Accurate Instance-Level CAD Model Retrieval in a Large-Scale Database.
IEEE/RJS International Conference on Intelligent RObots and Systems (IROS)(2022)
摘要
We present a new solution to the fine-grained retrieval of clean CAD models from a large-scale database in order to recover detailed object shape geometries for RGBD scans. Unlike previous work simply indexing into a moderately small database using an object shape descriptor and accepting the top retrieval result, we argue that in the case of a large-scale database a more accurate model may be found within a neighborhood of the descriptor. More importantly, we propose that the distinctiveness deficiency of shape descriptors at the instance level can be compensated by a geometry-based re-ranking of its neighborhood. Our approach first leverages the discriminative power of learned representations to distinguish between different categories of models and then uses a novel robust point set distance metric to re-rank the CAD neighborhood, enabling fine-grained retrieval in a large shape database. Evaluation on a real-world dataset shows that our geometry-based re-ranking is a conceptually simple but highly effective method that can lead to a significant improvement in retrieval accuracy compared to the state-of-the-art.
更多查看译文
关键词
accurate instance-level CAD model retrieval,CAD neighbor-hood,clean CAD models,detailed object shape geometries,fine-grained retrieval,geometry-based re-ranking,instance level,large-scale database,moderately small database,object shape descriptor,retrieval accuracy,shape database,shape descriptors,top retrieval result
AI 理解论文
溯源树
样例
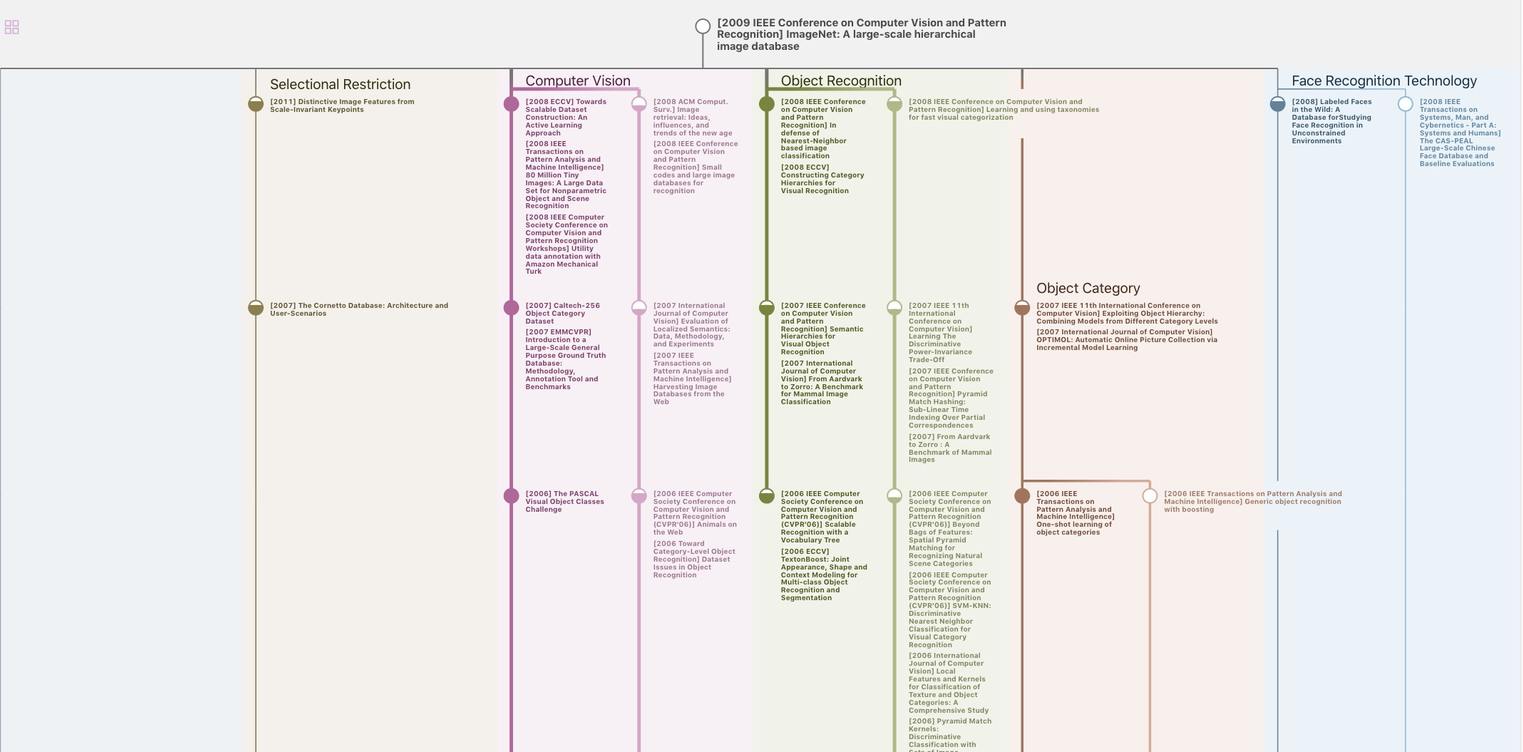
生成溯源树,研究论文发展脉络
Chat Paper
正在生成论文摘要