Lessons from infant learning for unsupervised machine learning
NATURE MACHINE INTELLIGENCE(2022)
摘要
The desire to reduce the dependence on curated, labeled datasets and to leverage the vast quantities of unlabeled data has triggered renewed interest in unsupervised (or self-supervised) learning algorithms. Despite improved performance due to approaches such as the identification of disentangled latent representations, contrastive learning and clustering optimizations, unsupervised machine learning still falls short of its hypothesized potential as a breakthrough paradigm enabling generally intelligent systems. Inspiration from cognitive (neuro)science has been based mostly on adult learners with access to labels and a vast amount of prior knowledge. To push unsupervised machine learning forward, we argue that developmental science of infant cognition might hold the key to unlocking the next generation of unsupervised learning approaches. We identify three crucial factors enabling infants’ quality and speed of learning: (1) babies’ information processing is guided and constrained; (2) babies are learning from diverse, multimodal inputs; and (3) babies’ input is shaped by development and active learning. We assess the extent to which these insights from infant learning have already been exploited in machine learning, examine how closely these implementations resemble the core insights, and propose how further adoption of these factors can give rise to previously unseen performance levels in unsupervised learning.
更多查看译文
关键词
Cognitive neuroscience,Computer science,Psychology,Engineering,general
AI 理解论文
溯源树
样例
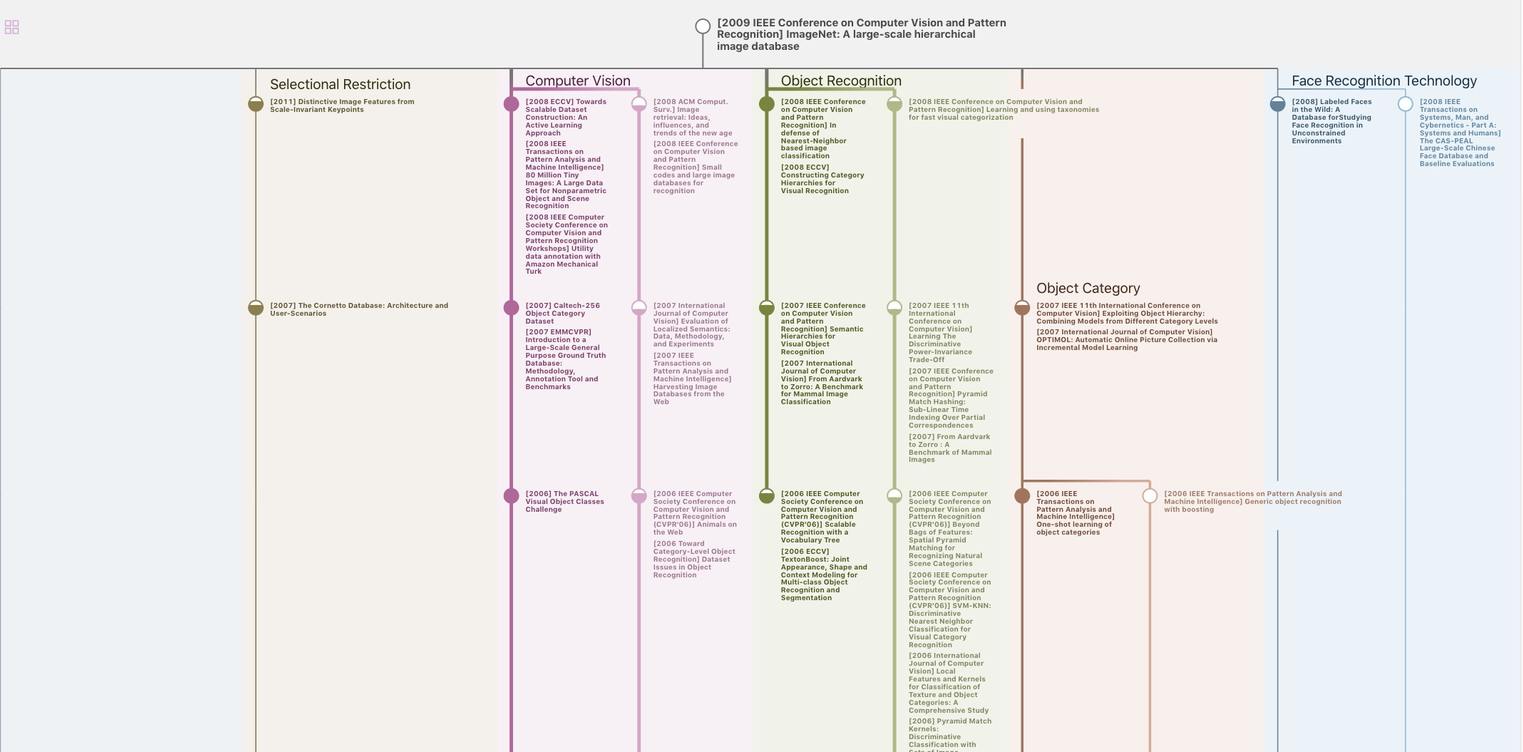
生成溯源树,研究论文发展脉络
Chat Paper
正在生成论文摘要