Generating transient noise artefacts in gravitational-wave detector data with generative adversarial networks
arxiv(2023)
摘要
Transient noise glitches in gravitational-wave detector data limit the sensitivity of searches and contaminate detected signals. In this paper, we show how glitches can be simulated using generative adversarial networks (GANs). We produce hundreds of synthetic images for the 22 most common types of glitches seen in the LIGO, KAGRA, and Virgo detectors. We show how our GAN-generated images can easily be converted to time series, which would allow us to use GAN-generated glitches in simulations and mock data challenges to improve the robustness of gravitational-wave searches and parameter-estimation algorithms. We perform a neural network classification to show that our artificial glitches are an excellent match for real glitches, with an average classification accuracy across all 22 glitch types of 99.0%.
更多查看译文
关键词
gravitational waves,detector characterisation,machine learning
AI 理解论文
溯源树
样例
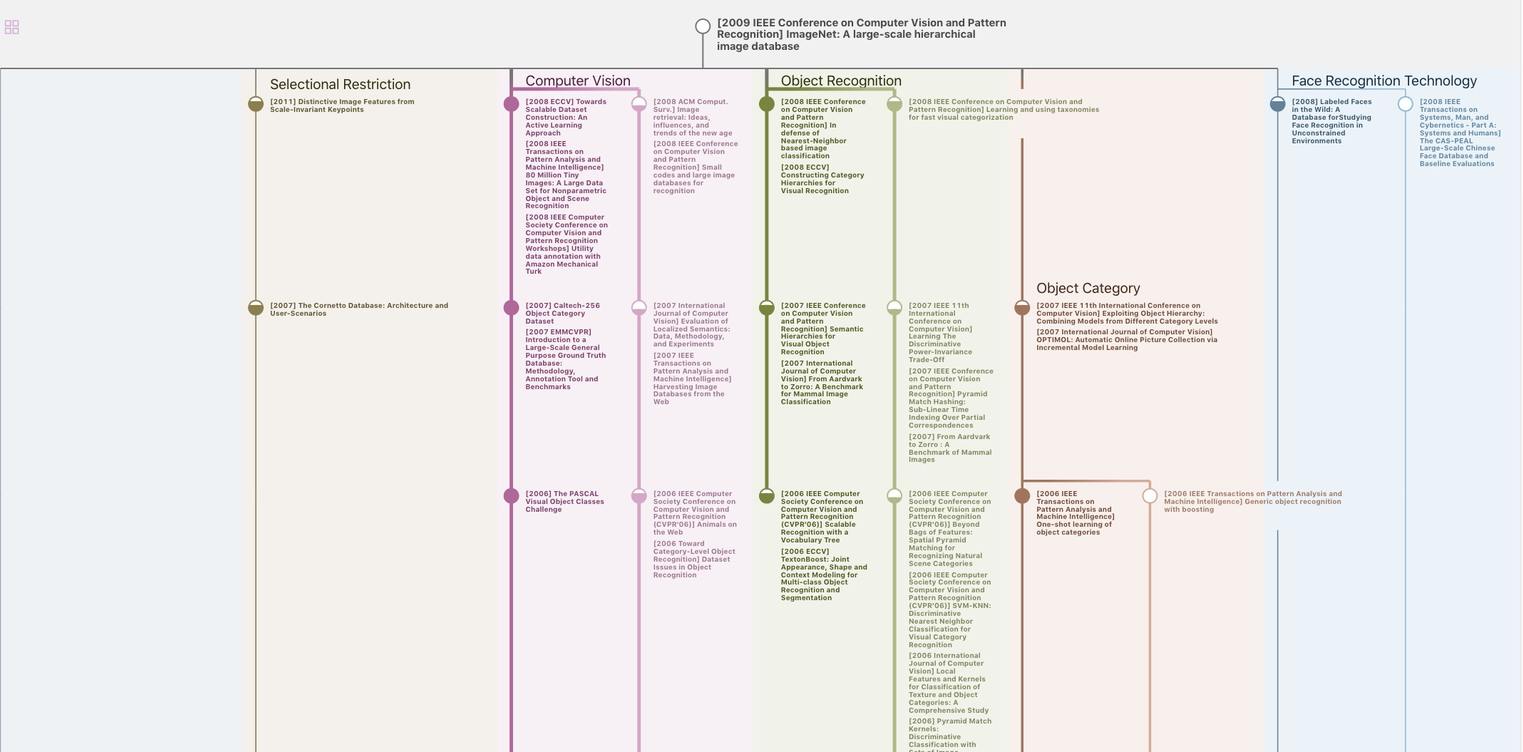
生成溯源树,研究论文发展脉络
Chat Paper
正在生成论文摘要