Self-Supervised Dense Consistency Regularization for Image-to-Image Translation
IEEE Conference on Computer Vision and Pattern Recognition(2022)
摘要
Unsupervised image-to-image translation has gained considerable attention due to recent impressive advances in generative adversarial networks (GANs). This paper presents a simple but effective regularization technique for improving GAN-based image-to-image translation. To generate images with realistic local semantics and structures, we propose an auxiliary self-supervision loss that enforces point-wise consistency of the overlapping region between a pair of patches cropped from a single real image during training the discriminator of a GAN. Our experiment shows that the proposed dense consistency regularization improves performance substantially on various image-to-image translation scenarios. It also leads to extra performance gains through the combination with instance-level regularization methods. Furthermore, we verify that the proposed model captures domain-specific characteristics more effectively with only a small fraction of training data.
更多查看译文
关键词
Image and video synthesis and generation, Computer vision theory, Deep learning architectures and techniques, Machine learning, Representation learning
AI 理解论文
溯源树
样例
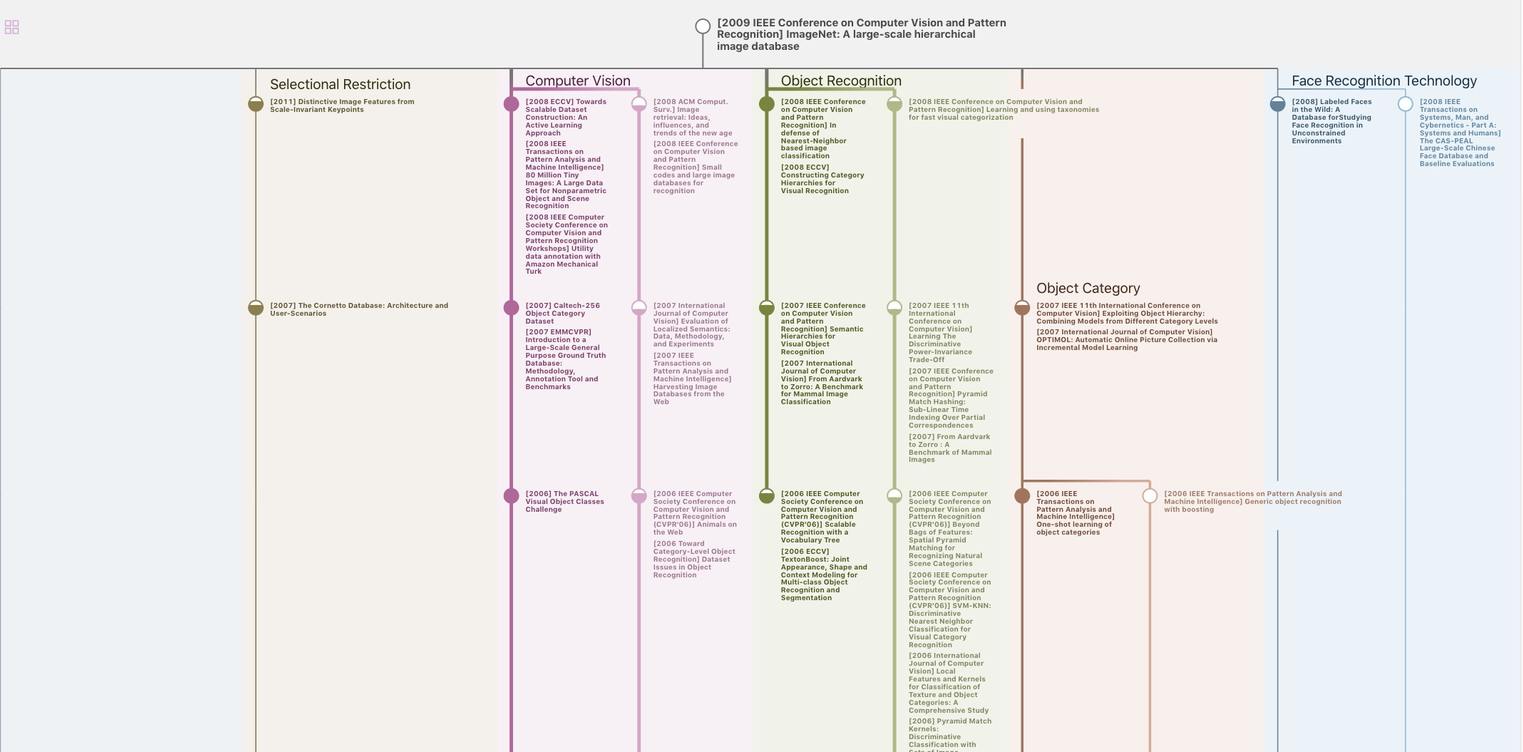
生成溯源树,研究论文发展脉络
Chat Paper
正在生成论文摘要