Self-Supervised Global-Local Structure Modeling for Point Cloud Domain Adaptation with Reliable Voted Pseudo Labels
IEEE Conference on Computer Vision and Pattern Recognition(2022)
Abstract
In this paper, we propose an unsupervised domain adaptation method for deep point cloud representation learning. To model the internal structures in target point clouds, we first propose to learn the global representations of unla-beled data by scaling up or down point clouds and then predicting the scales. Second, to capture the local structure in a self-supervised manner, we propose to project a 3D local area onto a 2D plane and then learn to reconstruct the squeezed region. Moreover, to effectively transfer the knowledge from source domain, we propose to vote pseudo labels for target samples based on the labels of their nearest source neighbors in the shared feature space. To avoid the noise caused by incorrect pseudo labels, we only select re-liable target samples, whose voting consistencies are high enough, for enhancing adaptation. The voting method is able to adaptively select more and more target samples during training, which in return facilitates adaptation because the amount of labeled target data increases. Experiments on PointDA (ModelNet-10, ShapeNet-10 and ScanNet-10) and Sim-to-Real (ModelNet-11, ScanObjectNN-11, ShapeNet-9 and ScanObjectNN-9) demonstrate the effectiveness of our method.
MoreTranslated text
Key words
3D from multi-view and sensors, 3D from single images, Recognition: detection,categorization,retrieval, Representation learning, RGBD sensors and analytics, Transfer/low-shot/long-tail learning
AI Read Science
Must-Reading Tree
Example
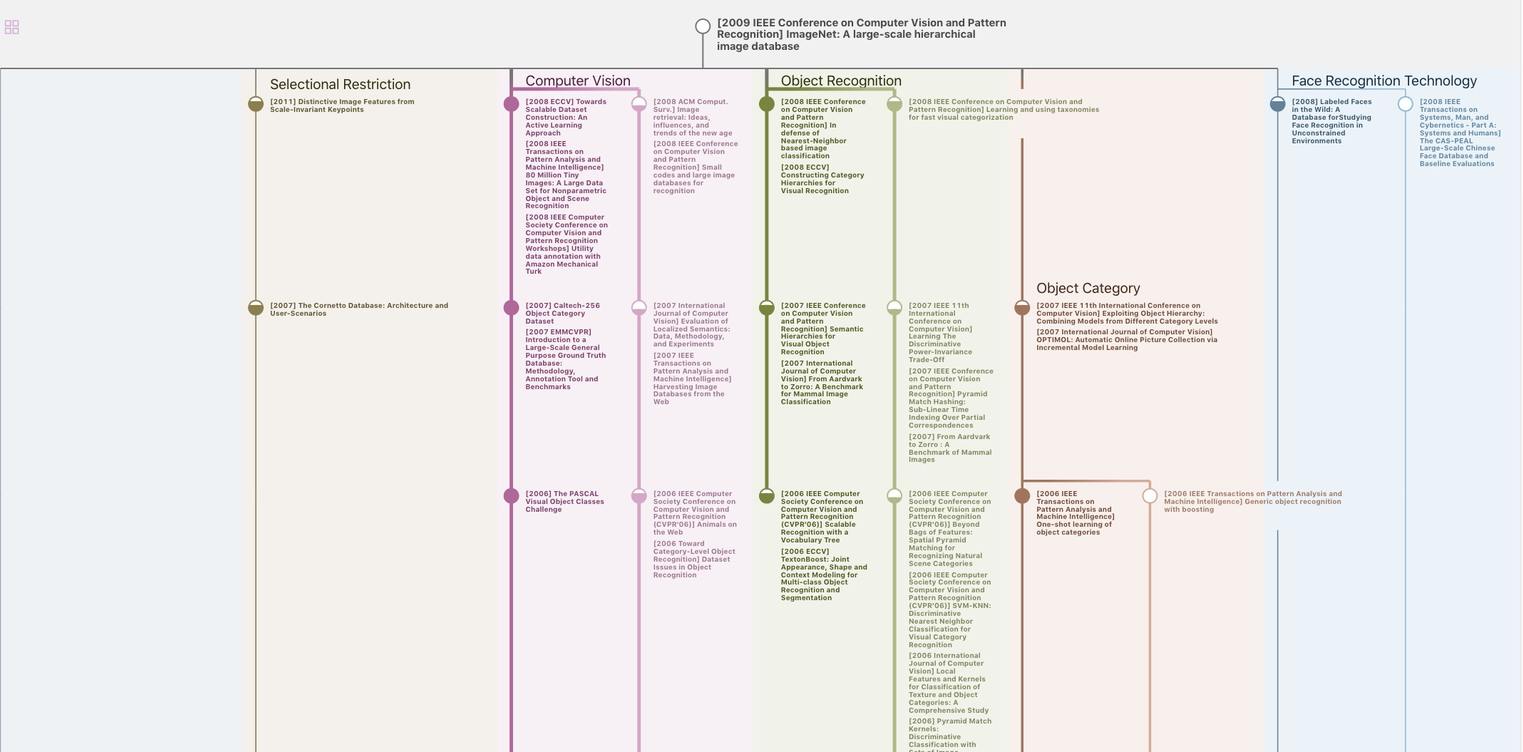
Generate MRT to find the research sequence of this paper
Chat Paper
Summary is being generated by the instructions you defined