Think Twice Before Detecting GAN-generated Fake Images from their Spectral Domain Imprints
IEEE Conference on Computer Vision and Pattern Recognition(2022)
摘要
Accurate detection of the fake but photorealistic images is one of the most challenging tasks to address social, biometrics security and privacy related concerns in our community. Earlier research has underlined the existence of spectral domain artifacts in fake images generated by powerful generative adversarial network (GAN) based methods. Therefore, a number of highly accurate frequency domain methods to detect such GAN generated images have been proposed in the literature. Our study in this paper introduces a pipeline to mitigate the spectral artifacts. We show from our experiments that the artifacts in frequency spectrum of such fake images can be mitigated by proposed methods, which leads to the sharp decrease of performance of spectrum-based detectors. This paper also presents experimental results using a large database of images that are synthesized using BigGAN, CRN, CycleGAN, IMLE, Pro-GAN, StarGAN, StyleGAN and StyleGAN2 (including synthesized high resolution fingerprint images) to illustrate effectiveness of the proposed methods. Furthermore, we select a spatial-domain based fake image detector and observe a notable decrease in the detection performance when proposed method is incorporated. In summary, our insightful analysis and pipeline presented in this paper cautions the forensic community on the reliability of GAN-generated fake image detectors that are based on the analysis of frequency artifacts as these artifacts can be easily mitigated.
更多查看译文
关键词
Image and video synthesis and generation, Computer vision for social good, Vision applications and systems
AI 理解论文
溯源树
样例
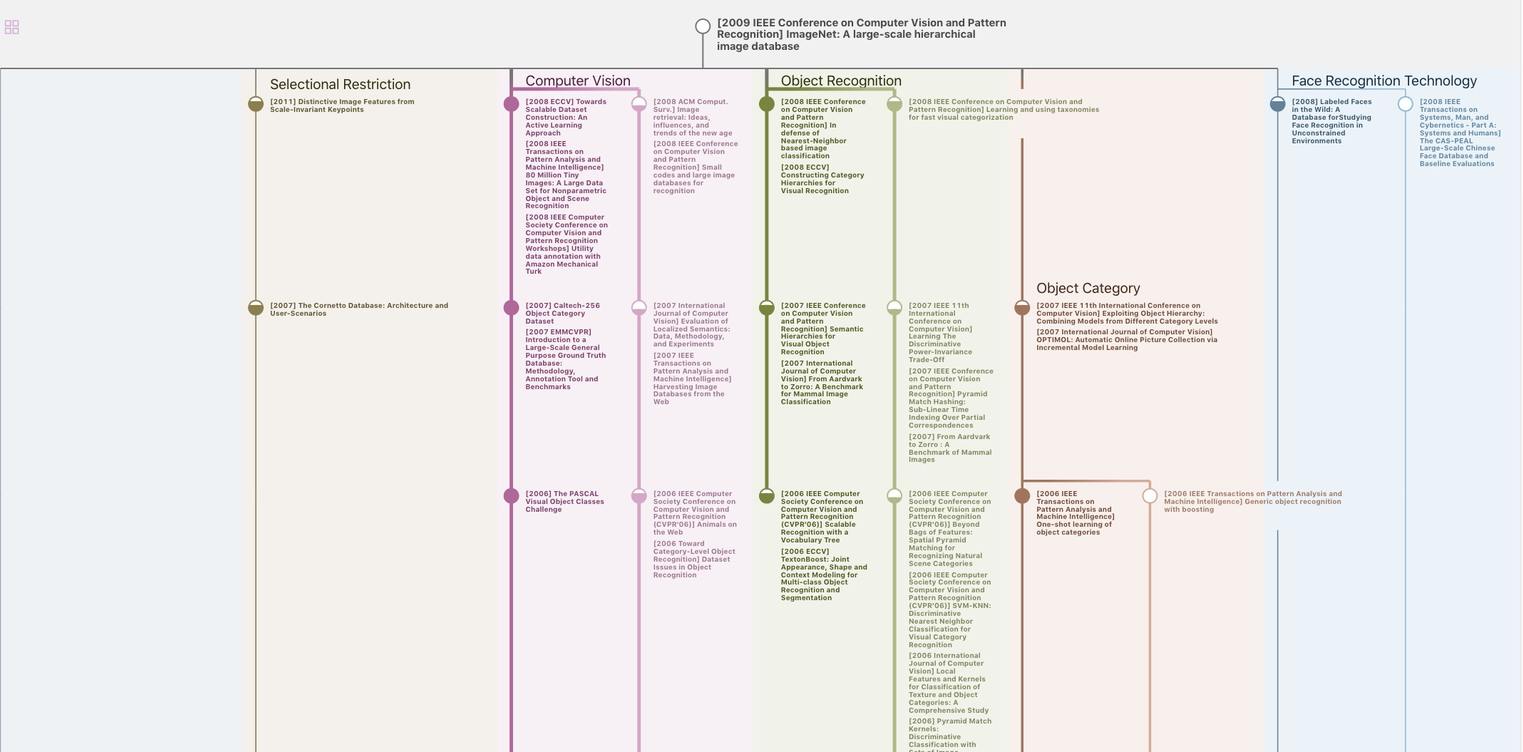
生成溯源树,研究论文发展脉络
Chat Paper
正在生成论文摘要