Convergence Rates of Non-Convex Stochastic Gradient Descent Under a Generic Lojasiewicz Condition and Local Smoothness.
International Conference on Machine Learning(2022)
摘要
Training over-parameterized neural networks involves the empirical minimization of highly non-convex objective functions. Recently, a large body of works provided theoretical evidence that, despite this non-convexity, properly initialized over-parameterized networks can converge to a zero training loss through the introduction of the Polyak-Lojasiewicz condition. However, these analyses are restricted to quadratic losses such as mean square error, and tend to indicate fast exponential convergence rates that are seldom observed in practice. In this work, we propose to extend these results by analyzing stochastic gradient descent under more generic Lojasiewicz conditions that are applicable to any convex loss function, thus extending the current theory to a larger panel of losses commonly used in practice such as cross-entropy. Moreover, our analysis provides high-probability bounds on the approximation error under sub-Gaussian gradient noise and only requires the local smoothness of the objective function, thus making it applicable to deep neural networks in realistic settings.
更多查看译文
关键词
generic łojasiewicz condition,local smoothness,stochastic,non-convex
AI 理解论文
溯源树
样例
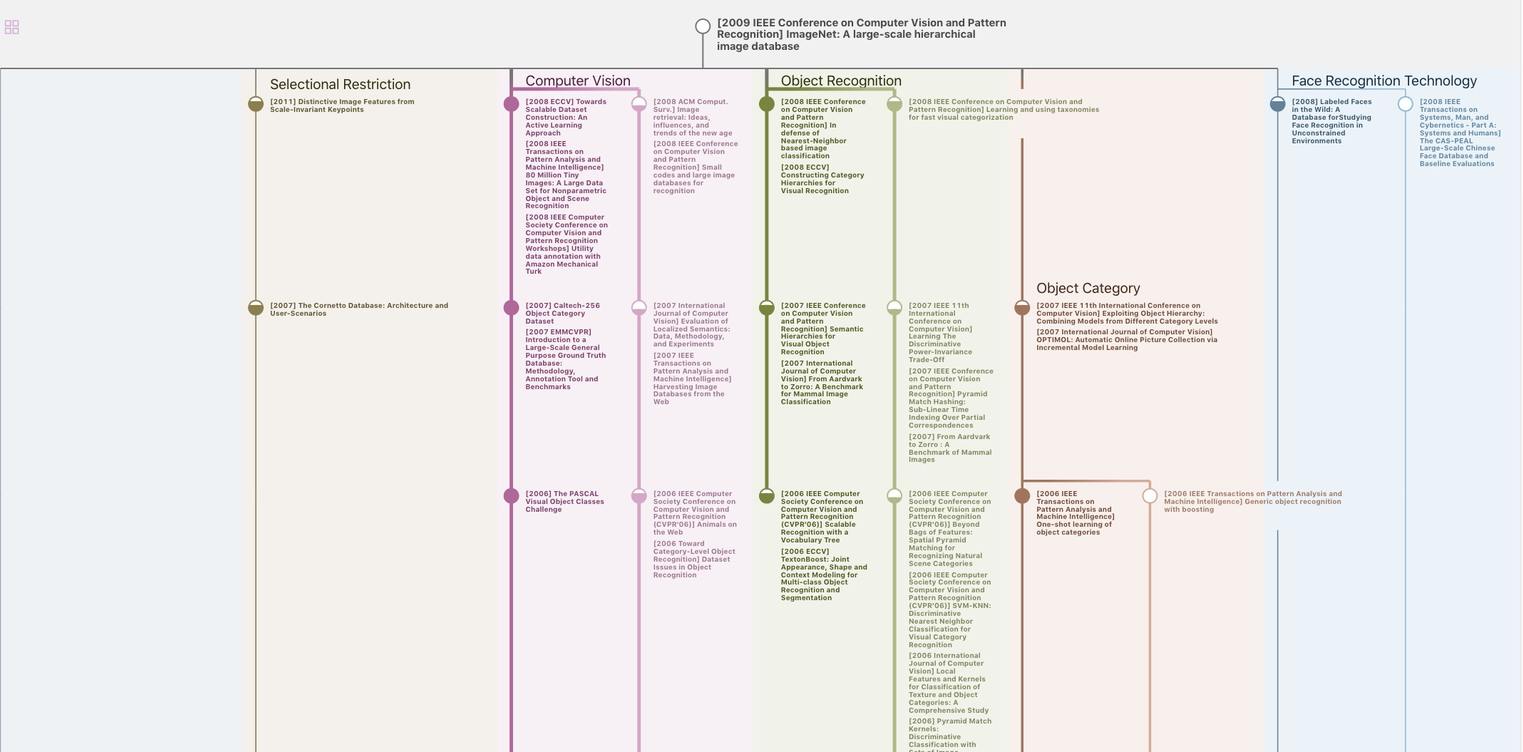
生成溯源树,研究论文发展脉络
Chat Paper
正在生成论文摘要