CEDAR: communication efficient distributed analysis for regressions.
Biometrics(2022)
摘要
Electronic health records (EHRs) offer great promises for advancing precision medicine and, at the same time, present significant analytical challenges. Particularly, it is often the case that patient-level data in EHRs cannot be shared across institutions (data sources) due to government regulations and/or institutional policies. As a result, there are growing interests about distributed learning over multiple EHRs databases without sharing patient-level data. To tackle such challenges, we propose a novel communication efficient method that aggregates the optimal estimates of external sites, by turning the problem into a missing data problem. In addition, we propose incorporating posterior samples of remote sites, which can provide partial information on the missing quantities and improve efficiency of parameter estimates while having the differential privacy property and thus reducing the risk of information leaking. The proposed approach, without sharing the raw patient level data, allows for proper statistical inference. We provide theoretical investigation for the asymptotic properties of the proposed method for statistical inference as well as differential privacy, and evaluate its performance in simulations and real data analyses in comparison with several recently developed methods.
更多查看译文
关键词
communication efficient,differential privacy,distributed learning,distributed statistical inference
AI 理解论文
溯源树
样例
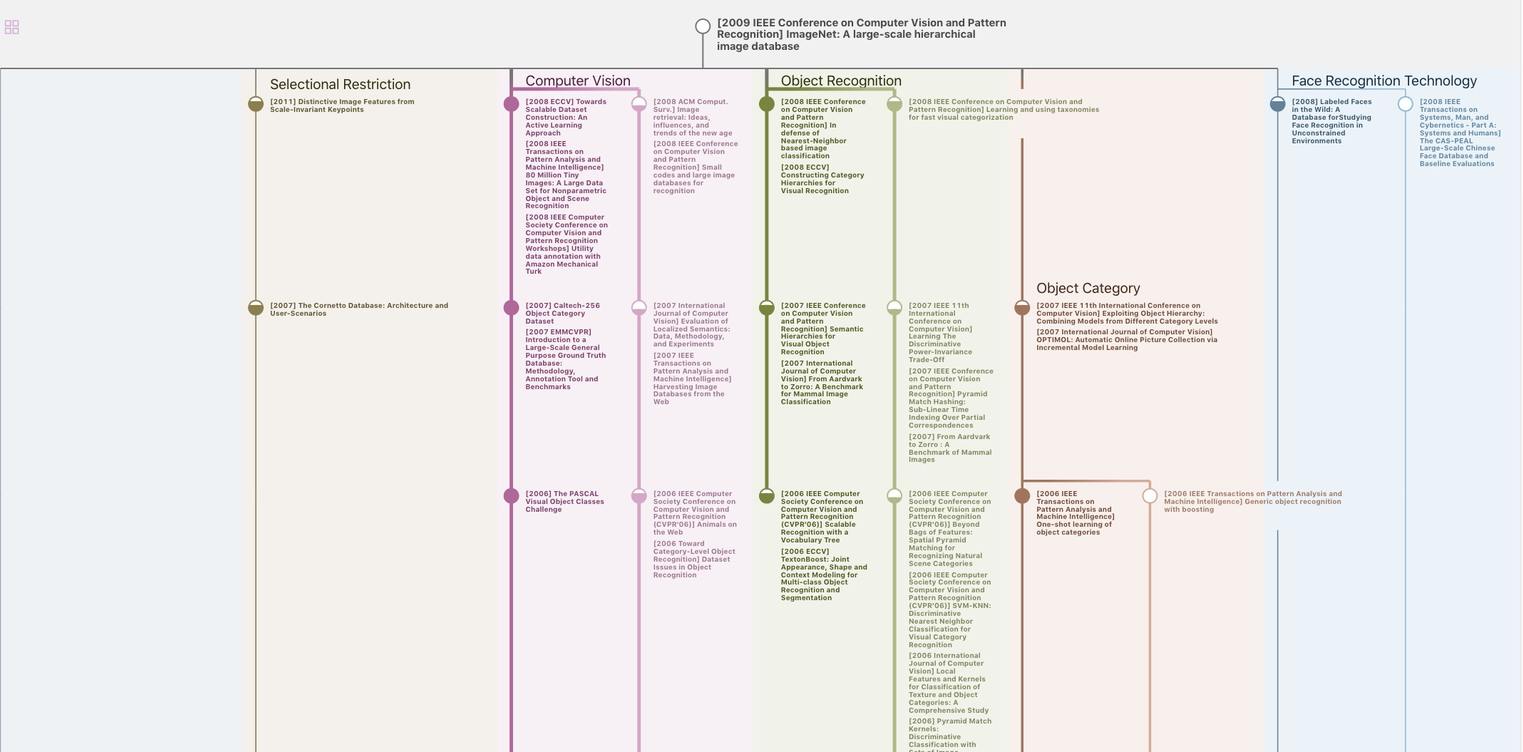
生成溯源树,研究论文发展脉络
Chat Paper
正在生成论文摘要