Bayesian approaches for Quantifying Clinicians' Variability in Medical Image Quantification
CoRR(2022)
Abstract
Medical imaging, including MRI, CT, and Ultrasound, plays a vital role in clinical decisions. Accurate segmentation is essential to measure the structure of interest from the image. However, manual segmentation is highly operator-dependent, which leads to high inter and intra-variability of quantitative measurements. In this paper, we explore the feasibility that Bayesian predictive distribution parameterized by deep neural networks can capture the clinicians' inter-intra variability. By exploring and analyzing recently emerged approximate inference schemes, we evaluate whether approximate Bayesian deep learning with the posterior over segmentations can learn inter-intra rater variability both in segmentation and clinical measurements. The experiments are performed with two different imaging modalities: MRI and ultrasound. We empirically demonstrated that Bayesian predictive distribution parameterized by deep neural networks could approximate the clinicians' inter-intra variability. We show a new perspective in analyzing medical images quantitatively by providing clinical measurement uncertainty.
MoreTranslated text
AI Read Science
Must-Reading Tree
Example
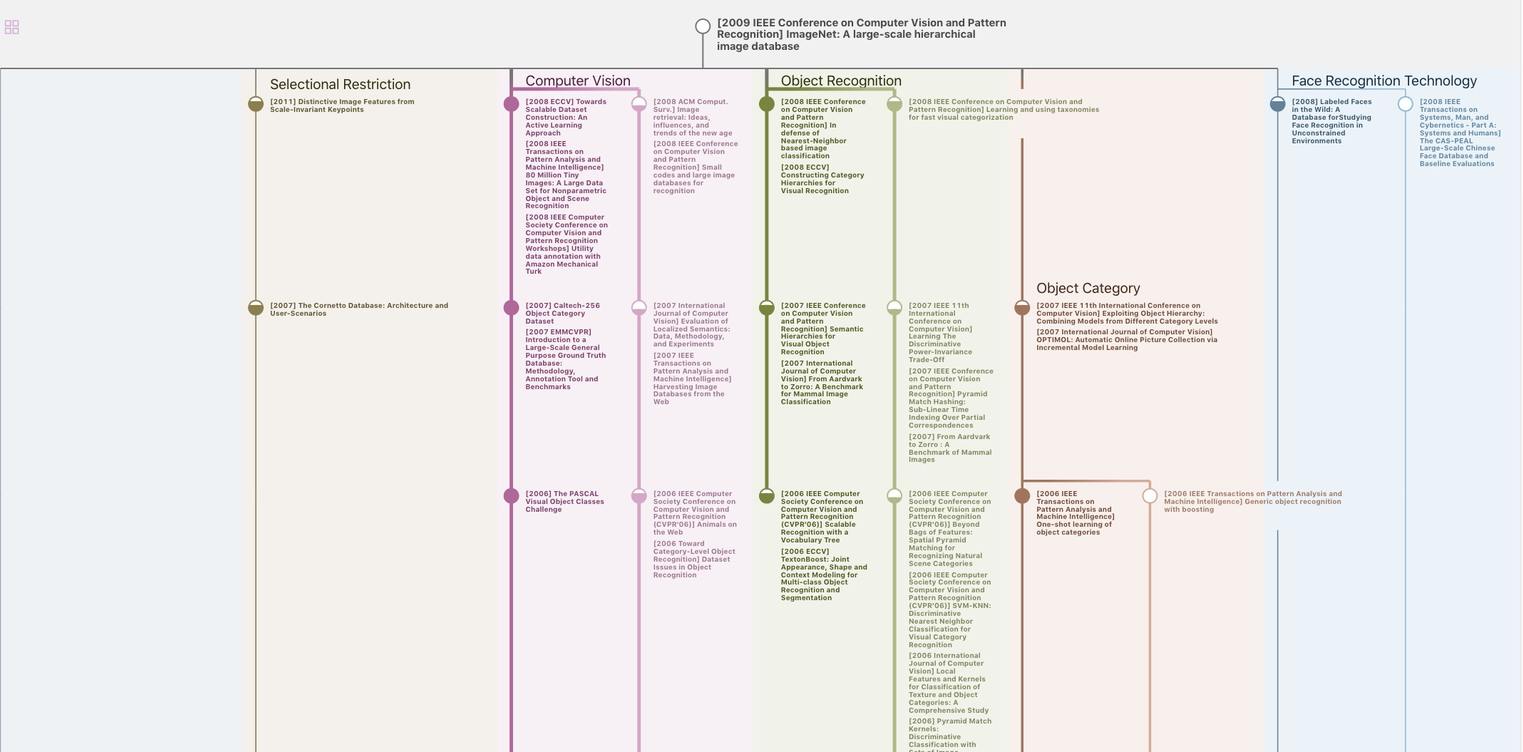
Generate MRT to find the research sequence of this paper
Chat Paper
Summary is being generated by the instructions you defined