Consistent Polyhedral Surrogates for Top-k Classification and Variants.
International Conference on Machine Learning(2022)
Abstract
Top-$k$ classification is a generalization of multiclass classification used widely in information retrieval, image classification, and other extreme classification settings. Several hinge-like (piecewise-linear) surrogates have been proposed for the problem, yet all are either non-convex or inconsistent. For the proposed hinge-like surrogates that are convex (i.e., polyhedral), we apply the recent embedding framework of Finocchiaro et al. (2019; 2022) to determine the prediction problem for which the surrogate is consistent. These problems can all be interpreted as variants of top-$k$ classification, which may be better aligned with some applications. We leverage this analysis to derive constraints on the conditional label distributions under which these proposed surrogates become consistent for top-$k$. It has been further suggested that every convex hinge-like surrogate must be inconsistent for top-$k$. Yet, we use the same embedding framework to give the first consistent polyhedral surrogate for this problem.
MoreTranslated text
Key words
consistent polyhedral surrogates,variants,classification
AI Read Science
Must-Reading Tree
Example
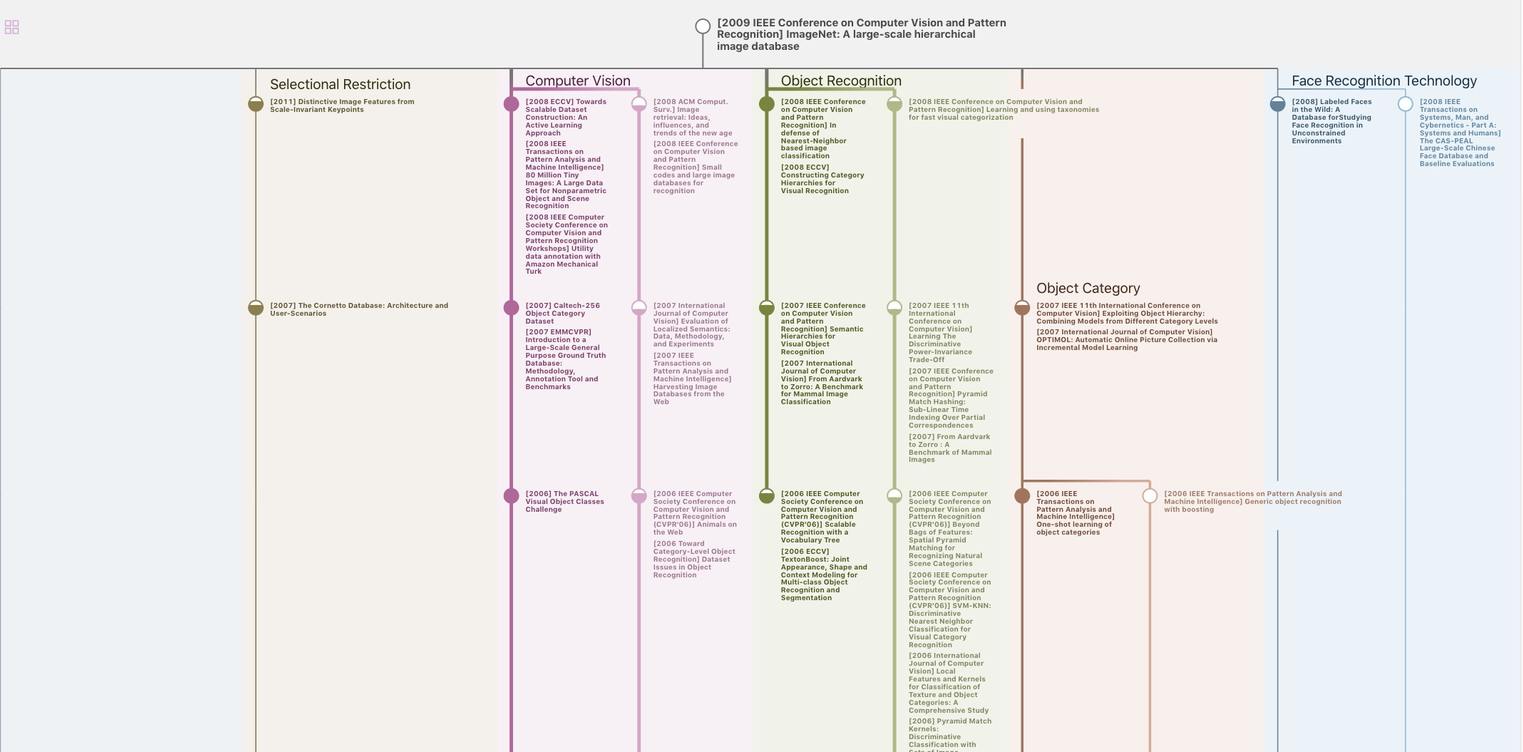
Generate MRT to find the research sequence of this paper
Chat Paper
Summary is being generated by the instructions you defined