Early diagnosis of amyotrophic lateral sclerosis based on fasciculations in muscle ultrasonography: A machine learning approach.
Clinical neurophysiology : official journal of the International Federation of Clinical Neurophysiology(2022)
Abstract
OBJECTIVE:Although fasciculation on muscle ultrasonography (MUS) is useful in diagnosing amyotrophic lateral sclerosis (ALS), its applicability to early diagnosis remains unclear. We aimed to develop and validate diagnostic models especially beneficial to early-stage ALS via machine learning.
METHODS:We investigated 100 patients with ALS, including 50 with early-stage ALS within 9 months from onset, and 100 without ALS. Fifteen muscles were bilaterally observed for 10 s each and the presence of fasciculations was recorded. Hierarchical clustering and nominal logistic regression, neural network, or ensemble learning were applied to the training cohort comprising the early-stage ALS to develop MUS-based diagnostic models, and they were tested in the validation cohort comprising the later-stage ALS.
RESULTS:Fasciculations on MUS in the brainstem or thoracic region had high specificity but limited sensitivities and predictive profiles for diagnosis of ALS. A machine learning-based model comprising eight muscles in the four body regions had a high sensitivity (recall), specificity, and positive predictive value (precision) for both early- and later-stage ALS patients.
CONCLUSIONS:We developed and validated MUS-fasciculation-based diagnostic models for early- and later-stage ALS.
SIGNIFICANCE:Fasciculation detected in relevant muscles on MUS can contribute to the diagnosis of ALS from the early stage.
MoreTranslated text
AI Read Science
Must-Reading Tree
Example
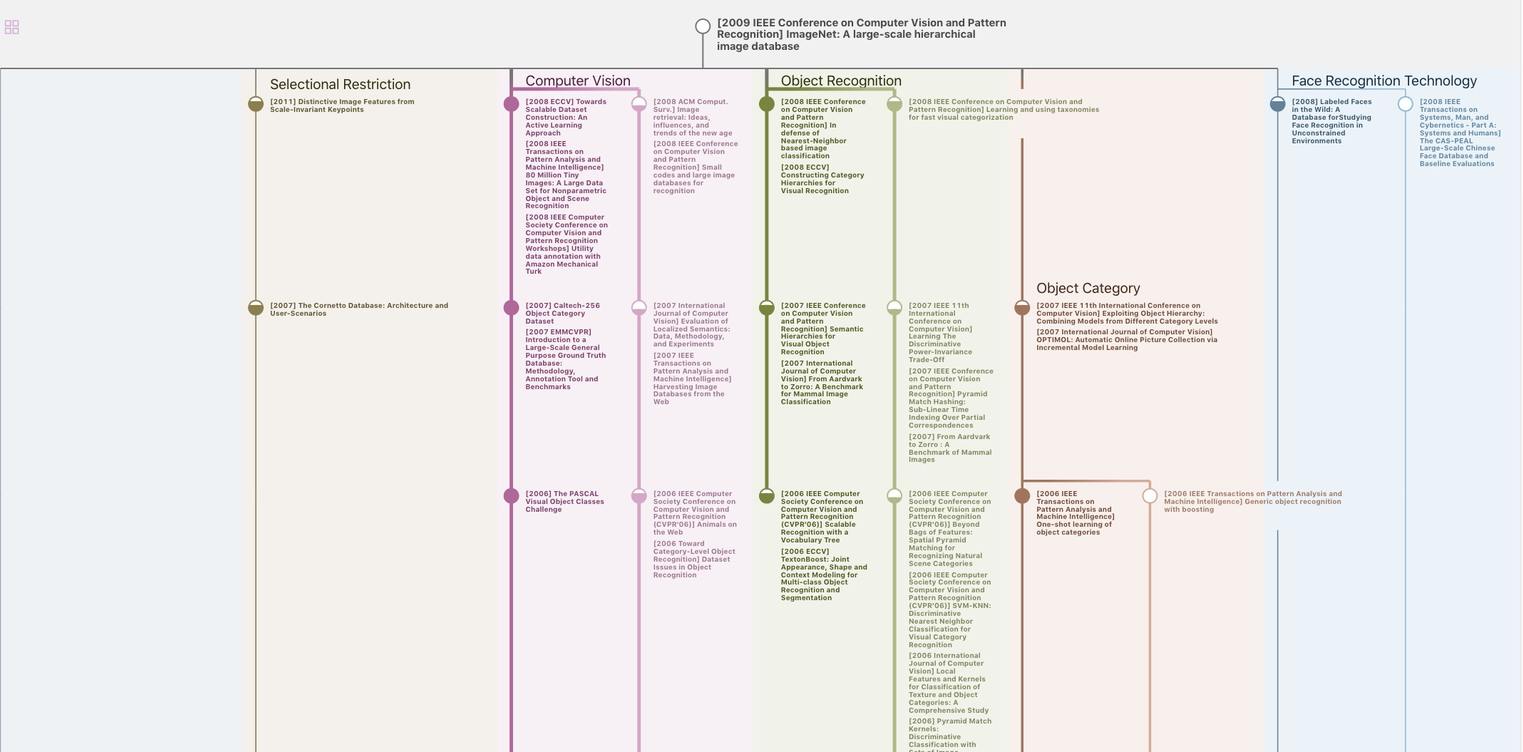
Generate MRT to find the research sequence of this paper
Chat Paper
Summary is being generated by the instructions you defined