MPC: Multi-view Probabilistic Clustering
IEEE Conference on Computer Vision and Pattern Recognition(2022)
摘要
Despite the promising progress having been made, the two challenges of multi-view clustering (MVC) are still waiting for better solutions: i) Most existing methods are either not qualified or require additional steps for incomplete multi-view clustering and ii) noise or outliers might significantly degrade the overall clustering performance. In this paper, we propose a novel unified framework for incomplete and complete MVC named multi-view probabilistic clustering (MPC). MPC equivalently transforms multi-view pairwise posterior matching probability into composition of each view's individual distribution, which tolerates data missing and might extend to any number of views. Then graph-context-aware refinement with path propagation and co-neighbor propagation is used to refine pairwise probability, which alleviates the impact of noise and outliers. Finally, MPC also equivalently transforms probabilistic clustering's objective to avoid complete pairwise computation and adjusts clustering assignments by maximizing joint probability iteratively. Extensive experiments on multiple benchmarks for incomplete and complete MVC show that MPC significantly outperforms previous state-of-the-art methods in both effectiveness and efficiency.
更多查看译文
关键词
Recognition: detection,categorization,retrieval
AI 理解论文
溯源树
样例
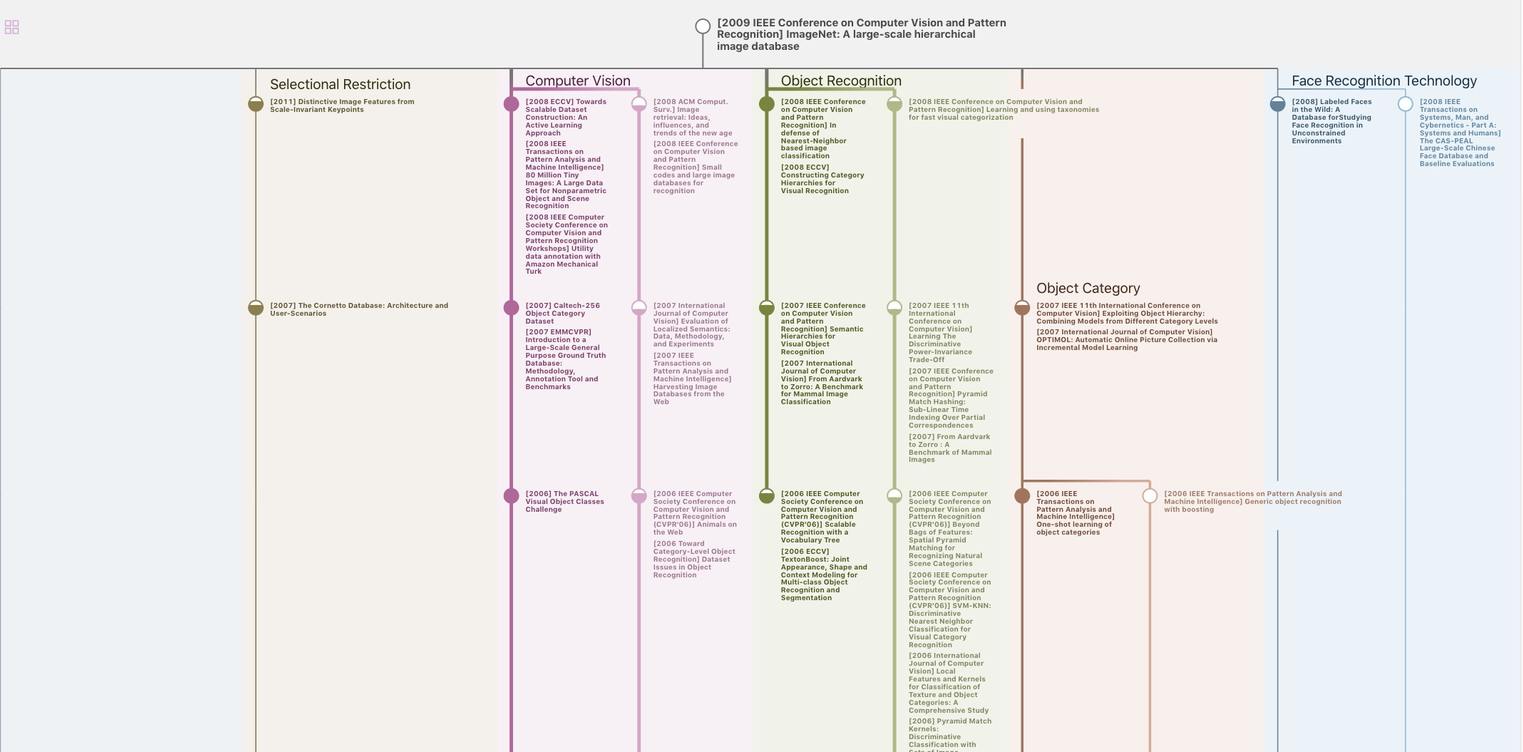
生成溯源树,研究论文发展脉络
Chat Paper
正在生成论文摘要