How Robust is Your Fairness? Evaluating and Sustaining Fairness under Unseen Distribution Shifts.
Transactions on machine learning research(2023)
摘要
Increasing concerns have been raised on deep learning fairness in recent years. Existing fairness-aware machine learning methods mainly focus on the fairness of in-distribution data. However, in real-world applications, it is common to have distribution shift between the training and test data. In this paper, we first show that the fairness achieved by existing methods can be easily broken by slight distribution shifts. To solve this problem, we propose a novel fairness learning method termed CUrvature MAtching (CUMA), which can achieve robust fairness generalizable to unseen domains with unknown distributional shifts. Specifically, CUMA enforces the model to have similar generalization ability on the majority and minority groups, by matching the loss curvature distributions of the two groups. We evaluate our method on three popular fairness datasets. Compared with existing methods, CUMA achieves superior fairness under unseen distribution shifts, without sacrificing either the overall accuracy or the in-distribution fairness.
更多查看译文
关键词
sustaining fairness,unseen distribution shifts,robust
AI 理解论文
溯源树
样例
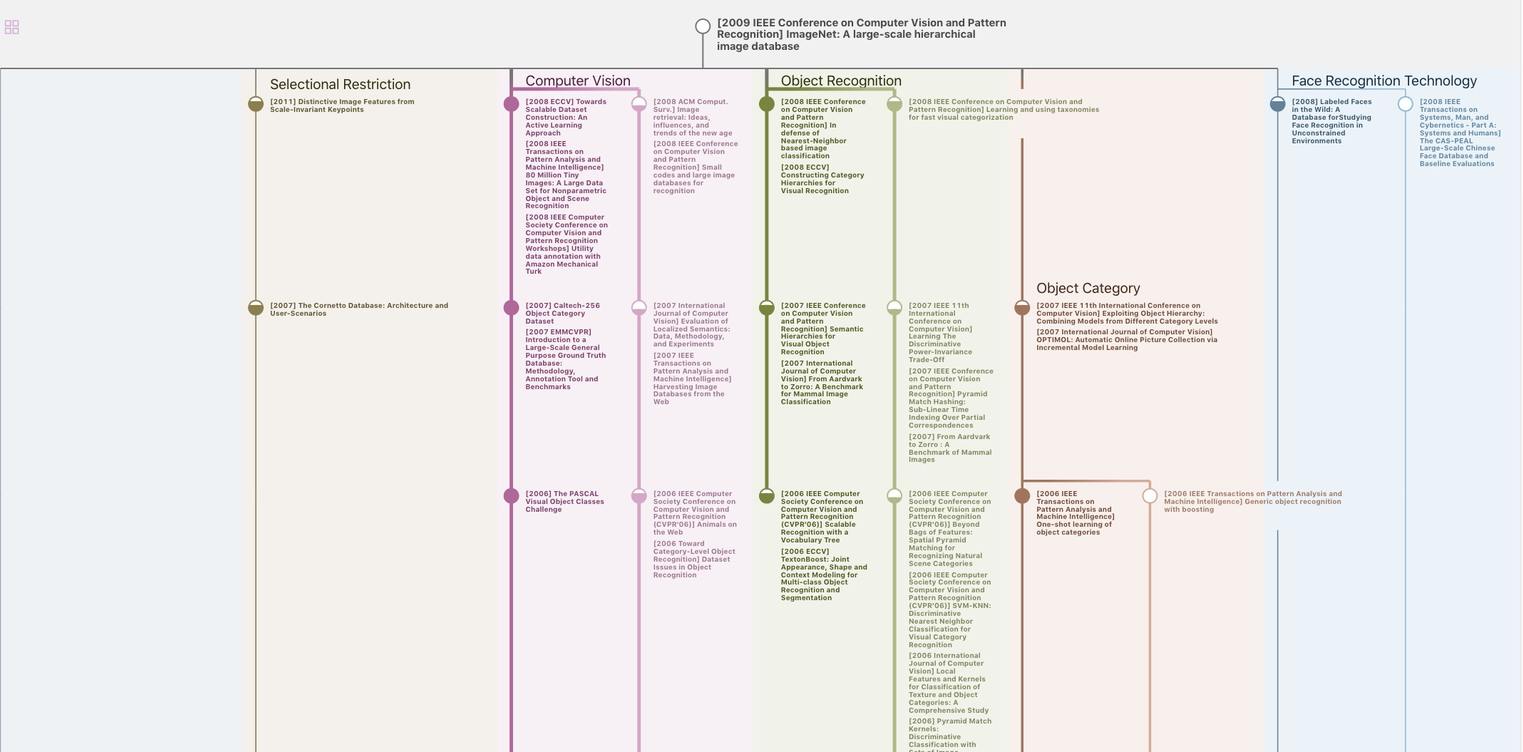
生成溯源树,研究论文发展脉络
Chat Paper
正在生成论文摘要