Robustness of Epinets against Distributional Shifts
arxiv(2022)
摘要
Recent work introduced the epinet as a new approach to uncertainty modeling in deep learning. An epinet is a small neural network added to traditional neural networks, which, together, can produce predictive distributions. In particular, using an epinet can greatly improve the quality of joint predictions across multiple inputs, a measure of how well a neural network knows what it does not know. In this paper, we examine whether epinets can offer similar advantages under distributional shifts. We find that, across ImageNet-A/O/C, epinets generally improve robustness metrics. Moreover, these improvements are more significant than those afforded by even very large ensembles at orders of magnitude lower computational costs. However, these improvements are relatively small compared to the outstanding issues in distributionally-robust deep learning. Epinets may be a useful tool in the toolbox, but they are far from the complete solution.
更多查看译文
关键词
epinets,shifts,robustness
AI 理解论文
溯源树
样例
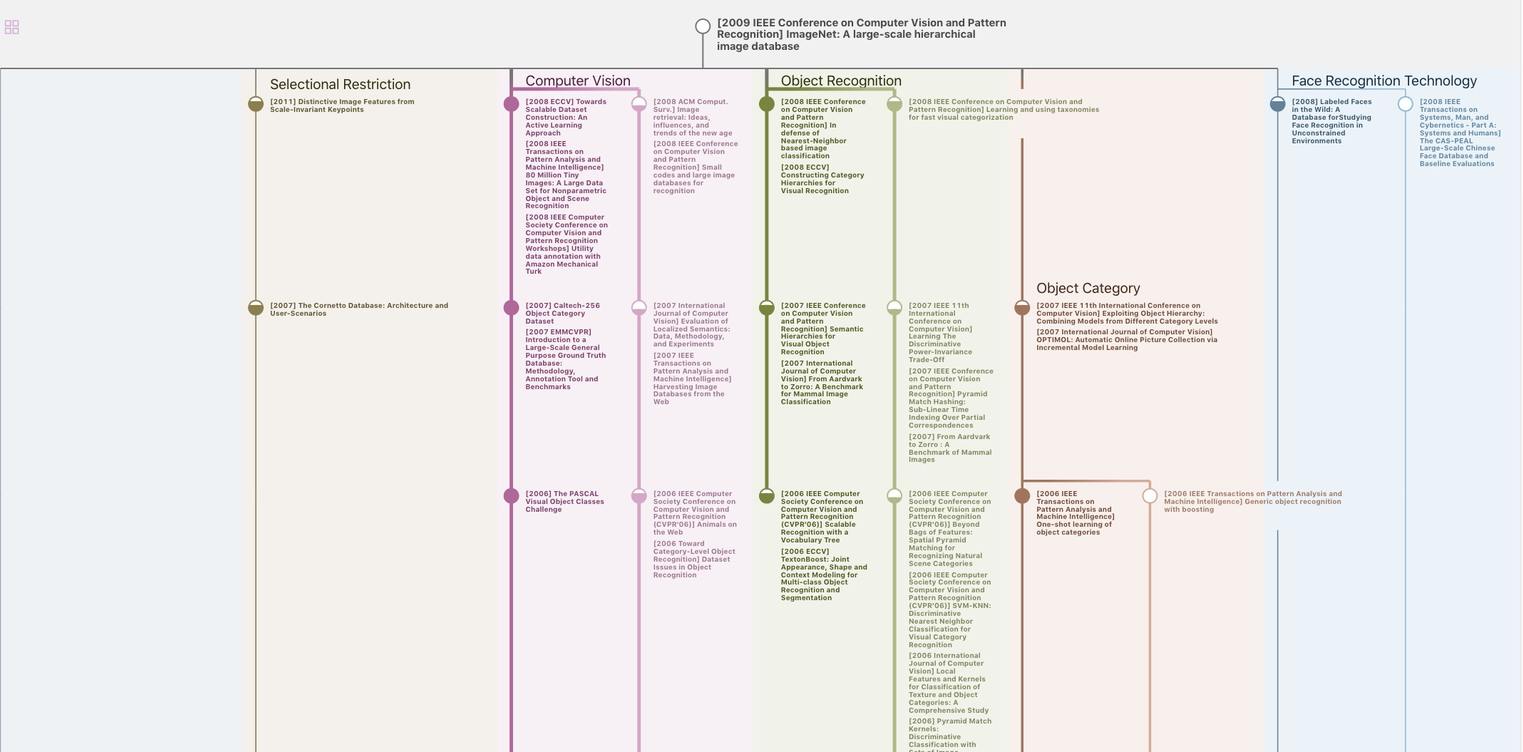
生成溯源树,研究论文发展脉络
Chat Paper
正在生成论文摘要