Reversible Vision Transformers
IEEE Conference on Computer Vision and Pattern Recognition(2022)
摘要
We present Reversible Vision Transformers, a memory efficient architecture design for visual recognition. By decoupling the GPU memory footprint from the depth of the model, Reversible Vision Transformers enable memory efficient scaling of transformer architectures. We adapt two popular models, namely Vision Transformer and Multiscale Vision Transformers, to reversible variants and benchmark extensively across both model sizes and tasks of image classification, object detection and video classification. Reversible Vision Transformers achieve a reduced memory footprint of up to 15.5× at identical model complexity, parameters and accuracy, demonstrating the promise of reversible vision transformers as an efficient backbone for resource limited training regimes. Finally, we find that the additional computational burden of recomputing activations is more than overcome for deeper models, where throughput can increase up to 3.9 × over their non-reversible counterparts. Code and models are available at https://github.com/facebookresearch/mvit.
更多查看译文
关键词
Deep learning architectures and techniques, Machine learning, Recognition: detection,categorization,retrieval, Representation learning, Video analysis and understanding
AI 理解论文
溯源树
样例
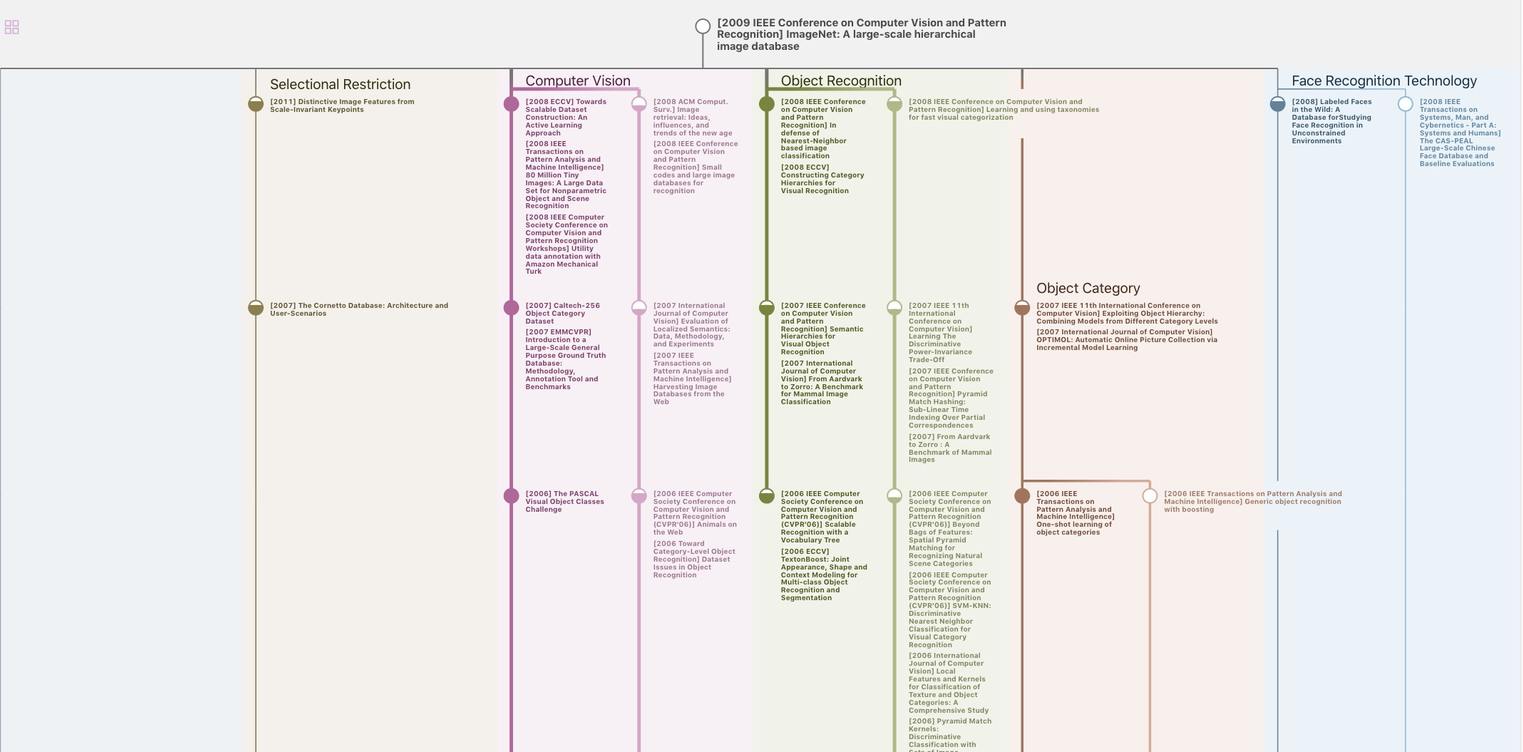
生成溯源树,研究论文发展脉络
Chat Paper
正在生成论文摘要