Cytokine TGF beta Gene Polymorphism in Asthma: TGF-Related SNP Analysis Enhances the Prediction of Disease Diagnosis (A Case-Control Study With Multivariable Data-Mining Model Development)
FRONTIERS IN IMMUNOLOGY(2022)
Abstract
Introduction: TGF-beta and its receptors play a crucial role in asthma pathogenesis and bronchial remodeling in the course of the disease. TGF-beta 1, TGF-beta 2, and TGF-beta 3 isoforms are responsible for chronic inflammation, bronchial hyperreactivity, myofibroblast activation, fibrosis, bronchial remodeling, and change the expression of approximately 1000 genes in asthma. TGF-beta SNPs are associated with the elevated plasma level of TGF-beta 1, an increased level of total IgE, and an increased risk of remodeling of bronchi. Methods: The analysis of selected TGF-beta 1, TGF-beta 2, TGF-beta 3-related single-nucleotide polymorphisms (SNP) was conducted on 652 DNA samples with an application of the MassARRAY((R)) using the mass spectrometry (MALDI-TOF MS). Dataset was randomly split into training (80%) and validation sets (20%). For both asthma diagnosis and severity prediction, the C5.0 modelling with hyperparameter optimization was conducted on: clinical and SNP data (Clinical+TGF), only clinical (OnlyClinical) and minimum redundancy feature selection set (MRMR). Area under ROC (AUCROC) curves were compared using DeLong's test. Results: Minor allele carriers (MACs) in SNP rs2009112 [OR=1.85 (95%CI:1.11-3.1), p=0.016], rs2796821 [OR=1.72 (95%CI:1.1-2.69), p=0.017] and rs2796822 [OR=1.71 (95%CI:1.07-2.71), p=0.022] demonstrated an increased odds of severe asthma. Clinical+TGF model presented better diagnostic potential than OnlyClinical model in both training (p=0.0009) and validation (AUCROC=0.87 vs. 0.80,p=0.0052). At the same time, the MRMR model was not worse than the Clinical+TGF model (p=0.3607 on the training set, p=0.1590 on the validation set), while it was better in comparison with the Only Clinical model (p=0.0010 on the training set, p=0.0235 on validation set, AUCROC=0.85 vs. 0.87). On validation set Clinical+TGF model allowed for asthma diagnosis prediction with 88.4% sensitivity and 73.8% specificity. Discussion: Derived predictive models suggest the analysis of selected SNPs in TGF-beta genes in combination with clinical factors could predict asthma diagnosis with high sensitivity and specificity, however, the benefit of SNP analysis in severity prediction was not shown.
MoreTranslated text
Key words
TGF - transforming growth factor, asthma, inflammation, prediction, development
AI Read Science
Must-Reading Tree
Example
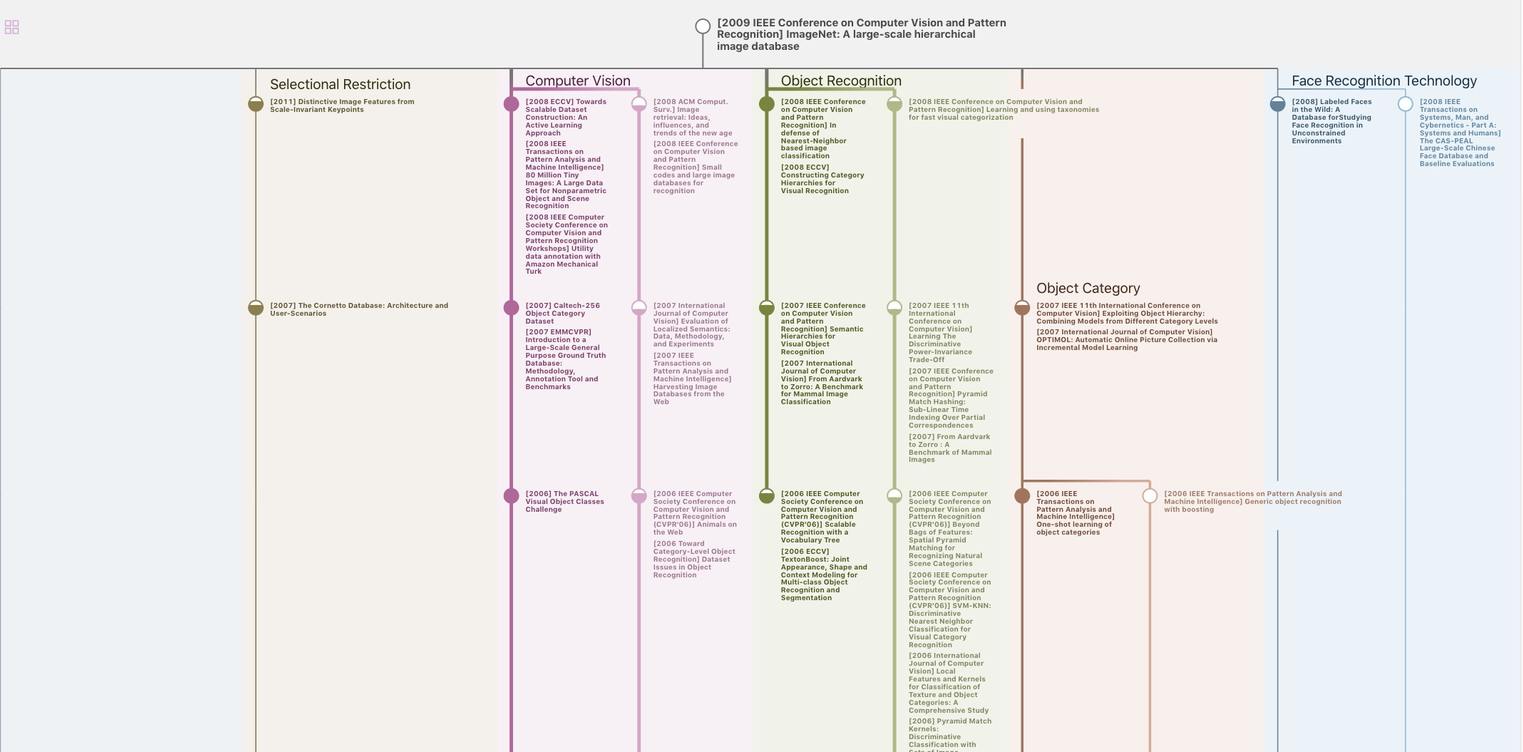
Generate MRT to find the research sequence of this paper
Chat Paper
Summary is being generated by the instructions you defined