AME: Attention and Memory Enhancement in Hyper-Parameter Optimization
2022 IEEE/CVF Conference on Computer Vision and Pattern Recognition (CVPR)(2022)
摘要
Training Deep Neural Networks (DNNs) is inherently subject to sensitive hyper-parameters and untimely feedbacks of performance evaluation. To solve these two difficulties, an efficient parallel hyper-parameter optimization model is proposed under the framework of Deep Reinforcement Learning (DRL). Technically, we develop Attention and Memory Enhancement (AME), that includes multi-head attention and memory mechanism to enhance the ability to capture both the short-term and long-term relationships between different hyper-parameter configurations, yielding an attentive sampling mechanism for searching high-performance configurations embedded into a huge search space. During the optimization of transformer-structured configuration searcher, a conceptually intuitive yet powerful strategy is applied to solve the problem of insufficient number of samples due to the untimely feedback. Experiments on three visual tasks, including image classification, object detection, semantic segmentation, demonstrate the effectiveness of AME.
更多查看译文
关键词
Optimization methods, Recognition: detection,categorization,retrieval, Segmentation,grouping and shape analysis
AI 理解论文
溯源树
样例
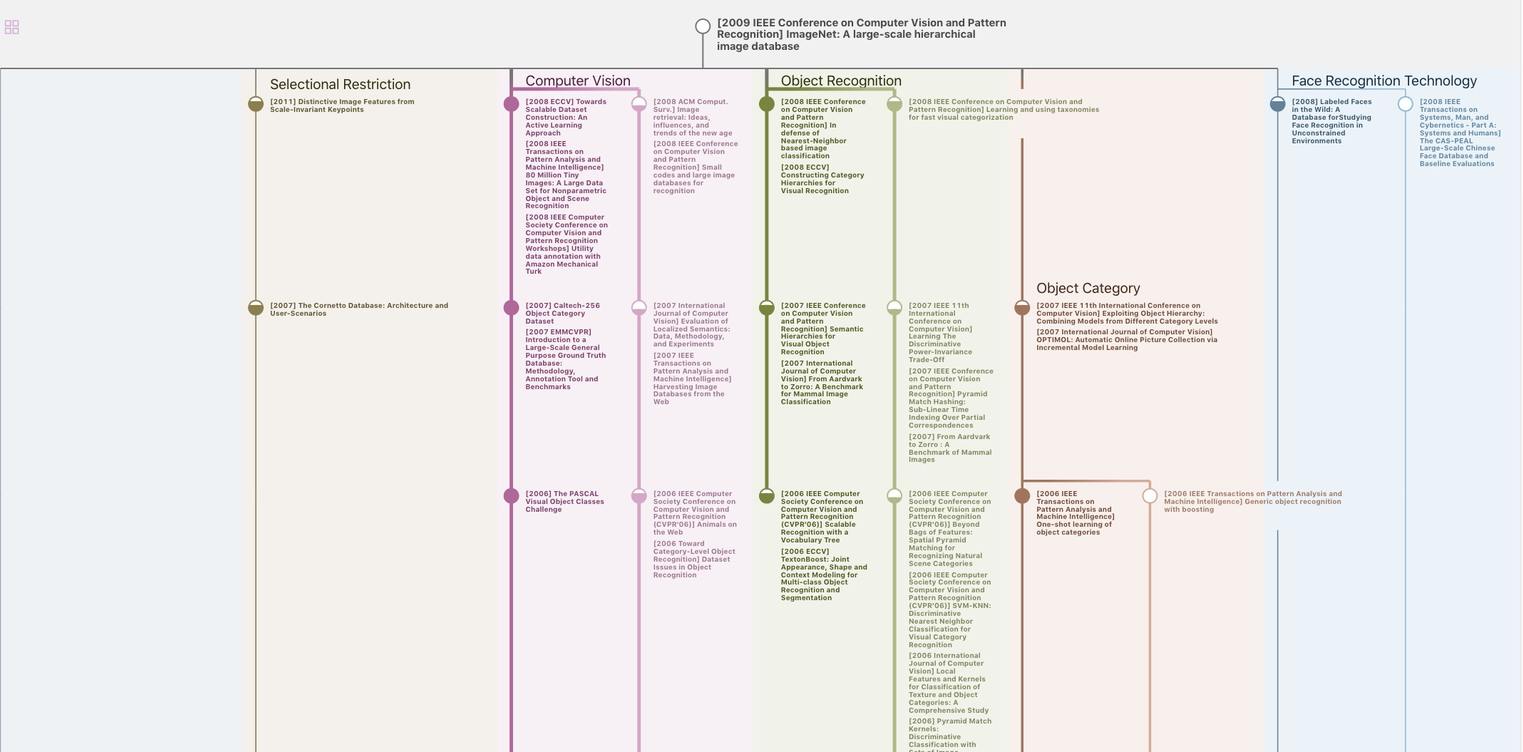
生成溯源树,研究论文发展脉络
Chat Paper
正在生成论文摘要