Agent with Tangent-Based Formulation and Anatomical Perception for Standard Plane Localization in 3D Ultrasound
MEDICAL IMAGE COMPUTING AND COMPUTER ASSISTED INTERVENTION, MICCAI 2022, PT IV(2022)
摘要
Standard plane (SP) localization is essential in routine clinical ultrasound (US) diagnosis. Compared to 2D US, 3D US can acquire multiple view planes in one scan and provide complete anatomy with the addition of coronal plane. However, manually navigating SPs in 3D US is laborious and biased due to the orientation variability and huge search space. In this study, we introduce a novel reinforcement learning (RL) framework for automatic SP localization in 3D US. Our contribution is three-fold. First, we formulate SP localization in 3D US as a tangent-point-based problem in RL to restructure the action space and significantly reduce the search space. Second, we design an auxiliary task learning strategy to enhance the model's ability to recognize subtle differences crossing Non-SPs and SPs in plane search. Finally, we propose a spatial-anatomical reward to effectively guide learning trajectories by exploiting spatial and anatomical information simultaneously. We explore the efficacy of our approach on localizing four SPs on uterus and fetal brain datasets. The experiments indicate that our approach achieves a high localization accuracy as well as robust performance.
更多查看译文
关键词
Reinforcement learning,Standard plane localization,Ultrasound
AI 理解论文
溯源树
样例
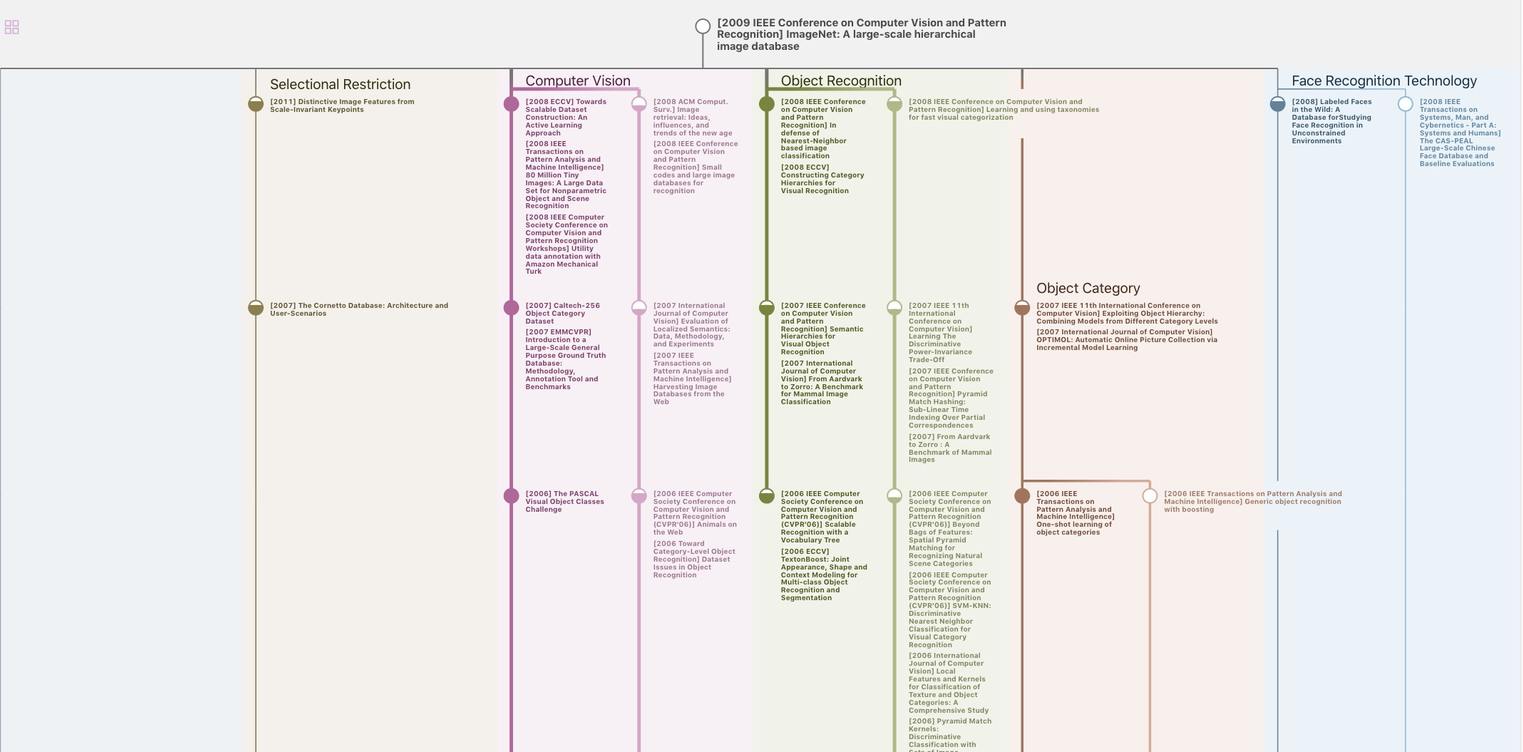
生成溯源树,研究论文发展脉络
Chat Paper
正在生成论文摘要