Source-Free Object Detection by Learning to Overlook Domain Style
IEEE Conference on Computer Vision and Pattern Recognition(2022)
Abstract
Source-free object detection (SFOD) needs to adapt a detector pre-trained on a labeled source domain to a tar-get domain, with only unlabeled training data from the tar-get domain. Existing SFOD methods typically adopt the pseudo labeling paradigm with model adaption alternating between predicting pseudo labels and fine-tuning the model. This approach suffers from both unsatisfactory accuracy of pseudo labels due to the presence of domain shift and lim-ited use of target domain training data. In this work, we present a novel Learning to Overlook Domain Style (LODS) method with such limitations solved in a principled man-ner. Our idea is to reduce the domain shift effect by en-forcing the model to overlook the target domain style, such that model adaptation is simplified and becomes easier to carry on. To that end, we enhance the style of each tar-get domain image and leverage the style degree difference between the original image and the enhanced image as a self-supervised signal for model adaptation. By treating the enhanced image as an auxiliary view, we exploit a student- teacher architecture for learning to overlook the style de-gree difference against the original image, also character-ized with a novel style enhancement algorithm and graph alignment constraint. Extensive experiments demonstrate that our LODS yields new state-of-the-art performance on four benchmarks.
MoreTranslated text
Key words
Transfer/low-shot/long-tail learning, Recognition: detection,categorization,retrieval, Self-& semi-& meta- & unsupervised learning
AI Read Science
Must-Reading Tree
Example
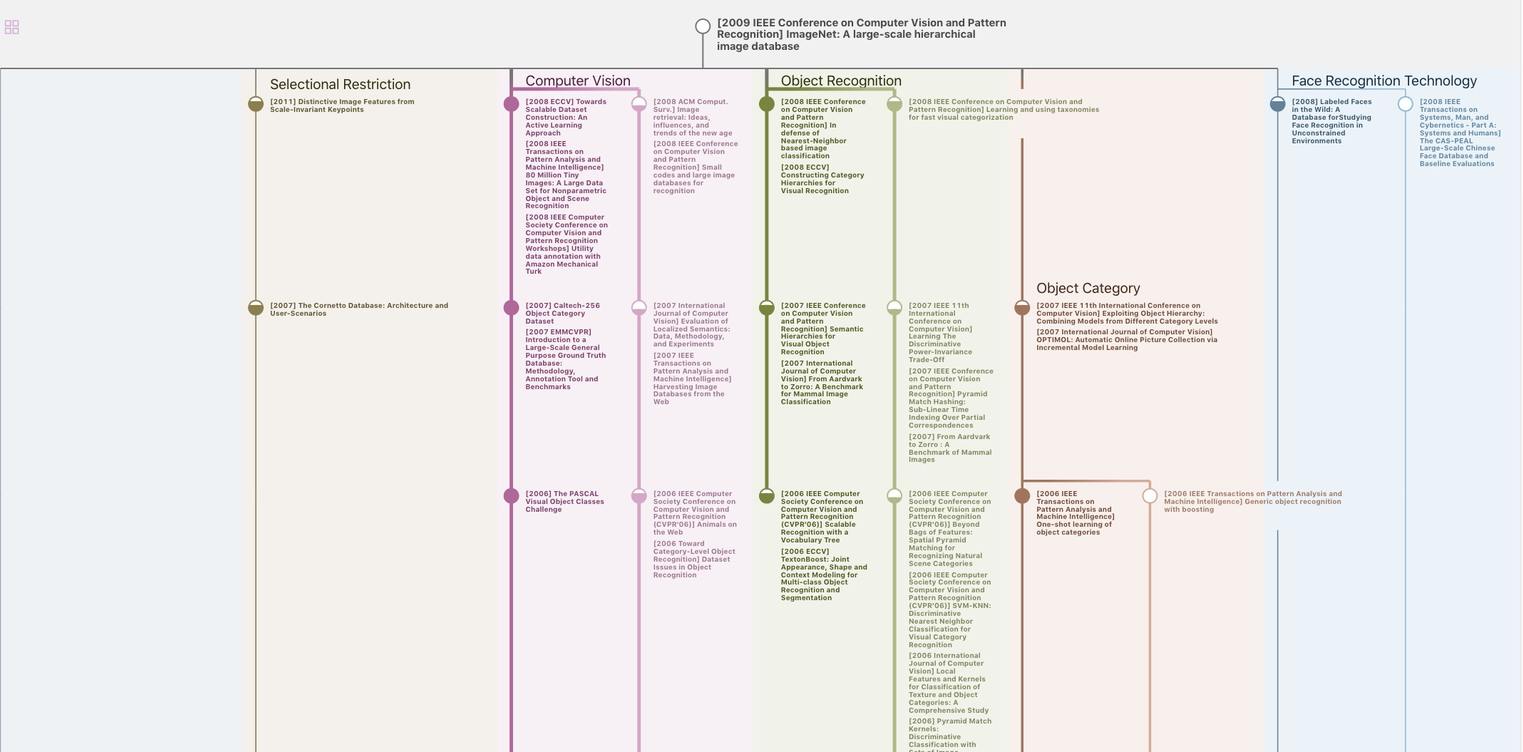
Generate MRT to find the research sequence of this paper
Chat Paper
Summary is being generated by the instructions you defined