Advances in Prediction of Readmission Rates Using Long Term Short Term Memory Networks on Healthcare Insurance Data
arxiv(2022)
摘要
30-day hospital readmission is a long standing medical problem that affects patients' morbidity and mortality and costs billions of dollars annually. Recently, machine learning models have been created to predict risk of inpatient readmission for patients with specific diseases, however no model exists to predict this risk across all patients. We developed a bi-directional Long Short Term Memory (LSTM) Network that is able to use readily available insurance data (inpatient visits, outpatient visits, and drug prescriptions) to predict 30 day re-admission for any admitted patient, regardless of reason. The top-performing model achieved an ROC AUC of 0.763 (0.011) when using historical, inpatient, and post-discharge data. The LSTM model significantly outperformed a baseline random forest classifier, indicating that understanding the sequence of events is important for model prediction. Incorporation of 30-days of historical data also significantly improved model performance compared to inpatient data alone, indicating that a patients clinical history prior to admission, including outpatient visits and pharmacy data is a strong contributor to readmission. Our results demonstrate that a machine learning model is able to predict risk of inpatient readmission with reasonable accuracy for all patients using structured insurance billing data. Because billing data or equivalent surrogates can be extracted from sites, such a model could be deployed to identify patients at risk for readmission before they are discharged, or to assign more robust follow up (closer follow up, home health, mailed medications) to at-risk patients after discharge.
更多查看译文
关键词
readmission rates,memory networks,term memory,long term short term,healthcare
AI 理解论文
溯源树
样例
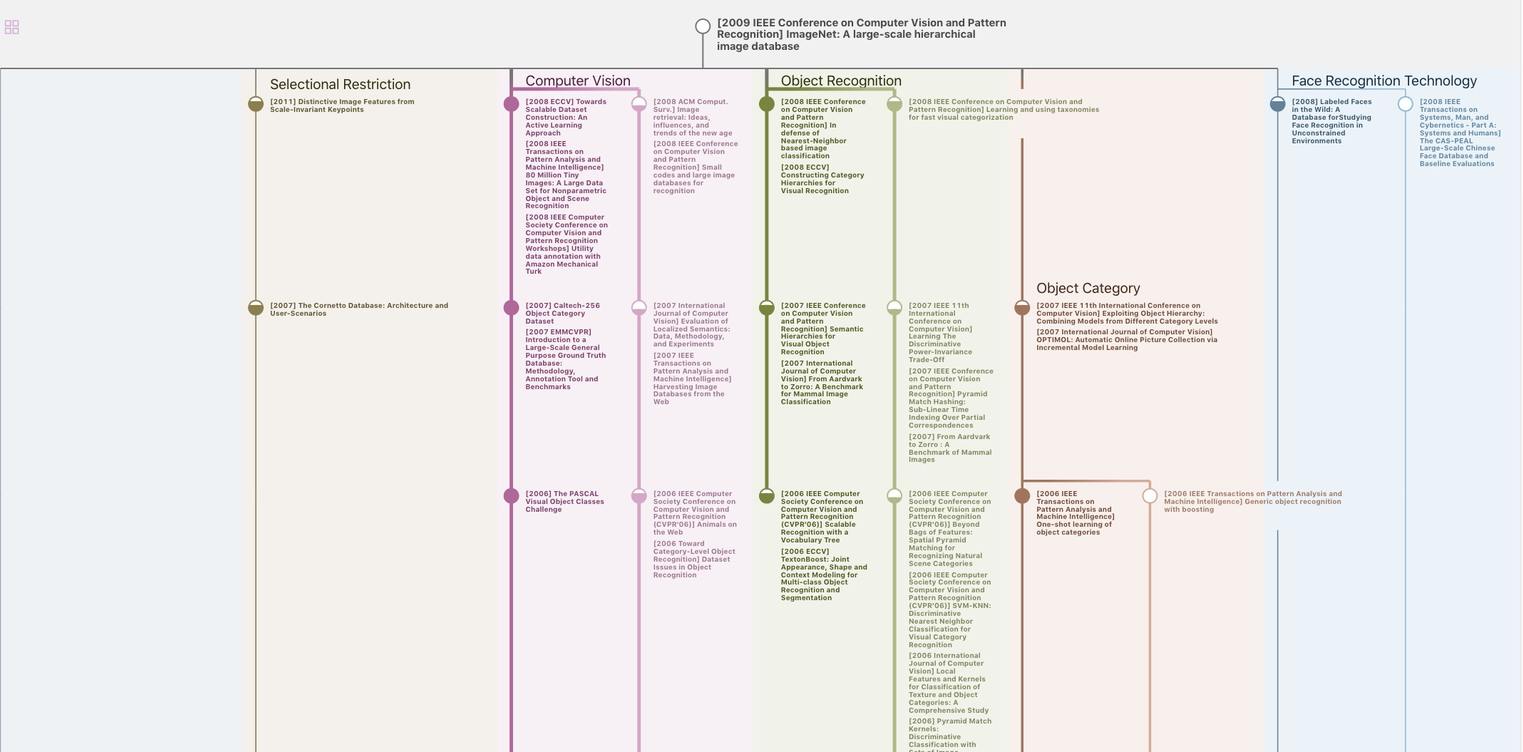
生成溯源树,研究论文发展脉络
Chat Paper
正在生成论文摘要