSL techniques and identify their shortcomings when integrated into the large-scale FL setting simulated with kinetics-400 dataset. We follow by proposing a novel federated SSL framework for video, dubbed FedVSSL, that integrates different aggregation strategies and partial weight updating. Extensive experiments demonstrate the effectiveness and significance of FedVSSL as it outperforms the centralized SOTA for the downstream retrieval task by 6.66% on UCF-101 and 5.13% on HMDB-51.
更多查看译文
关键词
Self-supervised learning, Federated learning, Video understanding, Model aggregation
AI 理解论文
溯源树
样例
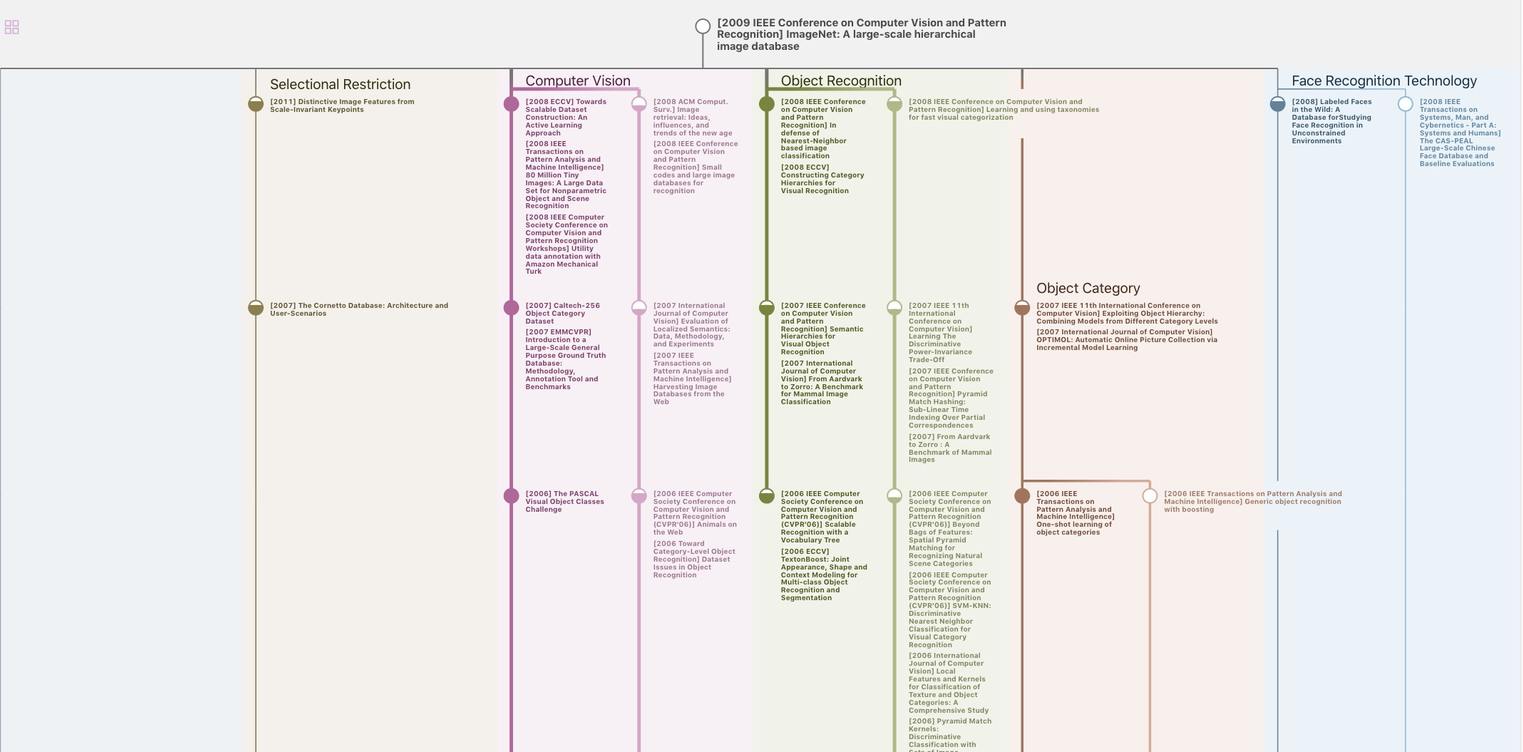
生成溯源树,研究论文发展脉络
Chat Paper
正在生成论文摘要